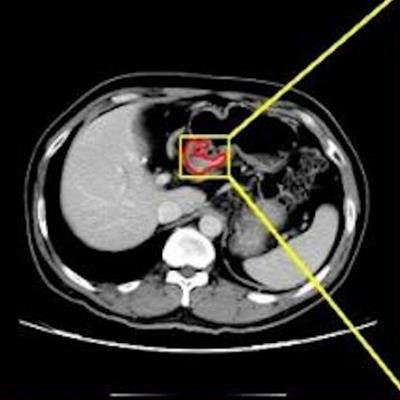
An artificial intelligence (AI) algorithm can predict the presence of occult peritoneal metastasis in gastric cancer patients based on preoperative CT scans, potentially helping in treatment decision-making and avoiding unnecessary surgeries, according to research published online January 5 in JAMA Network Open.
A team of researchers from Stanford University and several institutions in China found that their deep-learning model, called the Peritoneal Metastasis Network (PMetNet), yielded strong diagnostic performance in two different external validation cohorts of patients.
"These findings represent an important step forward in the accurate prediction of occult peritoneal metastasis by applying advanced deep learning techniques," wrote the authors, led by Dr. Yuming Jiang, PhD, of Stanford. "If validated in prospective studies, the PMetNet model could serve as a useful approach for the noninvasive prediction of occult peritoneal metastasis and may inform individualized surgical management of [gastric cancer]."
As patients with peritoneal metastasis have a poor prognosis and are typically not eligible for curative surgery, it's important to be able to preoperatively detect and diagnose these metastases to facilitate decision making and avoiding unnecessary surgeries, according to the researchers. But classic signs of pathology on CT only have a sensitivity of 28% to 51%.
As a result, the researchers sought to develop a deep-learning model to address this unmet need.
In a retrospective study, the authors first gathered clinicopathological and image data from patients with advanced gastric cancer seen at three different hospitals in China. A densely connected convolutional neural network was trained using preoperative contrast-enhanced abdominal CT scans from 1,225 consecutive patients who received either open or laparoscopic surgeries for advanced gastric cancer at Sun Yat-sen University Cancer Center in Guangzhou, China, between January 1, 2008, and January 31, 2013.
They then tested PMetNet on two different external validation cohorts from two other hospitals in China, including 504 patients at Nanfang Hospital in Guangzhou, China, and 249 patients from the Third Affiliated Hospital of Southern Medical University in Guangzhou, China, between January 1, 2007, and December 31, 2017.
Performance of AI for predicting occult peritoneal metastasis in gastric cancer patients | ||
External validation cohort 1 | External validation cohort 2 | |
Sensitivity | 75.4% | 87.5% |
Specificity | 92.9% | 98.2% |
Area under the curve | 0.946 | 0.920 |
In comparison, conventional clinicopathological variables produced area under the curve values ranging from 0.51 to 0.63.
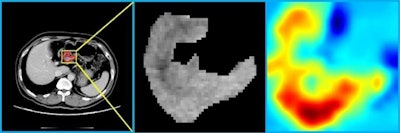
The researchers acknowledged a number of limitations to their study, including its focus on patients in Asian populations. As a result, their algorithm needs to be further evaluated in other ethnic groups, according to the authors.
They also noted that the model can't be used on its own to exclude patients who might be eligible for curative surgery.
"For clinical applications, it will be crucial to integrate information from investigations of other modalities, such as endoscopic ultrasonography and/or laparoscopy, to improve the specificity and sensitivity for the diagnosis of occult peritoneal metastasis," the authors wrote.