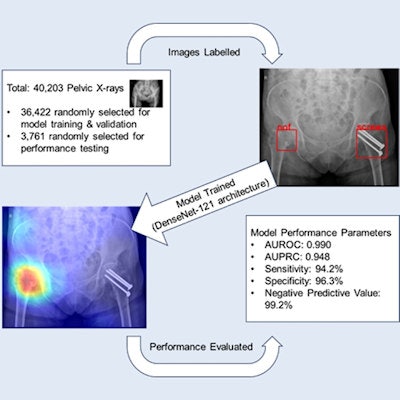
Researchers in Singapore have developed an AI algorithm that accurately detects hip fractures on pelvic x-rays -- even with the presence of metallic implants, according to a July 11 study published in iScience.
A group led by Yet Yen Yan, MD, of Changi General Hospital and colleagues at the Duke-NUS Medical School, developed a deep-learning model using more than 40,000 pelvic x-rays with and without fractures. The algorithm demonstrated high accuracy when it was tested on a set of emergency department (ED) images, the authors reported.
"The high sensitivity and negative predictive value of our model underscores the potential for [AI] solutions like ours to be particularly useful in urgent or emergency care settings, where emphasis is on avoiding missed diagnoses," the researchers wrote.
The global incidence of hip fractures is increasing due to an aging population and is estimated to reach 6.3 million by 2050, according to the authors. These fractures commonly occur in older adults, with misinterpretations of pelvic radiographs contributing to missed diagnoses and delayed surgical repair, they wrote.
Thus, hip fractures represent a promising target for AI algorithms, the authors wrote. To that end, they gathered 40,203 pelvic x-rays from their hospital's PACS. Of these, 18,803 were performed in the ED, and 21,400 were performed in an ambulatory or inpatient setting.
The images were used first to train and validate a DenseNet-121 convolutional neural network (CNN), which was selected based on its comparatively fewer parameters and faster training time, the authors noted. The presence of a hip fracture was defined as any fracture involving the proximal femora, with ground-truth labels (hip fracture present or absent) determined by two expert radiologists.
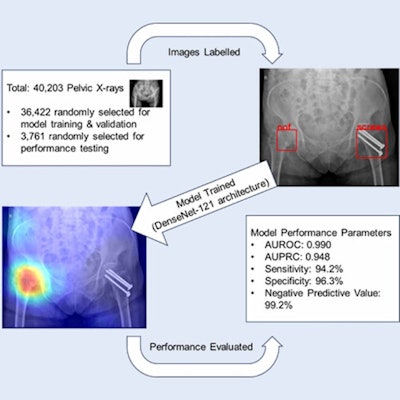
Of the 18,803 pelvic x-rays performed in the ED, 3,761 (20%) were randomly selected to form a holdout test set for subsequent evaluation of the model. This was done "to better approximate the potential real-world use scenario, " where patients with suspected hip fractures present almost exclusively to EDs, the authors wrote.
The holdout set contained 463 images (12.3%) that were positive for hip fracture. Orthopedic implants in either the proximal femur or the bony pelvis were also present in a significant proportion of the images, the researchers noted.
For identifying images correctly, the CNN achieved an area under the curve of the receiver operating characteristic curve (AUROC) of 0.990 and an area under the precision-recall (PR) curve (AUPRC) of 0.948, according to the results. Also, the model predicted 27 false-negatives, 121 false-positives, and it detected seven of seven nondisplaced fractures (sensitivity 100%) and 429 of 456 displaced fractures (sensitivity 94.1%).
In addition, a subgroup analysis of the model's performance was performed based on images with orthopedic implants in the holdout test set. There were 389 x-rays with at least one implant, of which 32 (8.2%) were positive for hip fracture.
Model performance in the presence of orthopedic implants | ||
Implant present | Implant absent | |
AUROC | 0.969 | 0.991 |
AUPRC | 0.914 | 0.950 |
Accuracy | 95% | 96% |
Sensitivity | 87% | 95% |
Specificity | 96% | 96% |
Positive predictive value | 66.7% | 77.9% |
Negative predictive value | 98.8% | 99.2% |
"This study demonstrates the ability for a [deep-learning] CNN solution to identify hip fractures on pelvic radiographs," the group wrote.
A major strength of the study was its inclusion of images regardless of perceived technical and diagnostic difficulty, the existence of metallic implants, or the presence of other radiographically identified pathologies, according to the authors. This investigation contrasts with most previous studies, which have excluded these subsets, they wrote.
The authors also noted that they were able to develop their own CNN model using the publicly available DenseNet-121 architecture, along with pelvic radiographs and radiology reports obtained from a single tertiary institution. Moreover, the entire study was carried out over 12 months, they added.
"The favorable performance achieved by this model demonstrates that it may be feasible for institutions to develop their own deep learning algorithms for computer aided diagnoses, based on patterns of local prevalence and local imaging parameters," the group concluded.
The full study is available to read in iScience.