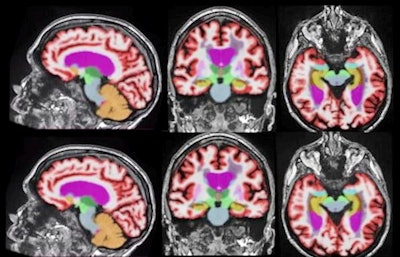
Minnies winners, page 3
Scientific Paper of the Year
Minnies 2021 winner: COVID-19 vaccination-associated axillary adenopathy: Imaging findings and follow-up recommendations in 23 women. Mortazavi S, American Journal of Roentgenology, February 24, 2021. Learn more about this study.
Working at a women's diagnostic imaging clinic at a large U.S. academic medical center during the peak of the COVID-19 pandemic had its privileges, said Dr. Shabnam Mortazavi, author of the Scientific Paper of the Year.
One was seeing patients who were among the first group to receive COVID-19 vaccines. At University of California, Los Angeles (UCLA), women began presenting with lumps under their arms after receiving vaccines and Mortazavi noted a pattern.
"We see something similar in influenza and shingles, but not to that extent," Mortazavi said.
At the time, there was just one published case report describing similar findings so she got to work on her own with the resulting paper published February 24 in the American Journal of Roentgenology.
In the study, Mortazavi included data on 23 women who began presenting at UCLA Health in December 2020 with axillary adenopathy on mammography, breast ultrasound, or breast MRI after receiving vaccinations for COVID-19.
She found a total of 13% of the women had symptoms of axillary lumps with tenderness. The adenopathy was detected incidentally on screening breast imaging in 43% of patients and on diagnostic imaging for other reasons also in 43%. Most of the adenopathy occurred with the Pfizer vaccine (52%), among asymptomatic women presenting for either screening or diagnostic purposes (86%), and on ultrasound (52%).
"This study highlights axillary adenopathy ipsilateral to the vaccinated arm with Pfizer-BioNTech or Moderna COVID-19 vaccine as a potential reactive process with which radiologists must be familiar," Mortazavi wrote in the paper.
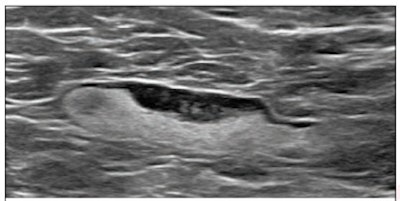
Scanning axilla during screening and diagnostic breast examinations with high-frequency, linear-array ultrasound transducers is the protocol at UCLA Health, Mortazavi noted. That isn't true at all institutions (there are different approaches), but it proved to be a second important advantage in this case, she said.
"If you don't look, you're not going to see anything," she said.
Mortazavi added she hopes to have laid the groundwork for additional studies. Today, she's not seeing as many patients presenting with axillary lumps and tenderness after receiving COVID-19 vaccines. People appear less worried than they were back in December 2020. But booster shots are rolling out and the fact remains that nobody knows exactly what will happen next.
Being able to reassure worried patients is what's most important, Mortazavi said. In that regard, she has more confidence moving forward -- after all, she now has a significant study to refer them to.
Mortazavi is an assistant professor of radiology at UCLA. She received an MD and master's degree in public health from the University of Tehran in 2011 and completed a residency in diagnostic radiology at the University of Minnesota Medical School in 2019.
Runner-up: The concept of gaslighting in the radiology workplace: Concepts and implications. Ballane B et al, American Roentgen Ray Society annual meeting, 2021. Learn more about this study.
Best New Radiology Device
Minnies 2021 winner: Spectral CT 7500 spectral CT scanner, Philips Healthcare
Spectral CT 7500 represents the latest generation of spectral CT (also known as dual-energy CT) technology from Philips Healthcare, and builds on the IQon Spectral CT scanner that the company released in 2013.
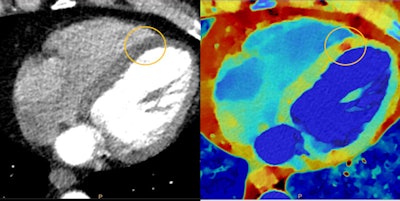
The general idea of the technology is that by acquiring images at different energy levels, spectral CT can be used to differentiate between different types of tissue, such as a malignant lesion from benign tissue. But the advancement of the technology has been slowed by perceptions that it's more difficult to perform than conventional CT.
Like IQon, Spectral CT 7500 aims to make spectral imaging easier to perform with the goal of eventually becoming a routine exam for multiple different clinical applications, according to Frans Venker, global business leader for CT and advanced molecular imaging. The company launched Spectral CT 7500 in May 2021.
In particular, Philips sees the new scanner as making spectral imaging available to a broader range of patients, such as children or those undergoing bariatric procedures. The company developed specialized pediatric scanning protocols for the former and a wider bore as well as a redesigned table for the latter.
Philips also sees a future for spectral cardiac imaging, Venker said. The company has developed new protocols to produce motion-free cardiac images, even for patients with irregular heartbeats. The company also improved the table speed on Spectral CT 7500 with the system now able to complete a head scan in 1 second and a full-body scan in 2 seconds.
Philips believes spectral imaging will lead to real-world clinical benefits that can be measured and has data from early installations of the system, such as at Westchester Medical Center in New York under the direction of Dr. Zvi Lefkovitz. In fact, an issue that was once the Achilles' heel of spectral CT -- its impact on workflow -- could become an advantage, according to Venker: The improved diagnostic confidence possible with the scans obviates the need for additional imaging studies.
Users have seen a 26% decline in the number of follow-up scans required after spectral CT and a 23% improvement in clinical confidence as well as lesion conspicuity. One site in Europe calculated that using spectral CT produced 52,000 euros in savings from not having to perform additional scans, Venker said.
Philips will continue to push the development of spectral CT with a focus on optimizing radiation and contrast while also improving the workflow of the technology.
"We are only scratching the surface of the clinical benefits but also the workflow benefits" of spectral CT, Venker said.
Runner-up: Magnetom Free.Max MRI scanner, Siemens Healthineers
Best New Radiology Software
Minnies 2021 winner: Genius AI Detection software for breast tomosynthesis, Hologic
Hologic's Genius AI Detection software for digital breast tomosynthesis exams has earned the women's imaging vendor its first-ever Minnies award as the winner of the Best New Radiology Software category for 2021.
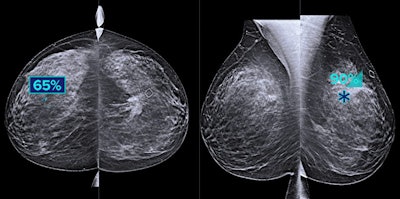
Integrated into the vendor's Dimensions digital mammography system, the deep learning-based Genius AI Detection software is designed to yield higher sensitivity and a lower false-positive rate than the firm's prior computer-aided detection software, according to Jennifer Meade, division president for Breast and Skeletal Health Solutions. Genius AI Detection was launched in December 2020.
"Genius AI Detection software aids in image interpretation by highlighting suspicious, and often subtle, areas of interest, and provides the radiologist the opportunity to prioritize the most concerning patient cases," Meade said. "Additionally, Genius AI Detection technology delivers key metrics at the time of image acquisition to help imaging sites triage patients."
Running on the acquisition workstation without the need for a separate server, Genius AI Detection provides metrics at the time the exam is acquired, helping radiologists to optimize workflow and expedite patient care by categorizing and prioritizing cases based on complexity and expected read time, according to Meade.
A Case Score indicates the confidence of the artificial intelligence (AI) software that a case contains a malignant lesion, while a Case Complexity Index categorizes exams by the number of findings it has identified. In addition, a Reading Priority Indicator flags the most concerning cases. Finally, a Read Time Indicator can be utilized to sort and select cases by relative reading times in order to balance workload across multiple radiologists, Meade said.
A multireader study conducted by Hologic with radiologists reading mammography and breast tomosynthesis image sets found that concurrent use of Genius AI Detection during interpretation led to a 9% difference in sensitivity for breast cancer detection, as well as an improvement in accuracy. Mean area under the curve for the 17 readers increased from 0.794 without Genius AI Detection to 0.825 with the use of the software, according to the company.
"Genius AI Detection technology is designed to be an indispensable support tool for clinicians that drives real and improved outcomes for patients, which is what we're all ultimately striving for with radiology software and innovation," Meade said.
Runner-up: AI computer-assisted triage software for incidental detection of pulmonary embolism on CT, Aidoc
Best New Radiology Vendor
Minnies 2021 winner: Rad AI
In contrast to the many other radiology artificial intelligence (AI) startups devoted to developing image analysis-based applications, our Minnies winner for Best New Radiology Vendor, Rad AI, has focused on streamlining workflow in nonimage-based tasks.
Rad AI's initial product is Omni, an AI application that's designed to save radiologists 60 or more minutes a day by automatically generating the "impression" section of the radiology report based on the dictated findings. The language suggested by the algorithm is customized for each radiologist and practice, said co-founder and CEO Doktor Gurson. The radiologist then reviews and signs off on the report.
Introduced in early 2021, Omni helps address burnout by enabling radiologists to dictate up to 35% fewer words per report and get a cognitive break in between reports, according to the company. Impressions are generated in 0.5 to 3 seconds, depending on the complexity of the study. As this process is entirely automatic, it doesn't affect the radiologist's workflow, Rad AI said.
In addition, Omni can improve report quality and accuracy for example by automatically including consensus guideline recommendations. The software also ensures the report answers the ordering clinician's main question and includes any significant incidental findings, the firm said.
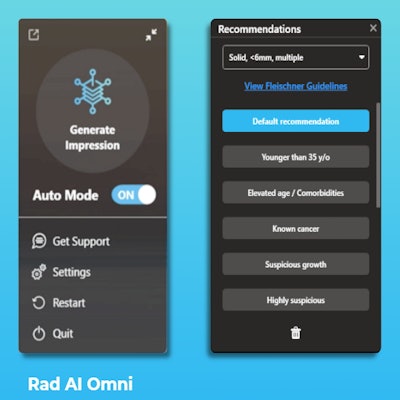
Notable Omni customers over the last year include Star Teleradiology in Tampa, FL; Radiology Associates in Little Rock, AR; Inland Imaging in Washington and Montana; Radiology Associates of North Texas; Radiology Inc. in Huntington, WV; Quantum Imaging & Therapeutic Associates in Lewisberry, PA; Carle Health in Urbana, IL; and Steinberg Diagnostic Medical Imaging in Las Vegas.
There's also more to come for Rad AI. The company, which has recently finalized a new round of funding, is preparing to launch its second product -- Continuity -- in the fourth quarter. Geared toward health systems, Continuity automatically identifies follow-up recommendations for a wide range of incidental findings, assesses clinical appropriateness based on national consensus guidelines and health system requirements, and specifically tracks appropriate follow-ups, according to the vendor.
Continuity also automates follow-up activities such as orders for imaging studies and lab tests, as well as referrals for outpatient provider confirmation. Patient communication can be performed using automated text messages, direct mail, and electronic health record-based messages, while a mobile-friendly provider portal can be used by physicians to track pending follow-ups and to confirm orders.
In addition, Continuity features dashboards to provide users with population health insights and confirms the health system's return on investment, according to Niven Shah, who works on business development at Rad AI.
Runner-up: Prescient Imaging
Best Educational Mobile App
Minnies 2021 winner: Radiology Assistant 2.0, BestApps (iOS)
A five-time finalist, Radiology Assistant 2.0 has been awarded the Minnie for Best Educational Mobile App for the second time.
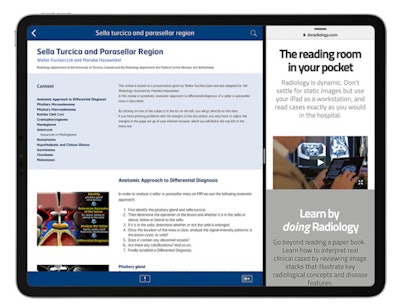
Developed by Dutch radiologists Dr. Wouter Veldhuis, PhD, of the University Medical Center Utrecht and Dr. Robin Smithuis of Rijnland Hospital Leiderdorp, Radiology Assistant 2.0 features peer-reviewed articles written by expert radiologists. Smithuis is also the founder of the Radiology Assistant educational website and nonprofit organization.
Radiology Assistant 2.0 previously won Best Educational Mobile App in 2018 while its predecessor, Radiology Assistant, was a finalist in 2013. The app has been updated frequently over the last few years with many new articles to help radiologists read COVID-19 studies as well as handle uterine anomalies, lung fibrosis, gallstone disease, ankle fractures, Crohn's disease, breast ultrasound, and CT staging of pancreatic cancer, according to Veldhuis.
New content is also in the works, including the third version of its article on MRI staging of rectal cancer. Articles on craniosynostosis and trigeminal neuropathy will also soon be added, according to Veldhuis.
"Our plans are to keep the app running and updated for the coming years as we have done for the past decade," Veldhuis said.
This effort has taken a lot of work for Veldhuis and Smithuis. The app code has been kept up to date since 2011, and it was completely rewritten in 2017 for the launch of Radiology Assistant 2.0, Veldhuis said.
"It continues to require updates to handle changes to mobile OS and device capacities," he said. "Just like all the articles, including all artwork, everything is original content."
Although free to download, the app does require a subscription to access article categories. In-app purchases are supported, and the fees will go toward a good cause. After costs for servers and hosting have been covered, all other proceeds from the subscriptions continue to be donated to the Medical Action Myanmar medical aid organization, which was co-founded by Smithuis' brother, Veldhuis said.
Runner-up: RadDiscord, a server on Discord
Best Radiology Image
Minnies 2021 winner: Deep learning-based image reconstruction of brain MRI scans maintains accuracy of volumetric quantification while enhancing image quality
While most of the attention around artificial intelligence (AI) has focused on its use for image analysis and interpretation, many radiologists are even more excited about AI's potential for improving image reconstruction.
The winner of this year's Best Radiology Image category is an excellent example. The winning image is a series of volumetric quantitative MRI scans, one acquired and reconstructed with a standard protocol, while the second series was acquired with a 60% shorter scan protocol and then processed with a deep-learning algorithm to enhance its image quality and quantitative accuracy.
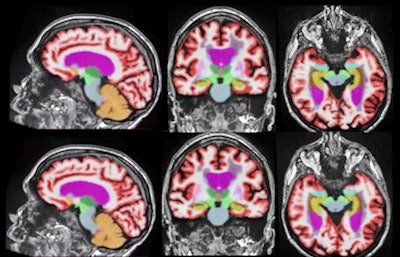
Can you tell the difference? Neither could a group of expert readers. And that's the whole idea -- deep learning-based reconstruction has enabled the production of sophisticated images at speeds that are better tolerated by patients, according to Dr. Suzie Bash, medical director of neuroradiology at San Fernando Valley Interventional Radiology & Imaging Center, a RadNet facility. Bash was lead author of the study, and she presented the findings at the 2021 edition of the European Congress of Radiology.
Bash said she has been applying quantitative metrics to MRI scans for the past 15 years, and in the last year and a half has become involved with deep-learning reconstruction. Despite the clinical benefits of quantitative volumetric MRI, some imaging facilities are hesitant to use it due to concerns about the complexity and time involved in acquiring the scans.
In the winning image series, T1-weighted images were acquired with an accelerated protocol that was 60% faster, then the data were processed with image reconstruction software (SubtleMR, Subtle Medical). Finally, automatic brain lesion quantification software was applied (NeuroQuant, CorTechs Lab) to both the enhanced accelerated images and the standard-of-care images.
Both series of images were analyzed by blinded neuroradiologist readers, who actually found the images acquired with the shorter protocol to be superior to the standard of care. Bash et al then applied a quantitative measure to both series to ensure there weren't any alterations to the data that would affect quantitative measurements. There were none.
"Not only did the images look better, but we knew we weren't changing or distorting them in any way," Bash said.
Bash and her colleagues have submitted the paper presented at ECR 2021 to the American Journal of Neuroradiology, with publication expected in the next few months. For future studies, she plans to investigate the accelerated protocol on patients with multiple sclerosis (the current studies involved both cognitively normal patients as well as those with mild cognitive impairment and Alzheimer's disease).
Bash believes that the study confirms that deep learning-based reconstruction can enable the acquisition of quantitative volumetric MRI images at speeds that will be better tolerated by patients. She also believes that deep learning-based reconstruction has a bright future.
"My prediction is that deep learning reconstruction will be the standard of care," she said. "It will be routine. I don't see any downsides to it."
Runner-up: PET images of abnormal radiotracer uptake in the lymph nodes of cancer patients after they received COVID-19 vaccinations