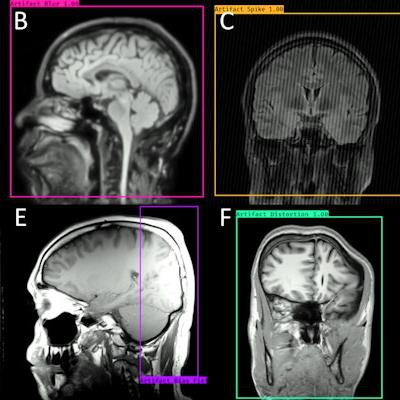
TORONTO - Scientists at Philips have developed an AI algorithm called VitaLenz that can automatically detect artifacts on MRI scans, according to a June 4 presentation at the International Society for Magnetic Resonance in Medicine (ISMRM) meeting.
Presenter Joel Batey, a MR clinical consultant at Philips, said that the algorithm -- called VitaLenz -- is a real-time object detection convolutional neural network (CNN) trained on 4,606 brain images. Its purpose is to assist MR technologists during scanning.
"As new technology to accelerate image acquisition is developed and productivity demands are increased, it is imperative that technology that assists the MR technologist is created in parallel," he said.
Advances in MR acceleration techniques have produced a paradigm shift in MR productivity and the integration of AI offers even more promise to integrate MR workflow and accelerate image acquisition, Batey said.
However, there is an absence in operator-assisted technologies, he noted. Thus, he said his group at Philips explored ways to fill this void -- specifically by developing an AI model for detecting common MRI artifacts.
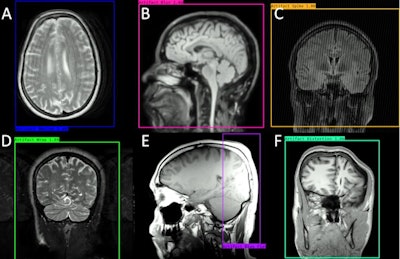
The model was trained on 4,606 brain images made up of three image contrasts (T1-weighted, T2-weighted, and FLAIR) and three imaging planes (axial, sagittal, and coronal). These images were then augmented to produce six common image artifacts: motion, wrap, blurring, bias field, RF spike, and geometric distortion.
Next, the model was tested on 570 MR images with and without artifacts and evaluated for sensitivity, specificity, and accuracy. In addition, a subset of test images was evaluated by eight registered MR technologists with an average of 17.2 years of experience to compare the model's performance versus human readers.
According to the analysis, VitaLenz showed similar performance compared with the human reader group in sensitivity and accuracy.
Comparison between readers and AI for detecting artifacts on brain MRI | ||
Readers (n = 8) | AI (VitaLenz) | |
Sensitivity | 85% | 81.25% |
Specificity | 86.96% | 66.67% |
Accuracy | 86.05% | 74.19% |
In addition, VitaLenz processing took on average 0.477 seconds per image to identify, label, and locate artifacts, Batey noted.
"This is almost eight times faster than the average time spent by readers to evaluate an image," he said.
This was a proof-of-concept study, Batey said, with his group concluding that VitaLenz was able to identify MR image artifacts with high sensitivity, accuracy, and speed. Ultimately, while the model showed significantly lower specificity, its high sensitivity means that it could potentially be used to identify images with artifacts in them that may need to be rerun or adjusted, according to Batey.
Moreover, the speed at which VitaLenz can evaluate images is significantly higher than that of the human readers and not subject to the fatigue of evaluating thousands of images that are acquired throughout a normal technologist's shift, he added.
"As MR image acceleration techniques advance, it is important to co-create tools that assist the technologist to ensure patient care and image quality is maintained," Batey concluded.