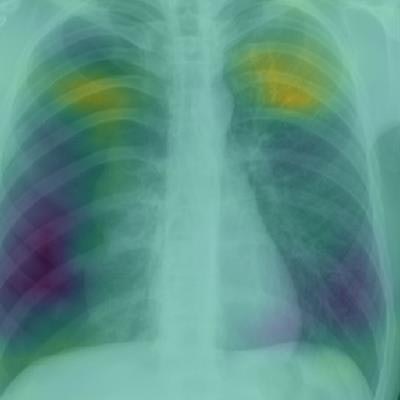
Artificial intelligence (AI) algorithms can automatically detect large pneumothoraces on chest x-ray exams -- potentially speeding up the detection and reporting of these critical findings, according to research presented at the RSNA 2017 meeting in Chicago.
After training and testing six different deep-learning networks, researchers from Thomas Jefferson University (TJU) in Philadelphia found that an ensemble approach blending results from the six models yielded the highest level of performance -- including 94% sensitivity -- for identifying large pneumothoraces.
"Deep convolutional neural networks show promise in the detection of large pneumothoraces," said presenter Dr. Paras Lakhani.
A critical finding
A large pneumothorax is considered a critical finding by most radiology departments. Although large pneumothoraces -- those that have more than 2 cm depth from the chest wall -- are fairly apparent to all clinicians and easy to spot on chest radiography, clinical demands may delay the reading of some of these x-rays until hours after they are obtained, according to Lakhani.
"Critical findings including large pneumothoraces may not be detected immediately," he said.
AI could be useful for this application, according to Lakhani.
"For example, such algorithms could process chest radiographs right after they are obtained, and the preliminary AI results could facilitate work prioritization on a reading worklist," he told AuntMinnie.com. "Radiologists could prioritize viewing studies flagged by the AI system as positive, which may allow for more rapid identification of such results."
The researchers sought to assess the efficacy of deep convolutional neural networks for differentiating large pneumothoraces from radiographic control cases that included normal findings, bullous disease, or resolved pneumothoraces. They used 644 deidentified frontal-view chest radiographs to train the algorithms. Of the 644 cases, 311 (48.3%) had a large pneumothorax and 333 (61.7%) served as controls -- including 119 cases of bullous disease (confirmed by CT or stability of findings on x-ray for six months), 102 cases of resolved pneumothorax, and 108 normal results. All findings were verified by two board-certified radiologists, Lakhani said.
Of the 644 images, 480 (74.5%) were used for training and 64 (10%) were used for validation. The remaining 100 (15.5%) were set aside for testing.
6 different models
Lakhani and colleagues evaluated six deep-learning models, including both untrained and pretrained versions of the AlexNet, GoogLeNet (Inception V1), and ResNet 34 architectures. The pretrained models were trained on the 1.2 million color images from the ImageNet database. The researchers elected to use a 34-layer ResNet model because "it's easier to train from scratch as opposed to deeper networks," he said.
All image data were resized to 256 x 256 pixels and were then augmented using quadrilateral rotations, translation, sharing, and contrast enhancement to increase the training dataset size by 48-fold. On-the-fly random cropping and horizontal flipping were also performed on the images.
The researchers trained the models on an Ubuntu Linux 14.04 computer powered by a Titan X Maxwell (Nvidia) graphics processing unit and 12 GB of RAM. They used the Caffe deep-learning framework (Nvidia) to run the AlexNet and Inception V1 models. The open-source Torch framework was used for ResNet 34.
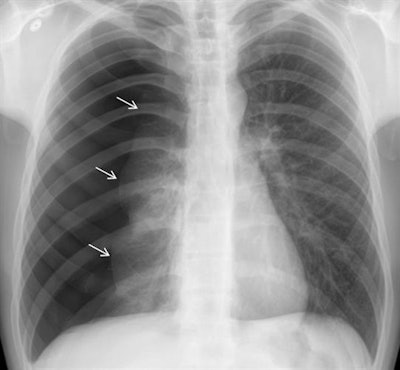
After testing all six models, the researchers found that combining the results of the six algorithms yielded the best performance.
Performance of deep-learning models in detecting large pneumothoraces | |||||||
Untrained | Pretrained | Ensemble approach (all 6 models) |
|||||
AlexNet | Inception V1 | ResNet 34 | AlexNet | Inception V1 | ResNet 34 | ||
Sensitivity | 90% | 86% | 74% | 92% | 94% | 80% | 94% |
Specificity | 84% | 80% | 88% | 88% | 78% | 88% | 88% |
AUC (95% CI) | 0.90 (0.84-0.97) | 0.90 (0.83-0.96) | 0.89 (0.83-0.95) | 0.94 (0.89-0.98) | 0.91 (0.85-0.97) | 0.90 (0.84-0.97) | 0.96 (0.92-1.00) |
The ensemble approach of blending all six models detected 47 (94%) of the 50 pneumothoraces in the testing dataset. There were six false-positive results, including two cases of bullous disease and four resolved pneumothoraces, Lakhani said.
"More training cases and other deep-learning strategies may improve these results," he said.