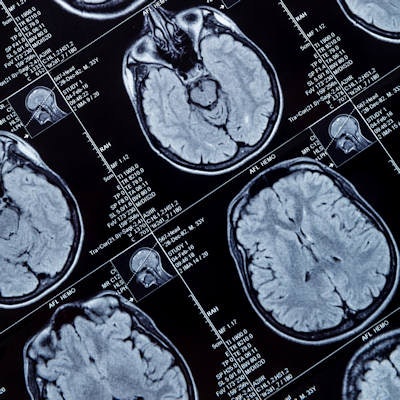
Using a deep-learning model with brain MRI data improves the modality's ability to identify even preclinical Alzheimer's disease, according to research published March 29 in Alzheimer's Research & Therapy.
The results demonstrate that there's a way to make neuroimaging with MRI in individuals with suspected Alzheimer's disease an even more useful prognostic tool, wrote a team led by Xinyang Feng, PhD, of Meta Platforms in Menlo Park, CA. At the time of the study, Feng was at Columbia University in New York City.
"Our study provides the proof-of-principle that imaging-based deep learning models that are examined in concert with a disease's pathophysiology will yield a highly accurate model and improve performance in prognosticating disease," the group noted.
Three core indicators have been identified for Alzheimer's disease -- amyloid pathology, tau pathology, and neurodegeneration -- and all have biomarkers that can be tracked, Feng and colleagues explained. The first two are detected using cerebrospinal fluid (CSF) evaluation or with PET imaging, while neurodegeneration can be assessed with PET but also with MRI-based measurements that show hippocampus formation. One of MRI's advantages over PET is that it does not require contrast.
But diagnosing prodromal Alzheimer's disease in patients with mild cognitive impairment can be tricky, which is why deep learning could make MRI more effective.
"[Our] ability to diagnose prodromal Alzheimer's disease when presented with a ... patient [with mild cognitive impairment] is currently inadequate," the authors wrote. "Showing that a deep learning algorithm can address the questions that relate to prodromal Alzheimer's disease would not only better validate its classification capabilities, but since derived from conventionally acquired MRI scans, would potentially expand its ... utility as a screening tool."
For their study, the investigators created a model that classified Alzheimer's dementia and generated brain maps of neuropathology; tested it with 975 MRI scans of patients with preclinical Alzheimer's disease and 1,943 healthy control exams; and compared its performance to other disease indicators such as amyloid pathology, tau pathology, and neuroimaging biomarkers of brain degeneration gleaned from conventional MRI data.
The researchers found that, overall, the MRI-based deep-learning model had a high accuracy of distinguishing between control exams and those exams that showed Alzheimer's indicators, with an area under the receiver operating curve (AUROC) of 0.973. The maps the model generated focused on the hippocampus, the area of the brain where Alzheimer's disease pathology manifests.
The team also found that the MRI-based deep learning model bested other methods of identifying preclinical Alzheimer's disease.
MRI-based deep learning model's performance for identifying prodromal Alzheimer's disease compared to other methods | |||||
Measure | CSF tau | CSF amyloid biomarkers | CSF tau/amyloid biomarkers | Conventional MRI measures of hippocampal volume | MRI-based deep-learning model |
AUROC | 0.682 | 0.702 | 0.703 | 0.733 | 0.788 |
The study suggests that using deep learning with brain MRI could make it more effective as a diagnostic tool for Alzheimer's disease, according to the researchers.
"Machine learning techniques ... that can extract useful information for the purposes of prodromal Alzheimer's disease detection, from conventional MRIs that have in any case been acquired, have the additional advantages of reducing patient burden and cost incurred by lumbar punctures, injection of radioactive ligands, or another additional testing," they concluded.