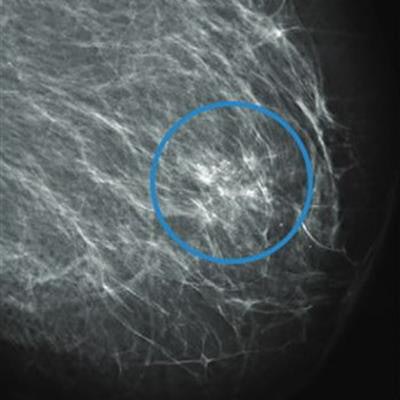
Using artificial intelligence (AI) as an aid in population-based breast screening can reduce radiologist workload by over three-fifths and avoid false-positive findings by over 25%, according to Danish research published April 19 in Radiology.
A team led by Andreas Lauritzen, a doctoral student from the University of Copenhagen, found that the AI algorithm detected and categorized normal, moderate-risk, and suspicious mammograms while also performing consistently across breast densities. The group wrote that using AI in this setting could improve breast cancer screening outcomes.
"AI-based screening would likely support most of these screening programs by increasing efficiency," Lauritzen and co-authors wrote.
Previous research suggests that population-based screening mammography reduces breast cancer mortality by detecting the early signs of breast cancer. However, this would mean more work for radiologists. Research also suggests that radiologists are more likely to miss disease signs in low-prevalence conditions.
AI has been increasingly looked at for its potential to differentiate between benign and malignant lesions on mammograms. While this technology is not able to replace radiologists, researchers have touted its potential in assisting radiologists while also relieving them of some burden.
Lauritzen and colleagues wanted to find out whether an AI-based screening protocol using a commercially available AI application (Transpara version 1.7.0, ScreenPoint Medical) could improve screening outcomes and reduce the number of mammograms read by radiologists. They also looked at the quality of AI-based and radiologist screening across BI-RADS densities.
The retrospective study sample included breast cancer screenings in 114,421 women with an average age of 59 years. The screenings resulted in 791 screen-detected cancers, 327 interval cancers, and 1,473 long-term cancers. A total of 2,107 false-positive screenings were included as well.
Comparison of AI-based breast screening performance to radiologists | |||
Radiologist | AI | p-values | |
Sensitivity | 70.8% | 69.7% | 0.02 |
Specificity | 98.1% | 98.6% | 0.001 |
The researchers found that radiologist workload fell by 62.6% to 71,585 images out of the total of 114,421. They also found that 25.1% of false-positive screenings were avoided (529 of 2,107).
While screening results were found to be consistent across BI-RADS densities, sensitivity did not reach statistical significance. Specify for AI-based screening, meanwhile, increased between 0.3 and 0.6 percentage points across all BI-RADS densities and were higher than radiologist screening specificities.
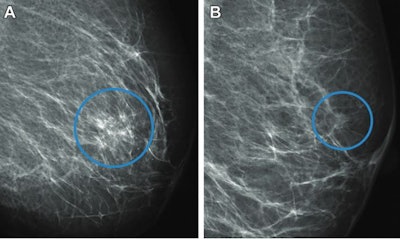
The study authors wrote that the AI-based screening protocol can be adjusted by changing the threshold for false positives. This can result in more conservative screening, or it can be used to match desired recall rates, which in turn could aid in single-reader programs, limited resource settings, or programs with nonspecialized radiologists.
However, Lauritzen et al added that this could increase reading time of suspicious mammograms for highly specialized radiologists.
"For institutions with comparable management regimens, the results of our AI-based screening protocol are likely generalizable, but further validation is needed," they wrote.
The team called for further research on thresholds and cancers that the AI misclassified, as well as a prospective trial to measure AI's impact on radiologist performance.