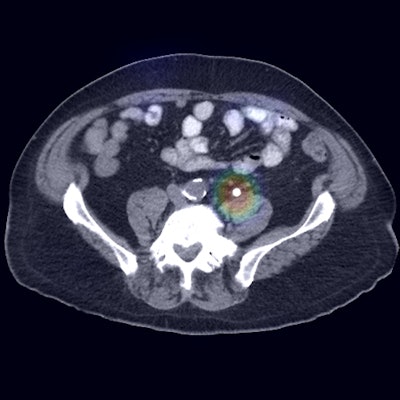
An artificial intelligence (AI) algorithm can accurately detect and classify urinary stones based solely on images from noncontrast single-energy CT scans, according to research presented at last week's Society for Imaging Informatics in Medicine's Conference on Machine Intelligence in Medical Imaging (C-MIMI) in Baltimore.
In a proof-of-concept study, an algorithm developed by a team from Massachusetts General Hospital (MGH) yielded more than 90% sensitivity and specificity for detecting urinary stones on single-energy CT scans. It was also highly accurate for classifying the stones by type -- a task that typically requires dual-energy CT scans.
As a result, the algorithm shows potential as an efficient triage tool in the emergency setting for patients suspected of having urinary stones, according to presenter and doctoral student Hyunkwang Lee.
"It can provide faster prioritization of positive cases for radiologist review, and rapid and accurate diagnosis," he said.
One-stop shopping?
Urinary stone disease, also known as urolithiasis, is a frequent complaint in the ambulatory and emergency setting. It's estimated to account for 731 visits per 100,000 people in the U.S., according to Lee.
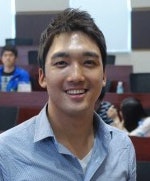
Currently, noncontrast CT is the reference imaging standard for the initial evaluation of a urinary tract stone. A dual-energy CT technique has also been developed to analyze the composition of stones, helping to facilitate targeted treatment for certain types of renal stones, Lee said.
"So radiologists normally detect a urinary stone based on the single-energy CT and classify the stone composition based on the dual-energy CT scans," he said.
The MGH researchers sought to determine if AI could both detect and classify urinary stones based on just the noncontrast, single-energy CT scans. They first gathered a dataset of 289 cases, including 161 patients without a urinary stone and 128 with a stone. Of the 128 patients with a urinary stone, 36 had a stone smaller than 4 mm, while 66 had a stone 4 mm to 10 mm in size. In addition, 26 patients had a stone larger than 10 mm.
Of the stones larger than 4 mm, 79 were classified as nonurate (72) or urate (7). All 120-kVp unenhanced CT images were acquired between January and December 2016 on a Discovery CT750 HD scanner (GE Healthcare). Approximately 80% of the dataset was used for training the GoogLeNet deep convolutional neural network, while 20% was subsequently used to evaluate its performance.
The group's cascade AI algorithm was first trained to identify a region of the urinary tract that covers the top of the kidneys to the bottom of the bladder. The algorithm can recognize 23 different body regions, including the kidneys and the bladder, Lee said. Next, the algorithm seeks to determine if a urinary stone is present or absent. In the final stage, the algorithm attempts to classify any detected urinary stone as nonurate or urate.
In a preprocessing step before training of the algorithm, the images were cleaned by removing image artifacts outside of the body trunk, Lee said. To make their algorithm more generalizable, the researchers also employed data augmentation to synthetically increase the size of their training dataset. This included random vertical flipping of images, as well as random rotation of the images from -10° to 10°, Lee said.
A sensitive and specific method
For the first stage of the process -- body region recognition -- the algorithm produced a mean 97.6% intersection of union for the 23 different body regions. The algorithm also produced a mean intersection of union of the urinary tract (top of kidneys to the bottom of the bladder) of approximately 99%, Lee said.
In testing, the algorithm was also highly sensitive and specific for detecting urinary stones:
- Sensitivity: 90.7%
- Specificity: 91.6%
- Accuracy: 91.1%
- Area under the receiver operating characteristic curve (AUC): 0.964
Delving further into the data, the researchers found that the algorithm's sensitivity was higher for larger stones.
Algorithm detection sensitivity by size of nodule | |||
Stones smaller than 4 mm | Stones 4-10 mm | Stones larger than 10 mm | |
Algorithm sensitivity | 14/17 (82.4%) | 51/57 (89.5%) | 32/33 (97%) |
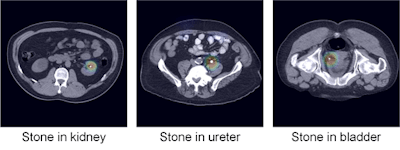
An accurate classifier
In addition, the algorithm was highly accurate for classifying urinary stones:
- Sensitivity: 71.4%
- Specificity: 97.3%
- Accuracy: 95.1%
- AUC: 0.961
Confusion matrix for algorithm in classifying urinary stones | ||
Algorithm prediction: nonurate stone | Algorithm prediction: urate stone | |
Ground truth: nonurate stone | 72 | 2 |
Ground truth: urate stone | 2 | 5 |
"Stones in the kidney, ureter, and bladder can be detected and classified [by the algorithm, and presented with] attention maps [on the image] to highlight the most significant regions for each prediction," Lee said.
Limitations
Lee noted that the algorithm can be confused by very small stones, and it sometimes predicts stones outside of the region of interest. He also acknowledged a number of limitations of the group's research, including its reliance on a limited dataset; there were a relatively small number of cases and the images were produced only by a CT scanner from one vendor. In addition, image analysis was performed on an image slice basis, rather than a patient-based analysis, Lee said.
"In the future, we will include more [image] data from multiple vendors and reform the [algorithm's] output to patient-based predictions, like the number of stones, the largest size of stone, and stone composition per patient," he said.
The proof-of-concept study does show, however, that AI can be feasibly used to detect and classify urinary stones based only on noncontrast, single-energy CT scans, Lee said. The algorithm takes approximately five seconds per patient to complete, and it shows potential for accelerating the triage of patients with urinary stones in the emergency setting, he added.