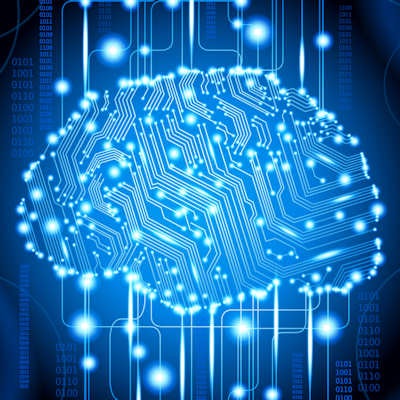
Deep learning can accurately differentiate benign and malignant breast tumors on ultrasound shear-wave elastography (SWE) and outperform methods typically used in computer-aided diagnosis (CADx) software, according to research published in the December issue of Ultrasonics.
After building and testing a deep-learning model for automatically learning image features on SWE and characterizing breast tumors, the team led by Qi Zhang, PhD, of Shanghai University in China found that the technique yielded more than 93% accuracy on the elastogram images of more than 200 patients. It was also more accurate than a semiautomated computer vision technique that analyzed images based on statistical features extracted by expert readers -- an approach that is frequently utilized in CADx software.
"The [deep-learning] architecture could be potentially used in future clinical [CADx] of breast tumors," Zhang and colleagues wrote.
Differentiating breast lesions
While standard ultrasound exams can identify morphological alterations caused by breast cancer, shear-wave elastography can also contribute additional information on the biomechanical and functional properties of breast tumors. As a result, SWE can help differentiate between benign and malignant lesions, according to the researchers (Ultrasonics, December 2016, Vol. 72, pp. 150-157).
CADx software for breast tumors visualized with both conventional ultrasound and SWE traditionally relies on statistical features such as tumor shape and morphological parameters, intensity statistics, and texture features for quantifying tumor heterogeneity. However, it usually takes expert knowledge or human labor to extract these features for the CADx software, they wrote.
Deep learning may be able to improve upon the performance of those methods, but the presence of artifacts, noise, and other irrelevant patterns such as irregular stiffness distributions can make it challenging to develop a deep-learning model that can learn from complex SWE image data.
"Another challenge is how to understand and utilize the patterns that may be task-relevant but are difficult to interpret by human observers, such as the black holes absent of color on SWE, i.e., the missing areas with invalid stiffness values," they wrote.
Because they do not focus on differentiating between image patterns that are useful or not useful, popular deep-learning methods such as the autoencoder and convolutional neural networks were unsuitable for this task, according to the researchers. As a result, they sought to use a deep-learning method based on the point-wise gated Boltzmann machine (PGBM), which incorporates a "gating" mechanism to estimate where these useful patterns occur. In the second phase, artificial neural networks based on restricted Boltzmann machines (RBMs) then provide distinct representations of the relevant image features for tumor classification. In the final layer, a support vector machine (SVM) uses this data to differentiate the lesions.
As a result, the group's method can automatically extract and learn from SWE image features and then classify benign and malignant breast lesions, according to the group.
"No specific features need to be manually identified by users and the training set is used by the [deep-learning] network to learn the inherent task-relevant patterns," the authors wrote.
To test the technique, they performed a retrospective study that included 121 female patients with a total of 227 images; some patients had multiple lesions and images were acquired for each lesion. There were 135 images of benign tumors and 92 images of malignant tumors, as determined by pathologic results following biopsy. All shear-wave elastography exams were performed by experienced radiologists on an Aixplorer ultrasound system (SuperSonic Imagine).
After subtracting the B-mode grayscale image from the composite color image to create a "pure" SWE image, the images were then downsampled in size and transformed from a matrix into a vector of pixels. These pixel values were used as input for the deep-learning network.
Accuracy improvements
In testing, the researchers found that their deep-leaning architecture outperformed other deep-learning approaches, as well as a method based on statistical features manually extracted from the "pure" SWE images, principal component analysis (PCA) for feature reduction, and an SVM classifier.
Breast tumor classification performance on SWE | ||||
Sensitivity | Specificity | Accuracy | Area under the receiver operator characteristics (ROC) curve | |
Method based on statistical features, PCA, and SVM | 81% | 92.8% | 87.6% | 0.902 |
Deep-learning technique | 93.4% | 97.1% | 93.4% | 0.947 |
All differences were statistically significant (p < 0.05).
"It is shown that breast tumors have apparent diversities: Benign tumors seem to be mainly covered by uniformly blue (i.e., low and homogeneous elastic moduli), while malignant tumors present with abundant and mixed colors (i.e., high and heterogeneous elastic moduli), especially at the margins of tumors, representing increased and heterogeneous stiffness at peritumoral tissues, which was called the stiff rim sign," the authors wrote. "The different elastic modulus distributions between benign and malignant breast tumors were efficiently utilized in our [deep-learning] architecture."
The researchers acknowledged that their deep-learning methods required a training time of 4,200 seconds for the dataset of 227 images. They judged this length of time to be acceptable, however, on their limited hardware resources.
In addition, CADx methods relying on statistical features require "semiautomatic image segmentation for delineating tumor borders and prior expert knowledge of breast tumor elasticity, which complicates the diagnosis procedures," they wrote. "Our [deep-learning] architecture does not need image segmentation or prior knowledge, and thus it may be more convenient and suitable for future clinical diagnosis."
Future work
The researchers said that future studies of their deep-learning architecture should be conducted on a larger group of patients with various tumor subtypes, so that it can adapt and classify subtypes of breast tumors and evaluate histopathologic severity. Their software could also be easily applied to diagnosing other diseases, according to the group.
"It only needs to be retrained on samples of the target diseases, and then it will build a new network for the new application," they wrote.
The researchers also believe it could also be adapted for use with multiple ultrasound techniques, including B-mode, Doppler ultrasound, contrast-enhanced ultrasound, and strain elastography.
"Combining multiple modalities could be valuable for diagnosis of breast tumors and other diseases and thus the deep learning with collaboration on multiple modalities could improve the classification performance," they wrote. "The combination of [deep learning] with multiview learning seems to be a promising technique for such a purpose."