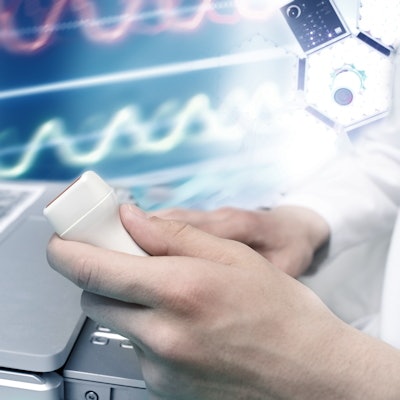
An AI assistance system can aid novice sonographers in assessing the rotator cuff, a study published December 1 in Ultrasound in Medicine & Biology found.
Researchers led by Rui Tang from Peking University Third Hospital in Beijing, China, found that their AI system can accurately identify standard planes and perform automatic tissue segmentation.
“Remarkably, the system demonstrated superior performance compared with similar studies for both functions,” Tang and colleagues wrote.
While ultrasound is a go-to method for assessing shoulder joint diseases, it is a user-dependent modality. Novice ultrasonographers may struggle with imaging the shoulder since they need a large understanding of the anatomical distribution characteristics of local structures within the shoulder region.
Previous studies have demonstrated the use of deep learning in diagnosing muscle status using CT, MRI, and ultrasound images to detect rotator cuff tears.
Tang and co-authors tested the performance of an AI assistance system for shoulder ultrasound imaging. They developed the system by using a standard plane recognition module based on the ResNet50 network and an automatic tissue segmentation module using the Mask R-CNN model. The team used a dedicated data set of shoulder joint ultrasound images to assess the model’s use in clinical practice.
The researchers found that the standard plane recognition model, which used 59,265 ultrasound images, achieved a recognition accuracy of 94.9% in the test set. This included an average precision rate of 96.4%, a recall rate of 95.4%, and an F1 score of 95.9%.
The researchers also found that the automatic tissue segmentation model, tested on 1,886 ultrasound images, achieved an average intersection over union value of 96.2%. This indicates robustness and accuracy, they noted.
Finally, the team found that the model achieved mean intersection over union values exceeding 90.0% for all standard planes. It highlighted that this indicates the model’s effectiveness in describing the anatomical structures.
The study authors suggested that the system can serve as an aid for assisting novice sonographers in shoulder ultrasound scanning, as well as offer support to ultrasound practitioners in diagnosing shoulder diseases.
“When compared to traditional approaches, the system demonstrates the ability to rapidly classify standard planes and automatically locate and segment tissues without requiring manual intervention,” they wrote. “This is expected to significantly reduce the learning curve associated with shoulder joint ultrasound, while simultaneously enhancing the quality and efficiency of sonographers in screening for shoulder joint diseases.”
The full study can be found here.