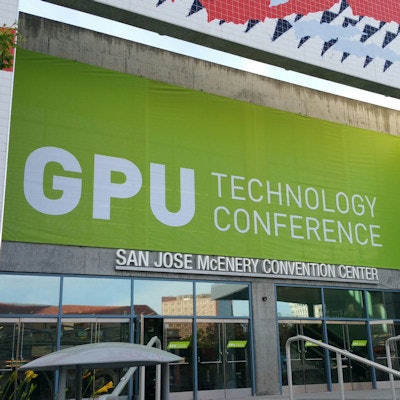
SAN JOSE, CA - Physicians and machines will work better together rather than separately when it comes to deploying artificial intelligence (AI) for analyzing medical images in the years to come, according to a series of presentations on May 9 at the NVIDIA GPU Technology Conference (GTC).
In a track devoted to AI applications in healthcare before an audience of mostly Silicon Valley software developers, prominent radiologists including Dr. Keith Dreyer, Dr. Curtis Langlotz, and Dr. Daniel Rubin elucidated their vision of human clinicians working hand in hand with computers to solve some of the thorniest problems in healthcare, addressing medical errors, overutilization, and more-targeted screening.
Rather than allowing computers to replace radiologists, they believe AI and related concepts such as machine learning and deep learning will leverage the strengths of both to make medicine more precise, efficient, and standardized.
A dark vision of the future
The arrival of artificial intelligence in the past several years has prompted a number of social theorists and computer experts to conjure a dark vision of the future in which computers replace humans for many tasks, including some for which humans train for years to become proficient -- like radiology.
Speaking just a few miles from where driverless cars are being pioneered, Dreyer of Massachusetts General Hospital began his talk by reviewing a controversial statement from computer scientist Geoffrey Hinton made in October 2016. Hinton said the use of AI algorithms for interpreting radiology images would make the specialty obsolete in five to 10 years, and "we should stop training radiologists now."
But Dreyer said he doesn't see AI in such zero-sum terms. Interpreting images is just one part of the average radiologist's responsibilities; a computer algorithm that only performs that task will hardly replace radiologists.
"I don't look at AI inside of healthcare to automate interpretation," Dreyer said. "I tend to think of it as to improve outcomes in patient care. You have to think beyond just creating the algorithm."
While you could apply AI to reduce the costs of radiologist interpretation -- or the professional component, to borrow language from healthcare economics -- that represents just 20% of the cost of delivering imaging services, with the rest of the expense made up by the technical component, or the work involved in running the machines and performing procedures.
What's more, technology has already been used to eliminate many of the repetitive, manual tasks that once consumed significant resources -- such as reporting via transcription -- and yet there are still radiologists.
Dreyer believes there are a number of areas in which AI will help radiologists in the future, in particular with decision support. Many radiologists interpret images using gut findings based on their training, but surveys performed by the American College of Radiology (ACR) have shown that radiologists will follow decision-support guidelines if they are available, as would be possible with artificial intelligence, he said.
A good example is BI-RADS, where reports are highly structured because they are required to be that way by the Mammography Quality Standards Act (MQSA). Implementing such structured reporting throughout the rest of radiology would confer many benefits in terms of being able to apply automated analysis to reports.
"Wouldn't it be great if [the radiology report] was automated and you create AI to analyze that?" he asked.
To make this vision a reality, Massachusetts General Hospital and sister institution Brigham and Women's Hospital in 2016 created the Clinical Data Science Center under Dreyer's direction to help guide the translation of AI algorithms from the development phase to clinical use. The center offers an environment in which algorithms can be run in a research setting and trained on massive clinical datasets that have been validated through radiologist interpretation to represent the ground truth for particular conditions.
Dreyer acknowledged to the audience that figuring out which health conditions to target first was a challenge, given the myriad diseases, modalities, and variables that come into play. However, initiatives such as the clinical challenges being sponsored at GTC 2017 offer forums for leveraging the strengths of clinicians and algorithm developers.
Dreyer concluded with a request to radiologists who are skeptical of artificial intelligence to take another perspective.
"Ask for AI that augments [and] not just automates your solutions," he said. "It's not man versus machine; that's what's got to come out of people's minds. This is going to be man with machine, versus man without machine. I want to be the one who has the machine so that I can make my practice run better."
AI and autopilot
Taking a similar position to Dreyer was Langlotz of Stanford University, who described emails he receives from medical students who are considering radiology as their area of specialization but are concerned about whether it is a viable profession.
As an example, Langlotz pointed out that airline pilots also make heavy use of technology, but when he asked the GTC 2017 audience how many would want to fly in a self-piloted airplane, few hands went up.
"It's a highly perceptual activity; it's a high-risk activity. Human lives are at stake," Langlotz said of airline pilots. "Much of it is routine, but there are clearly times when rapid judgment is required. I'm glad to have autopilot. I'm glad to have all the technology that can summarize all the data that's coming from the airplane ... but I'm also glad that there's somebody there who can run the controls when that's necessary."
Echoing the theme was Dr. Daniel Rubin, also from Stanford, who discussed ongoing work at the university to address a number of challenges in healthcare, such as the variation in how physicians practice medicine. When confronted with the same patient or the same image, many physicians don't come up with the same conclusion. And AI can help with that.
"Physicians don't need to be replaced. They need help doing their jobs taking care of patients," Rubin said. "If we get to the day that robots can put hands on patients, then maybe we will be at a point that we have to worry about the careers of physicians, but for the time being physicians are the caregivers, not computers. But physicians are imperfect, and they need help."