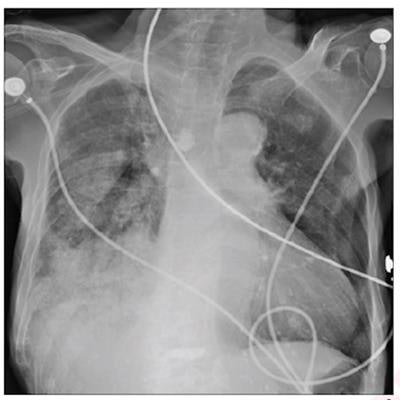
A deep-learning AI model based on chest x-rays can predict 30-day mortality in patients with community-acquired pneumonia (CAP), according to a recent study in the American Journal of Roentgenology.
A team led by Eui Jin Hwang, MD, PhD, of Seoul National University College of Medicine in Korea tested a deep-learning model using x-rays of community-acquired pneumonia, with the AI model outperforming the CURB-65 score, an established tool used in daily practice to assess CAP. The model could provide valuable information at the time patients are diagnosed, the authors suggested.
"The deep-learning model may guide clinical decision-making in the management of patients with CAP by identifying high-risk patients who warrant hospitalization and intensive treatment," the group wrote.
Pneumonia was the number one cause of death in 2019 among infectious diseases and the second most common cause of death in 2020 after COVID-19, according to the Centers for Disease Control and Prevention. Chest x-ray is an essential tool for diagnosing CAP, yet risk predictions for patients based on initial imaging remains a challenge, the researchers noted.
In this study, Hwang and colleagues developed a model to predict 30-day mortality using chest x-rays from 7,105 patients from their hospital. They tested the model in two separate datasets (n = 467 and n = 381) from two different hospitals. The two groups had an overall 30-day mortality rate of 11%.
The model's performance for predicting mortality was calculated using areas under the curve (AUC) compared with CURB-65 scores, which are severity scores comprising five clinical variables.
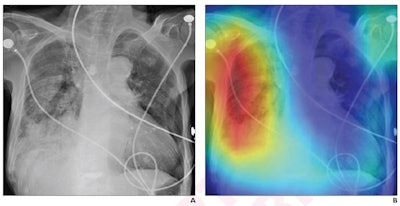
The deep-learning model predicted 30-day all-cause mortality in patients with CAP with AUCs ranging from 0.77 to 0.8 in the two test cohorts. Additionally, the model showed a statistically significant higher specificity (range of 61% to 69%) than the CURB-65 score (44% to 58%).
"An advantage of using chest radiographs as an input to the DL model compared to use of clinical variables is that chest radiographs may allow automated processing that is not influenced by subjective evaluation by physicians," the authors explained.
Yet they also wrote that an important shortcoming of the DL model is the difficulty in explaining the logical background of its predictions. While class activation maps display areas of pneumonia in patients with high predicted risk, they do not provide this logical explanation, they wrote.
"Since this lack of interpretability may hinder the DL model's clinical adoption, future research should seek to better understand the basis of the [its] predictions," the researchers concluded.
The study was published June 14 in the American Journal of Roentenology.