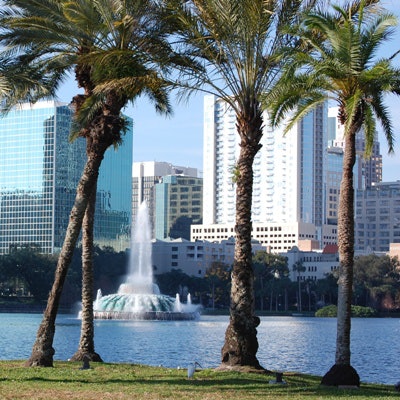
ORLANDO, FL - After analyzing quantitative information from breast ultrasound exams, machine-learning technology can achieve high sensitivity and specificity for breast cancer, according to research presented on Monday at the American Institute of Ultrasound in Medicine (AIUM) meeting.
Researchers from the University of Pennsylvania trained a machine-learning algorithm using quantitative grayscale and color Doppler features extracted from ultrasound images of more than 150 solid breast masses. They found that the software could achieve more than 90% sensitivity and specificity for breast cancer.
"Adding Doppler vascularity features to sonographic morphologic features markedly improves the diagnostic performance of machine-learning algorithms for breast cancer diagnosis," said presenter Dr. Laith Sultan. He shared the team's findings during a research session on Monday.
Quantitative Doppler
While abnormal angiogenesis is commonly linked with malignant breast lesions, breast ultrasound studies do not routinely utilize quantitative Doppler information for characterizing lesions, Sultan said. To assess the role of quantitative Doppler ultrasound in enhancing the diagnosis of breast cancer, the research team used machine-learning methods based on computerized training and testing of image features.
Quantitative grayscale and color Doppler features were extracted from margins defined by users in 160 biopsy-proven solid breast masses. The eight computerized grayscale features describe lesion morphology and margin sharpness:
- Angular variation
- Brightness difference
- Margin sharpness
- Axis ratio
- Depth-to-width ratio
- Radius variation
- Skelton norm
- Tortuosity
Three vascularity features were analyzed on color Doppler: vascular fraction area, flow velocity index, and flow volume index. The breast lesions' vascular features were measured in three regions on the color Doppler image: the center and rim of the lesions, as well as the surrounding area, Sultan said.
Training
The grayscale features with the patients' ages and the color Doppler features were used independently to train the deep-learning system using a logistic regression classifier. Cross-validation was performed using the leave-one-out testing method. They also measured the dispersion of regression coefficients from the mean.
"Those cases with the highest dispersion represented weak learning or low-confidence diagnosis and were pruned [from training]," Sultan said.
Next, the researchers assessed the method's diagnostic performance by performing receiver operating characteristic (ROC) analysis and measuring the area under the curve after each round of training. They also calculated sensitivity and specificity at the Youden index threshold for each ROC curve.
Sultan and colleagues found that the age of patients with benign lesions (45.9 ± 13.4 years) was significantly younger than the age of patients with malignant lesions (57.8 ± 12.1 years). They also discovered that the diagnostic performance of the machine-learning algorithm improved as more features were added and when the 18 cases (12%) with the highest dispersion values were removed from the training process.
Diagnostic performance of machine-learning algorithm | |||||
Trained only with grayscale features | Trained with grayscale and color Doppler features | Trained with grayscale and color Doppler features and using only selected cases | |||
Area under the curve | 0.85 ± 0.03 (95% CI: 0.79-0.91) | 0.89 ± 0.03 (95% CI: 0.83-0.94) | 0.96 ± 0.01 (95% CI: 0.92-0.99) | ||
Sensitivity | 87% | 79% | 92% | ||
Specificity | 69% | 89% | 95% |
"Our analysis identified about 12% of cases that do not contribute to the learning process and had high dispersion values," Sultan said. "When pruned, a very high diagnostic performance was achieved."
The image analysis software is already in use in the institution's lab, Sultan said.
"As a future vision, the application of these systems in ultrasound devices to be used in regular clinical settings would be of great value in providing an independent second opinion for diagnosis," he said. "This would be of substantial importance, especially in underserved regions with low expertise by providing second confirmatory, highly accurate computer-aided diagnosis."