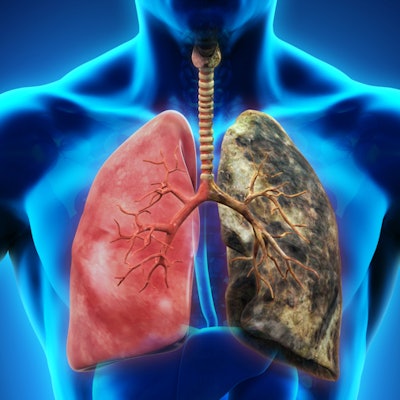
Applying a deep-learning algorithm to PET/CT imaging results can help clinicians distinguish between tuberculosis (TB) and lung cancer, according to a study published May 14 in Thoracic Cancer.
The most effective algorithm is one that integrates a variety of "inputs," wrote a team led by Xiaolei Zhang, PhD, of the Universiti Putra Malaysia in Serdang.
"The use of [a multiple input deep learning model was] shown to be more effective for the classification of lung cancer and pulmonary nodules compared to models with single inputs ... [and was] capable of providing valuable auxiliary diagnostic information to assist physicians in accurately distinguishing between tuberculosis nodules and lung cancer," the group reported.
Tuberculosis and lung cancer can manifest in similar ways -- which can lead to misdiagnosis of lung cancer, Zhang and colleagues cautioned.
"When lung cancer is misdiagnosed as tuberculosis and treated as such, not only will the patient's condition not improve, but it may also lead to lymph node metastasis and distant metastasis," they wrote.
PET/CT is a valuable tool for the diagnosis, staging, and treatment assessment for cancer. Using artificial intelligence with PET/CT can further improve its efficacy when it comes to distinguishing between cancer and other pulmonary conditions, according to the investigators.
"The integration of deep learning models with radiomics is becoming increasingly common in the processing of medical image information," they wrote. "However, multi-input deep learning models that integrate multidimensional information are infrequently reported in the literature."
To address this gap, the team conducted a study that included a total of 174 patients (97 with lung cancer and 77 with tuberculosis). The authors used a deep-learning algorithm to extract 100 radiomic features from both PET and CT imaging as well as 2,048 deep-learning features from a residual neural network, then created and tested the following four models:
- A machine-learning model with radiomic features (traditional)
- A deep-learning model with image features (DCNN)
- A deep-learning model with both radiomic features and deep-learning features (radiomics-DCNN)
- A deep-learning model with radiomic features, deep-learning features, and clinical information
The team evaluated the models for area under the receiver operating curve (AUC), sensitivity, specificity, and F1 scores (a machine learning metric that is used in classification models).
The group found that the integrated model was the most effective for distinguishing between lung cancer and tuberculosis.
Performance of four deep learning algorithms to distinguish between tuberculosis and lung cancer on PET/CT imaging | ||||
Measure | DCNN | Traditional radiomics | Radiomics-DCNN | Integrated model |
Sensitivity | 71% | 77% | 84% | 85% |
Specificity | 71% | 76% | 83.3% | 84% |
F1-score (1 as reference) | 0.70 | 0.75 | 0.82 | 0.84 |
AUC (1 as reference) | 0.72 | 0.76 | 0.82 | 0.84 |
The integrated deep-learning model shows promise for improving PET/CT results when it comes to identifying lung cancer, according to the researchers.
"The key contribution of this study is the design of a new deep learning model as the classifier, which is believed to be better equipped to integrate information from different dimensions," they concluded. "The proposed deep learning model integrated multiple dimensions of information, including radiomic features, deep learning features, and clinical data."