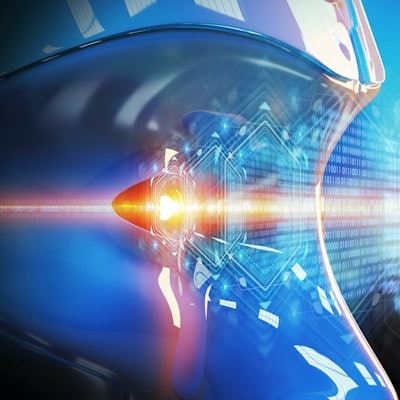
An artificial intelligence (AI) algorithm can help to avoid missed fractures on whole-body trauma CT exams performed on emergency department patients, according to research published October 3 in Scientific Reports.
After training a convolutional neural network (CNN), a team of researchers led by Takaki Inoue and Satoshi Maki of Chiba University in Japan found that it significantly increased the sensitivity of orthopedic surgeons in detecting pelvic, rib, and spine fractures.
"Application of the CNN model may lead to reductions in missed fractures from whole-body CT images and to faster workflows and improved patient care through efficient diagnosis in polytrauma patients," the authors wrote.
Fractures can be missed in the emergency department, resulting in delayed treatment and a poor prognosis. In their study, the researchers sought to explore the potential for AI to help avoid missed fractures of the pelvis, rib, or spine.
They trained and tested a CNN using 7,664 whole-body axial CT slices of the chest, abdomen, and pelvis from 200 patients. Of the 7,664 CT images, 5,217 images from 181 patients were used for training and validation, while 2,447 images from 19 patients were set aside for a test dataset. The test dataset included 5.8% with pelvic fractures, 5.5% with spine fractures, and 3.6% with rib fractures.
On its own, the algorithm produced a sensitivity of 78.6%, precision of 64.8%, and an F1 score of 0.711. The researchers then assessed the performance of three orthopedic surgeons on the test set, both without and then with the help of AI. Orthopedic surgeons 1 and 2 had three years of experience, while orthopedic surgeon 3 had eight years of experience.
Impact of AI on detection of fractures on whole-body CT | |||
Without AI assistance | With AI assistance | p-value | |
Orthopedic surgeon 1 | 69.7% | 82.9% | < 0.0001 |
Orthopedic surgeon 2 | 67.6% | 81.8% | < 0.0001 |
Orthopedic surgeon 3 | 76.4% | 82.6% | 0.0003 |
AI also sharply decreased the time to diagnosis, dropping from 278.4 seconds to 162.3 seconds for surgeon 1, from 205.2 seconds to 144.5 seconds for surgeon 2, and from 233.7 seconds to 155.5 seconds for surgeon 3. All differences were statistically significant (p < 0.0001).
"With CNN model assistance, surgeons showed improved sensitivity without loss of precision in detecting fractures and the time of reading and interpreting CT scans was reduced, especially for less experienced orthopedic surgeons," the authors wrote. "Moreover, the precision reduction was not observed with CNN model assistance."
The authors noted that the CNN could serve as a triage system in a busy emergency department.
"If the CNN can detect a fracture before orthopedic surgeons or radiologists have a chance to review the images, the suspected fracture image can become a high priority in the worklist," the authors wrote. "If the physician can prioritize interpretation of images with potentially positive findings, delays in diagnosis can be minimized, thereby improving patient care."
Furthermore, use of AI can also lead to shorter reading times, according to the authors.
"Even though each examination takes just a few minutes, a reduction in reading time has a significant impact for emergency staff who make multiple clinical decisions each day," they wrote. "Emergency physicians and radiologists on long shifts may experience fatigue and oculomotor strain, resulting in a reduced ability to focus and to detect fractures. Fracture recognition using CNN is not only able to detect subtle findings that are difficult for inexperienced physicians to diagnose but also prevents cognitive errors due to human fatigue and biased image interpretation."