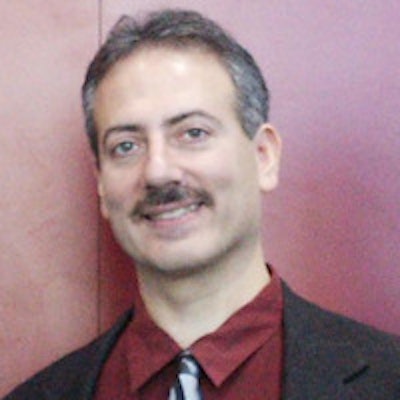
Clinical adoption of computer-aided detection (CAD) software has plateaued and has largely been confined to mammography. To change that, next-generation CAD software will need to deliver efficiency gains, interactivity, and algorithms that are personalized for patients, according to Dr. Eliot Siegel of the University of Maryland.
For example, CAD must evolve from being an inflexible tool that marks a suspected area of abnormality to an application that interacts with the user and provides a confidence level and rationale for the reason that it was marked, Siegel noted.
Furthermore, the next generation of CAD will move to a "paradigm in which CAD detection and diagnosis algorithms become more personalized and take into consideration additional facts about the patient, lung nodule characteristics, and previous exams rather than acting as though they have blinders on about all the information now routinely available in the [electronic medical record], previous studies, and previous imaging reports," Siegel told AuntMinnie.com. "This will ultimately change CAD into a tool that becomes an essential part of our everyday practice."
Siegel discussed the clinical and research perspective on challenges in CAD development and commercialization during a keynote session at the recent SPIE Medical Imaging 2014 meeting in San Diego.
Failure to launch
Despite a vast body of research showing CAD's benefits in a range of clinical applications, incorporation of the technology into clinical practice has been disappointingly slow. Reimbursement is only available for use with mammography, and it's difficult to otherwise make the business case to radiologists to spend more money on CAD when they are already experiencing low margins, Siegel said.
"There is major pressure related to decreased reimbursement, and the impression is that reimbursement will continue to drop," he said.
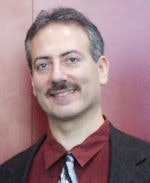
Siegel also noted that there is much skepticism among his colleagues about the added value of CAD. Many only use it for mammography because that's the only clinical application for which reimbursement is routinely available, he said.
"Radiologists will not pay any significant amount for, say, CAD lung nodule detection for chest radiography, even with a hypothetical scenario of 10% or even 20% increase in sensitivity," he said. "I believe that they would pay more, however, for something that increased their efficiency and productivity."
One of the biggest barriers to widespread clinical adoption of CAD beyond mammography is that CAD applications are currently not built into workflow, Siegel said. As it exists today, CAD requires additional steps and has an unwieldy interface, followed by manual and error-prone insertion of results into an imaging report via dictation.
"Additionally, there is a relatively high barrier for [CAD] programs to reach the marketplace, including perceived [U.S. Food and Drug Administration] challenges, the difficulty in integrating an application -- even a really good one -- into an interpretation workstation, and the associated difficulty in developing a workstation around a single CAD application," Siegel told AuntMinnie.com. "There are many excellent CAD applications that have been presented at scientific meetings, but most of these do not integrate into a typical reading environment."
Clinical enhancements
In terms of clinical benefits, the next generation of CAD software will need to improve radiologist efficiency and productivity, as well as increase accuracy and reliability without compromising efficiency, Siegel said. It should also be affordable, raise confidence, and will allow radiologists to measure things they couldn't otherwise measure, such as liver or pulmonary "texture," he said.
In a way, CAD could function in a manner analogous to a resident or a fellow in an academic practice.
"The resident or fellow looks through the chart and old reports and pathology, and summarizes at the image interpretation workstation what is important for me to know in a way that is context-sensitive," Siegel said.
This automated capability would require tight integration with the electronic medical record (EMR) -- or a system that represents the EMR -- or would necessitate additional systems that can mine data from one or more EMRs, RIS, and other information systems in an enterprise, he said.
Siegel predicts that computer algorithms will increasingly and routinely be utilized in an unsupervised or semisupervised manner to gather supplemental information, such as quantification of bone mineral density, interstitial lung disease severity, the presence of an aortic aneurysm, and assessment of coronary artery calcification.
"This will allow radiology data to be discoverable by the increasing number of decision-support algorithms that will be incorporated into the routine practice of medicine," he said.
Reporting templates such as those from the RSNA radiology reporting initiative could then utilize the results from these algorithms. Furthermore, the Annotation and Image Markup (AIM) standard offers a means of adding information to an image in a clinical environment, opening the doors to a future in which image content can be easily and automatically searched, according to Siegel.
An imaging 'physical' exam
Computer algorithms could also be employed to create an imaging "physical" exam, extracting additional information from routine or screening imaging exams. Data could be gathered on cardiac output and lung parenchymal texture; body fat; bone mineral density; hepatic, splenic, and renal function; hepatic fat and texture; edema; vascular collaterals; gastric inflammation; and dozens of other parameters that could be important in current or future patient care, Siegel said.
For quantitative imaging like this to be used in clinical practice, it can't decrease productivity, he said. It should provide lesion tracking over time, and it would be a major advance to also get an estimation of error of measurement.
Quantitative imaging and CAD can also facilitate personalized medicine. For example, after CAD discovers a lung nodule, it could then extract morphology, lesion volume, and other pixel-based data. These data could then be analyzed and compared with reference data such as from the National Lung Screening Trial (NLST) to provide an estimate of the likelihood of malignancy using similar patients with similar nodules or to a local hospital or network database, he said.
"The next generation of CAD will reflect the trend toward big data and personalized medicine, and shift away from the current second-reader approach and toward one in which CAD algorithms increasingly serve as visualization and image measurement/annotation and quantification tools," Siegel said.
Examples of this include probability maps, lesion tracking, highlighting certain findings to draw attention to the reader, and segmentation of structures such as the liver to provide data on size, he said.
Challenges
CAD applications must be able to be integrated into the image acquisition, display, and interpretation workflow; they won't be adopted if they constrain the throughput of the radiologist, Siegel noted.
CAD needs to also yield a high level of accuracy in individual patients, not just to demonstrate efficacy in separating two groups of patients (i.e., those with cancer and those without). Furthermore, commercialization and FDA clearance of CAD products are currently big hurdles and need to be revisited, he said.
Siegel also envisions an "Apps Store" for next-generation CAD algorithms, enabling their use on a single platform. He would also like to see a business ecosystem that could support, for example, downloading of algorithms on the fly, or tools that generate consensus from multiple CAD programs.
In the future, Siegel predicts increasing utilization of CAD for lung nodule detection and in other thoracic applications such as detecting pulmonary emboli. In addition, CAD software will see use in brain volume measurements, vascular applications such as quantifying coronary artery calcification, virtual colonoscopy, and lymph node detection, Siegel said.
"We will soon also see automated, unsupervised applications that detect a checklist of different findings and automatically populate those in imaging reports," he said.
Recommendations
Siegel stressed the importance of high-quality radiologist training to optimize the use of CAD. Training should also include discussion of the technology's pitfalls, he noted. In addition, CAD results should be presented by color code and other visual or even auditory cues according to probability/confidence rather than just marking the candidate lesion, he said.
"[We should also] evaluate the many advantages associated with an interface and host workstation such as that proposed by DICOM Working Group 23 to allow a variety of different algorithms to run on a single host workstation so [that users] don't have to purchase a separate workstation and server for each application," Siegel said. "This could enable a business ecosystem that would give smaller companies and developers the opportunity to develop innovative software that could run on a workstation in a manner analogous to the Apple and Android applications."
CAD has largely been employed in a second-reader mode rather than its future likely role as a visualization tool that can be toggled on and off in a more collaborative image-analytic approach. Also on Siegel's wish list would be for CAD to perform image recognition before a study is reviewed to screen for a broad range of abnormalities such as rib fractures, compression spine fractures, pneumothorax, etc.
Siegel also pointed out that "image analysis is still a really difficult task, even in the age of [IBM's] Watson [project] and Siri."
"I can teach an 8-year old child to find the adrenals as consistently in less than 15 minutes," he said. "I have never seen anybody successfully tackle the problem of automatically finding and segmenting the adrenals. I'll wash the car of the first developer who can create a program that finds them more consistently than that 8-year old."