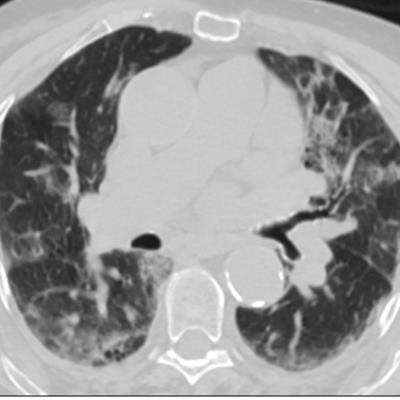
An "unsupervised" artificial intelligence (AI) model -- that is, one trained without image annotations -- can yield accurate predictions of a COVID-19 patient's survival based on their chest CT exams, according to research published online in Medical Image Analysis.
Researchers from Massachusetts General Hospital (MGH) developed their pix2surv algorithm using just a training set consisting of chest CT exams without any image annotations of COVID-19 patients with known survival times. In testing, pix2surv outperformed laboratory tests as well as current visual and quantitative image-based methods for predicting patient survival.
What's more, pix2surv stratified patients into low- and high-risk groups by a wider margin than those produced by other predictive techniques, according to the researchers. The algorithm also generates individualized Kaplan-Meier survival for each patient.
"The use of unsupervised AI as an integral part of the survival prediction model makes it possible to perform prognostic predictions directly from the original CT images of patients at a higher accuracy than what was previously possible in quantitative imaging," said Hiroyuki Yoshida, PhD, in a statement.
Although several methods have been proposed for predicting COVID-19 patient outcomes based on their imaging studies, these techniques have involved subjective assessment, semiautomated schemes, or supervised deep-learning models, according to the researchers.
"Therefore, there is an unmet need for an objective survival analysis system that would automatically extract, select, and combine image-based features for the calculation of complete time-to-event information for optimally predicting the prognosis of patients with COVID-19, without the laborious and expensive annotation effort that is required by supervised learning schemes," the authors wrote.
Building on previous work that resulted in a supervised AI model for predicting COVID-19 patient survival from chest CT images, the researchers elected in this study to use a weakly unsupervised training approach with a generative adversarial network (GAN).
For training, the researchers merely provided pix2surv with chest CT images along with the patient's survival times. This method doesn't require specific image annotations for algorithm training, and it can produce end-to-end survival analysis directly from 2D CT image slices, they said.
"It is a much more precise and highly advanced AI technology," Yoshida said.
The investigators assembled a database of 214 COVID-19 patients from the medical records of MGH and the Brigham and Women's Hospital. Although all 214 patients were considered for mortality analysis, only 141 patients were assessed for progression as 73 patients received their CT exam after already being admitted to the intensive care unit.
Using a bootstrap-based evaluation involving over 100 bootstrap replicates, the researchers compared the performance of pix2surv and four other predictive methods: lab tests, visual assessment of CT for a total severity score, visual assessment of CT for crazy paving and consolidation (CPC), and semiautomated assessment of the percentage of well-aerated lung parenchyma.
Performance of unsupervised AI model for predicting COVID-19 disease progression and mortality | |||||
Semiautomated assessment of percentage of well-aerated lung parenchyma | Visual assessment of CT total severity score | Visual assessment of CT for crazy paving and consolidations | Lab tests | AI model (pix2surv) | |
Prediction of progression (C-index) | 60.7% | 62.6% | 62.1% | 69.8% | 82.5% |
Prediction of mortality (C-index) | 53.4% | 54.6% | 56.8% | 62.5% | 80.8% |
The model's prognostic performance for estimating the disease progression and mortality of patients with COVID-19 was significantly better (p < 0.0001) than those based on established laboratory tests or existing image-based visual and quantitative predictors, according to the researchers.
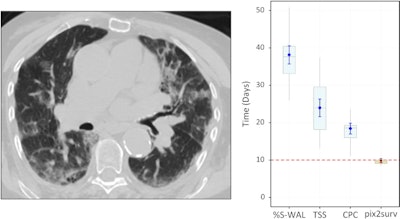
The authors believe that their model could also be utilized for other diseases.
"Issues such as Long COVID or generalization of the model to other
diseases manifested in medical images provide topics for future studies," Yoshida said.