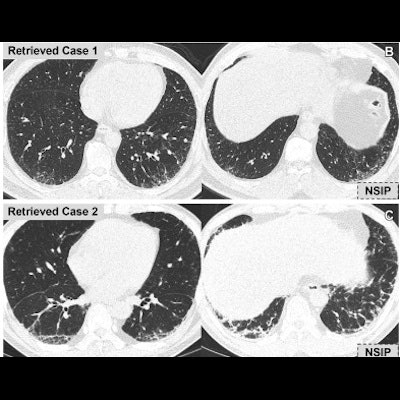
Using a content-based image retrieval (CBIR) search engine with a deep-learning algorithm boosts reader performance for diagnosing interstitial lung disease (ILD) on CT exams, according to a study published October 12 in Radiology.
"This system can be expected to provide radiologic decision support to centers where thoracic imaging expertise is unavailable or cases of ILD are scarce," wrote a team led by Dr. Jooae Choe, PhD, of the University of Ulsan College of Medicine in Seoul.
The term "interstitial lung disease" covers a variety of parenchymal lung disorders that can share features on clinical presentation, making diagnosis tricky, the authors noted. High-resolution CT is used to diagnose these disorders, but readers must have experience and expertise to best interpret findings.
Choe and colleagues investigated whether using a content-based image retrieval (CBIR) search engine -- which classifies and retrieves images that have similar features to the image being interpreted -- with a deep-learning algorithm could make diagnosis more straightforward, even for readers with varying levels of experience.
The study included 288 patients with confirmed interstitial lung disease and available CT exam images between January 2000 and December 2015. The image database the team used had four disease classes:
- Usual interstitial pneumonia (98 cases)
- Nonspecific interstitial pneumonia (101 cases)
- Cryptogenic organizing pneumonia (40 cases)
- Chronic hypersensitivity pneumonitis (49 cases)
The authors chose 80 CT exams out of the 288 to be test exams for the content-based image retrieval search engine; the engine chose the top three similar CT images for these 80 exams from the database by comparing disease patterns that had been quantified by a deep-learning algorithm.
Eight readers with a range of CT interpretation experience read the CT exams in two sessions two weeks apart, before and after applying content-based image retrieval. (The reader group consisted of three radiologists -- two of which were thoracic specialists -- two radiology residents, and two nonradiology physicians.)
The combination of the content-based image retrieval search engine with deep learning improved interpretation metrics across all readers, the group found.
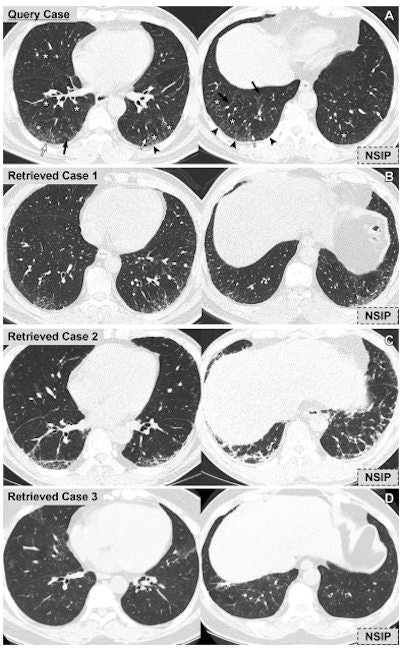
Diagnostic accuracy for interpretation of interstitial lung disease CT exams, before and after use of a content-based image retrieval (CBIR) search engine | ||
Performance measure | Before CBIR | After CBIR |
Overall diagnostic accuracy | 46.1% | 60.9% |
Diagnostic accuracy for usual interstitial pneumonia | 52.4% | 72.8% |
Diagnostic accuracy for idiopathic nonspecific interstitial pneumonia | 42.9% | 61.6% |
Interreader agreement also improved, from a kappa agreement of 0.32 before using CBIR to 0.47 after using it.
The study suggests a new way for radiologists to learn patterns on CT exams that improve their diagnostic accuracy, wrote Dr. Mark Wielpütz of the University of Heidelberg in Germany in an accompanying editorial.
"Until now, radiologists have referred to textbooks and online databases to find patterns that may help them in making a correct diagnosis," he noted. "Those methods require text-based searches for a pattern that may be only vaguely understood by the radiologist. A pattern-based and automated search should help radiologists and may be a game changer for computer-aided diagnosis."