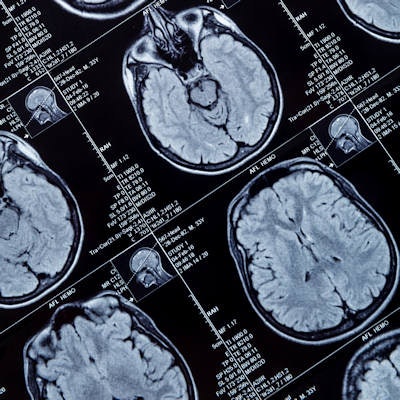
Autism spectrum disorder (ASD) is a complicated condition that can be difficult to diagnose, especially since its evaluation is currently conducted by assessing behavioral symptoms, according to a study published in the September 1 issue of the Journal of Neuroscience Methods.
That's why identifying a neuroimaging biomarker on brain MRI could improve the diagnosis of autism spectrum disorder -- and incorporating machine learning into the mix could make the imaging even more effective, wrote a team led by Ming Xu, PhD, of the Chinese Academy of Science in Beijing.
"[MRI's] noninvasive imaging techniques are promising tools for investigating the neurological underpinnings of autism spectrum disorder, which are essential for developing discriminative neuroimaging biomarkers for clinical diagnosis," the group wrote. "In recent years, various machine learning approaches have been applied in ASD classifications ... [these] approaches can extract more informative features in a data-driven manner, and facilitate exploring more complex abnormal imaging patterns for individual-level diagnosis."
Autism spectrum disorder tends to appear in early childhood, and its cause remains unclear, the group noted. The condition can be quite burdensome for both its sufferers and their families, making precise diagnosis important.
To boost MRI's contribution to the evaluation of autistic children, researchers have begun to explore the use of machine learning with the modality (J Neurosci Methods, 1 September 2021, Volume 361, article 109271). Xu's group reviewed 119 studies from the past 10 years that describe current ASD classification methods using machine learning across a variety of MRI techniques, including functional MRI, T1-weighted, and diffusion-tensor imaging protocols. Machine learning approaches included feature extraction, model training, model testing, and performance evaluation.
The literature review found a range of accuracy for classifying ASD of 60% to 98% across a variety of MRI techniques and machine learning approaches.
The quest for an imaging biomarker to diagnose autism spectrum disorder is yet to be fulfilled, but using machine learning with imaging shows potential for getting there, according to Xu and colleagues.
"Even though this field is still far from clinical use, we believe that with the development of new powerful mathematical tools and closer interdisciplinary cooperation, imaging-based diagnosis of ASD is promising and will eventually be achieved," they concluded.