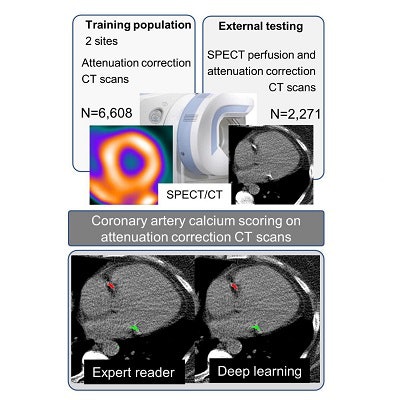
A deep-learning (DL) model developed for SPECT/CT myocardial perfusion imaging (MPI) could help clinicians identify patients at risk for major adverse cardiac events, according to a study published October 7 in the Journal of Nuclear Medicine.
An international team of researchers developed a DL model that automatically calculates coronary artery calcium (CAC) scores for patients undergoing SPECT/CT MPI scans by using data from the attenuation correction component of the scans. They found the model's predictions were significantly associated with an individual's risk of adverse events such as heart attacks.
"DL CAC scores can be quantified automatically following SPECT/CT MPI, without impeding clinical workflow, in order to improve classification of a significant proportion of patients," wrote corresponding author Piotr Slomka, PhD, of Cedars-Sinai Medical Center in Los Angeles, and colleagues.
SPECT myocardial perfusion imaging is widely used to diagnose coronary artery disease by measuring blood flow deficits in myocardial tissue. SPECT/CT adds a CT component for attenuation correction, a step applied during image reconstruction to remove distortions and improve diagnostic accuracy.
Research suggests these attenuation correction images can be used to measure amounts of CAC, which is a well-established marker of atherosclerosis. While it is possible to quantify CAC manually from SPECT/CT scans, the method can be time consuming as it requires scrolling between CT image slices, and it is not commonly performed.
Moreover, it is also possible to visually estimate CAC on these scans, but visual estimation is inherently subjective and requires experience to be performed accurately, according to the authors.
In this study, the researchers developed a DL model that mimics the clinical approach of scrolling between slices to quantify CAC and explored associations between CAC scores it predicted and major adverse cardiovascular events (MACE), such as death or myocardial infarction.
The model was trained using imaging data on 6,608 patients who underwent SPECT/CT MPI with CT attenuation correction at one of two centers (Yale University in New Haven, CT and Cardiovascular Imaging Technologies in Kansas City, MO), while 2,271 patients without known coronary artery disease who underwent SPECT/CT MPI at a third center (the University of Calgary in Alberta, Canada) were used as a testing cohort.
First, the researchers determined how well the DL model identified CAC on SPECT/CT images compared with experts who annotated the images. Based on the DL model results, CAC was 0 in 908 patients, between 1-100 in 596 patients, between 100-400 in 354 patients, and > 400 in 413 patients.
Concordance between the DL model and the expert reads in these CAC categories in the external testing population was excellent, with a linearly weighted kappa score (level of agreement) of 0.80, according to the findings.
Next, the researchers analyzed associations between the DL model's CAC predictions and MACE. During a median follow-up period of 2.8 years, 320 patients experienced at least one event. Compared to patients with a CAC score of 0, patients with CAC scores between 1-100 (hazard ratio [HR] of 2.20), 101-400 (HR 4.58), and CAC > 400 (HR 5.92) were at significantly increased risk. The risk was similar across categories based on expert annotated CAC categories.
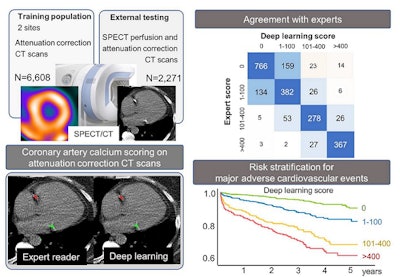
In addition, the DL model SPECT/CT attenuation correction results were obtained fully automatically in less than 2 seconds per scan, compared to approximately 5 minutes for expert annotations, the group added.
"DL CAC scores from CTAC could be utilized clinically to significantly improve risk stratification in patients undergoing SPECT/CT MPI without the need for physician or technician time for manual annotation," the authors wrote.
The authors noted limitations of the study, namely that the model was trained to differentiate coronary from noncoronary calcifications using expert annotations. Some lesions are challenging for expert readers to annotate, and the DL model would also be expected to have difficulties with these areas. Thus, while the DL method provides fully automated results, they would still need to be verified by a physician, they wrote.
Additional research is warranted, according to the authors.
"Our work adds to a growing body of literature supporting integration of CAC scores when interpreting MPI," Slomka and colleagues concluded.