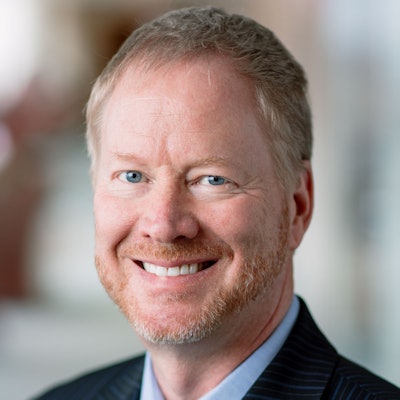
Is developing deep-learning algorithms for medical imaging applications an art or a science? It's much more of an art at the moment, according to an article from a team led by Dr. Bradley Erickson, PhD, of the Mayo Clinic in Rochester, MN, that was published online January 31 in the Journal of the American College of Radiology.
Although deep-learning algorithms are easier to train than traditional machine-learning methods, they require more data, and much more care is needed when analyzing results, according to the group. It's also not easy at this point to determine the optimal deep-learning architecture for solving a particular imaging problem.
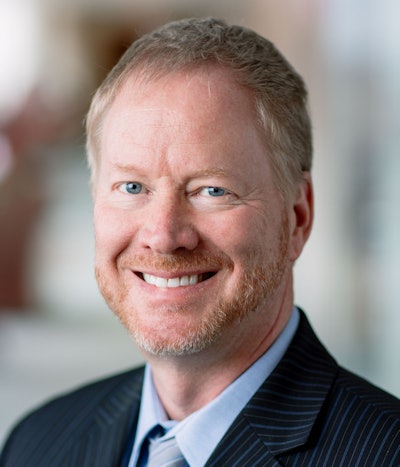
Fortunately, deep-learning theory continues to advance, yielding better learning techniques and enhanced understanding of the best architectures for certain classes of problems, according to the authors.
"[Deep learning] has dramatically improved the performance of computer algorithms outside of medicine, and it can be expected to dramatically improve performance of medical applications in the near future," they wrote.
In these early days of applying deep learning in medical imaging, though, the development of algorithms requires dealing with a number of challenges, including utilizing methods for increasing the size and variability of training datasets and settling on the best architecture for a convolutional neural network (CNN). There currently isn't a rule of thumb for selecting the appropriate CNN architecture to perform a given image classification task, according to the authors.
It can also be challenging to understand what image features the deep-learning applications are "seeing" and considering important during the analysis. However, there are ways -- such as adding and removing image features or displaying saliency or attention maps -- that can offer insight into how these networks operate, according to the researchers.
It's also likely that deep learning will uncover some unexpected new image features that can facilitate or enhance diagnosis, they noted.
"Although much radiology in the past has been hypothesis-driven, discovery science is needed to help us take the next major steps forward by identifying unexpected or otherwise unobserved findings in our data," the authors wrote. "Tools that can highlight these findings are essential to the vitality of our specialty. This is one reason why [deep learning] seems to be a critical tool for advancing radiology."