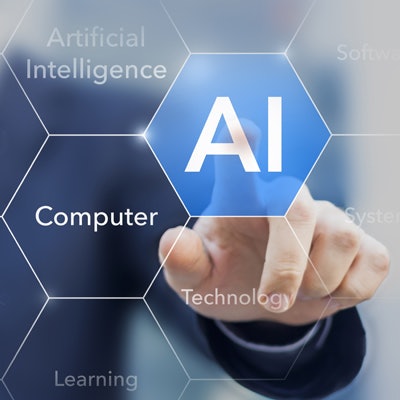
An artificial intelligence (AI) algorithm can improve the specificity of mammography interpretation without affecting sensitivity and reduce the mammography workload for radiologists by nearly one-fifth, according to a new study published online August 6 in Radiology.
How can this be accomplished? By eliminating cancer-free mammograms from the workflow, say researchers from the Massachusetts Institute of Technology (MIT) in Cambridge, MA, and Harvard Medical School in Boston.
"In our simulated triage workflow, in which radiologists would only read mammograms above the cancer-free threshold, our model showed a workload reduction of 19.3%, a significant improvement in specificity, and a noninferior sensitivity," wrote a team led by Adam Yala, a doctoral candidate at MIT.
Radiologists' diagnostic performance for reading mammograms can range widely, and practices have tried a variety of technologic and workflow solutions to address the problem, including computer-aided detection (CAD), Yala and colleagues noted. But CAD can't necessarily fix limitations in radiologist sensitivity and specificity.
The researchers hypothesized that a deep-learning model trained to identify and eliminate cancer-free mammograms from the workflow would improve radiologist efficiency and specificity without negatively affecting sensitivity.
Their study included 223,109 consecutive screening mammograms performed in 66,661 women between January 2009 and December 2016; the researchers obtained cancer outcomes via data from a regional tumor registry. This total number of mammograms was split into three separate groups:
- 212,272 for training the deep-learning algorithm to eliminate cancer-free exams
- 25,999 for validating the algorithm
- 26,540 for testing the algorithm
The group simulated a deep-learning workflow in which radiologists did not read mammograms triaged as cancer-free and read only those with suspicious findings. The researchers then calculated radiologists' sensitivity, specificity, and percentage of mammograms read.
The team established a test reading set for the radiologists that included the 26,540 mammograms for testing the algorithm from 7,176 women. Yala and colleagues found that the deep-learning algorithm workflow performed comparably to or better than radiologists reading the mammograms without using the algorithm -- with the radiologists reading 80.7% of the mammograms (that is, cancer-free exams being excluded), for a reduction in workflow of 19.3%.
Radiologist reading performance without and with deep-learning algorithm | ||
Performance measure | Without deep-learning algorithm | With deep-learning algorithm |
Sensitivity | 90.6% | 90.1% |
Specificity | 93.5% | 94.2% |
Only the improvement in specificity was statistically significant (p = 0.002). The model also had similar predictive accuracies by area under the receiver operating characteristic curve for all age groups, races, and breast densities, the team found.
The study takes a different approach to prior work on CAD by clearing cancer-free mammograms from the worklist rather than marking those with suspicious features, the group noted.
"Instead of annotating images to draw added attention to potentially malignant findings (to improve sensitivity), we propose to triage cancer-free mammograms from the workflow to improve both specificity and efficiency," the team concluded. "Our model improved specificity, improved efficiency, and did not impact sensitivity. These two approaches (CAD and triage) may be complementary, giving more attention to mammograms that warrant it and removing attention from those that do not."