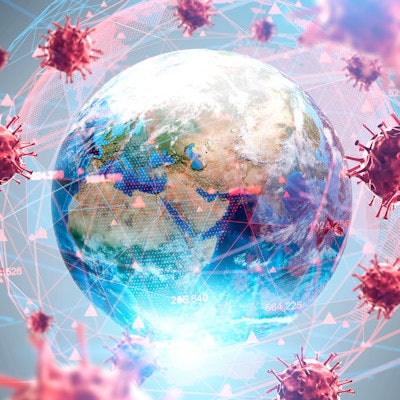
Deep-learning models that have been trained on datasets from multiple institutions around the world yield promising and generalizable results for identifying COVID-19 pneumonia on chest CT exams, as well as in predicting adverse patient outcomes.
In an article published online February 5 in the European Journal of Radiology, a multinational team of researchers led by Kuang Gong, PhD, of Massachusetts General Hospital shared how combining analysis of CT-derived biomarkers with information from electronic health records (EHR) can offer a high level of prognostic performance in patients with COVID-19.
The researchers trained and tested a deep-learning network to segment regions of COVID-19 lung infection using 369 noncontrast chest CT exams acquired at five institutions around the world on CT scanners from GE Healthcare, Philips Healthcare, and Siemens Healthineers.
Two image biomarkers -- total opacity ratio and consolidation ratio -- were then extracted and used together with analysis of vital signs and laboratory test results from the EHR in three of the institutional datasets to identify the factors that are predictive of severe patient outcomes, according to the researchers.
"Results indicated that age, [oxygen saturation], CT-derived biomarkers, [platelet count], and [white blood cell count] were the most important prognostic predictors of COVID-19 pneumonia in our prognosis models," the authors wrote.
Performance of final deep-learning algorithms for predicting COVID-19 prognosis | |||
Institute 1 dataset | Institute 2 dataset | Institute 3 dataset | |
Correlation coefficient with manual segmentation | 0.755 | 0.919 | 0.824 |
Area under the curve for final prognosis prediction | 0.85 | 0.93 | 0.86 |
Although the researchers created specific models for each individual cohort due to different treatment policies and variations in measurement time among the institutions, they said they intend to pursue a united prognosis model in the future. They also plan to conduct prognosis analysis using chest x-rays and EHR data.
Deep Covid DeteCT
Meanwhile, another multinational research group led by Edward Lee, PhD, of Stanford University in California has developed Deep COVID DeteCT (DCD), a deep-learning model that can detect COVID-19 on chest CT scans without requiring special image processing. The convolutional neural network can also enable prognostic predictions by tracking image features on the algorithm over time on sequential CT studies, according to the authors, who detailed their experience in a study published online January 29 in NPJ Digital Medicine.
Developed and tested using image data that included 3,529 COVID-19-positive patients from 13 institutions in eight countries, DCD analyzes the entire chest CT volume to distinguish between COVID-19 pneumonia, non-COVID-19 pneumonia, and normal controls. The imaging datasets were acquired from a variety of vendors -- Canon Medical Systems, NeuSoft Medical Systems, Philips, and Siemens -- and had heterogeneous protocols, including both contrast and non-contrast chest CT exams, according to the researchers.
They found that DCD yielded generalizable performance on patients from two sites in China, as well as from Stanford University; Unity Health Toronto; Rajaie Cardiovascular Medical and Research Center in Tehran, Iran; the Henry Ford Health System in Detroit; the Federal University of São Paulo in São Paulo, Brazil; Koç University School of Medicine, Istanbul, Turkey; Tehran University of Medical Sciences in Iran; and the MosMedData COVID-19 dataset from Russia.
Deep COVID DeteCT produced an area under the curve ranging from 0.808 to 0.916 for identifying COVID-19 pneumonia, 0.741 to 0.895 for non-COVID-19 pneumonia, and 0.806 to 0.98 for normal controls. Accuracy levels ranged from 70.7% to 88.1%.
The researchers also demonstrated how DCD could be utilized to track the course of COVID-19 on sequential CT scans and predict a patient's prognosis.
"We show indicative patterns in DCD features that correlate with the patient outcome," the authors wrote. "Finally, we show heat maps that highlight the visual progression of a patient's disease trajectory over time."