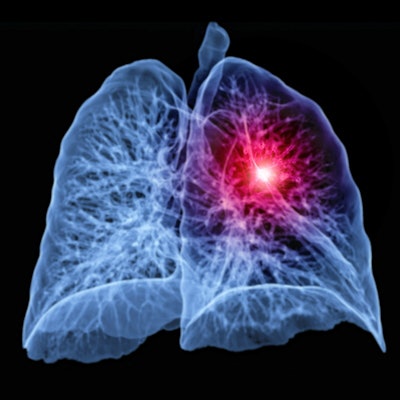
Combining artificial intelligence (AI) with Lung-RADS can sharply increase specificity in low-dose CT (LDCT) lung cancer screening programs without hurting sensitivity, according to a January 19 study in the Journal of the American College of Radiology.
Researchers from the University of Saskatchewan led by Dr. Scott Adams devised a lung nodule management strategy that utilizes a risk score produced by an AI algorithm to potentially upgrade or downgrade an initial Lung-RADS categorization by a radiologist. They estimated their approach would yield over 50% higher specificity and save a minimum of $72 per patient receiving CT lung cancer screening.
"Using an AI risk score combined with Lung-RADS at baseline lung cancer screening may result in fewer follow-up investigations and substantial cost savings," the authors wrote.
The researchers compared the performance of the strategy involving a risk score produced by an AI model developed in 2019 by Google with that of six radiologists on a dataset of 3,197 baseline LDCT studies weighted to be representative of a baseline lung-cancer screening population. The researchers used an AI risk-score threshold (0.27 on a scale of 0 to 1) that yielded the same average 91% sensitivity produced by the six radiologists.
Performance of AI + Lung-RADS for CT lung cancer screening | ||
Average of six radiologists | AI risk score + Lung-RADS | |
Sensitivity | 91% | 91% |
Specificity | 61% | 96% |
What's more, their nodule management strategy upgraded 41 (0.2%) of initial Lung-RADS category 1 or 2 classifications to category 3, while downgrading 5,750 (30%) initial Lung-RADS category 3 or higher classifications to category 2.
After calculating costs based on the 2019 Medicare Physician Fee Schedule nonfacility National Payment Amount, the researchers estimated that management changes resulting from the use of this "AI-informed" strategy would yield cost savings up to $72 per patient screened.
"Substantial cost savings were demonstrated due to the increased specificity afforded by the AI algorithm and reduced need for interval investigations after baseline lung cancer screening," the authors wrote.
The researchers noted that future work on this approach could consider developing additional actionable AI thresholds to refine management recommendations, particularly for Lung-RADS category 4 lung nodules. It may also be helpful for AI output to directly associate to Lung-RADS categories or similar risk categorization systems, they said.
"Using an operating point that results in sufficiently higher sensitivity than that of radiologists may allow AI algorithms to serve as a tool to identify normal studies that may not require review by a radiologist, as has been suggested for screening mammography," they wrote. "Systematic manual review of cases deemed high or low risk by the AI algorithm should be another area of further investigation to identify new radiologic signs that may potentially improve radiologists' accuracy even in the absence of AI. "