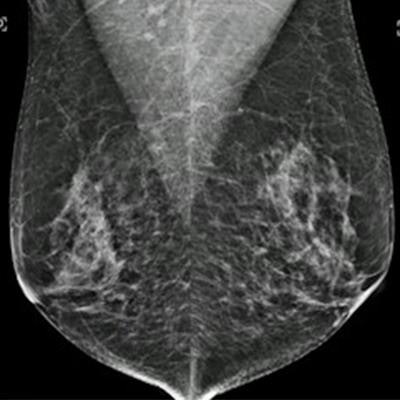
An artificial intelligence (AI) model shows near-complete agreement with radiologists for classifying breast density on mammography, suggest findings presented April 17 at the American Roentgen Ray Society (ARRS) annual meeting in Honolulu.
Presenter Dr. Lianne Philpotts from Yale University in New Haven, CT, shared study results that confirmed 99% agreement between an AI model they developed and radiologists for categorizing dense breast tissue.
"This indicates a pretty strong support for use [of AI] as an adjunct or standalone density assessment tool," Philpotts said.
Breast density can confound mammography interpretation, and the specialty continues to tackle this challenge. Conventional mammography can fail to detect cancers in dense tissue, so supplemental imaging is used to further aid in breast cancer screening.
However, breast density is not a one-size-fits-all problem. Different categories of breast density have been identified, ranging from entirely fatty to extremely dense. Although AI tools are being developed for medical imaging use, Philpotts emphasized that classifying breast density is largely subjective and susceptible to interobserver variability, making the use of AI more complex.
She and colleagues sought to assess clinical agreement between an AI tool they developed and breast radiologists for categorizing breast density on digital mammograms, either full-field or synthetic 2D. The group's model used an enterprise imaging platform (Visage 7, Visage Imaging) trained on more than 33,000 mammograms from Yale. These were interpreted by breast radiologists, and the AI classifier was based on radiologist reports using BI-RADS fifth edition.
From there, radiologists interpreting screening mammograms were presented with an automatically displayed window of breast density with BI-RADS categories A through D from the AI platform. They could either agree with the model on density category or select the category they believed was most appropriate. Images were available only on routine craniocaudal and mediolateral views, and a bilateral assessment option was also available.
The AI density display also contained confidence ratings. A value above 87.4% indicated certainty, while values between 87.4% and 73.7% indicated reasonable certainty and values below 73.7% meant uncertainty.
For the study, the team included data from 93,650 screening mammograms that had density assessment recorded by 164 readers. Out of the readers, 66 radiologists reviewed at least 100 cases each while 19 were high-volume readers who reviewed more than 2,000 cases each.
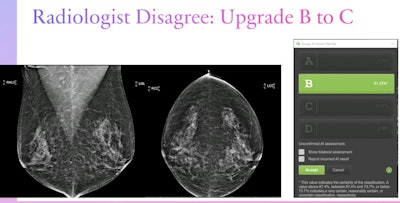
The researchers found that the AI model showed "extremely good" agreement with the radiologists, at 99.3%. The model also showed very high agreement with high-volume imaging readers, at 99.3%.
Of 606 cases where there was disagreement between the algorithm and the radiologist readers, 603 were by only one category (i.e., a change from category A to B, or category C to D).
Philpotts noted nearly equal changes in upgrading and downgrading between categories B and C, indicating a few areas of dense fibrous and glandular tissues and an almost equal mix of fatty and dense tissue, respectively. Of these, 107 cases were upgraded to category C from B while 125 were downgraded to category B from C.
"Densities right in the middle are always a challenge," she said. "This may be reflected perhaps on a bias from the radiologist or, if a patient is having screening ultrasound, they [radiologists] may tend to upgrade versus downgrading. It's hard to know exactly."
Philpotts said the model could guide supplemental screening, including more access by technologists to help with workflow optimization.
"Given recent federal legislation regarding breast density notification, determination of breast density is very important and standardized density [categorization] is needed," she concluded.