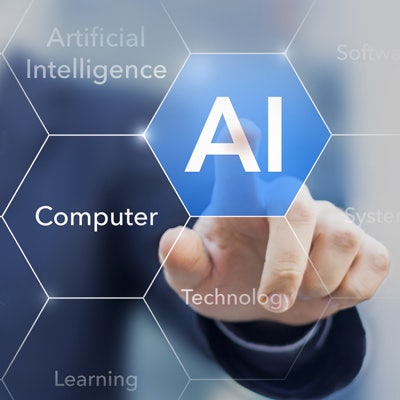
Artificial intelligence (AI) algorithms can identify follow-up recommendations included in radiology reports, potentially giving radiologists a tool to ensure their recommendations have been followed, according to research published online December 29 in the Journal of the American College of Radiology.
Researchers led by Dr. Emmanuel Carrodeguas of Harvard Medical School in Boston trained a variety of machine-learning and deep-learning models to identify follow-up recommendations in radiology reports. Although machine-learning models outperformed deep-learning algorithms in their study, both showed promising results and were considered to be feasible methods.
"Automatic identification of follow-up recommendations could have wide implications for establishing and timely performance of collaboratively developed follow-up care plans for actionable findings in radiology reports to improve quality and experience of care for patients," the authors wrote.
The researchers manually reviewed 1,000 randomly selected ultrasound, CT, and MRI reports produced in 2016 at their institution and annotated the reports for follow-up recommendations. Overall, 12.7% of the reports included follow-up recommendations.
Using 850 of these reports, the researchers trained deep-learning models -- recurrent neural networks -- as well as three types of traditional machine-learning algorithms (support vector machine, random forest, and logistic regression).
Next, Carrodeguas and colleagues assessed the performance of the algorithms for identifying follow-up recommendations on the remaining 150 reports by calculating their precision, recall, and F1 score -- a measure of a test's accuracy that considers both precision and recall. They also compared the algorithms' results with Information From Searching Content With an Ontology-Utilizing Toolkit (iSCOUT), a previously validated natural language processing (NLP) system.
Accuracy of AI tools for identifying follow-up recommendations | |||||
Deep-learning algorithm (recurrent neural networks) | iSCOUT NLP software | Random forest machine-learning algorithm | Logistic regression machine-learning algorithm | Support vector machine machine-learning algorithm | |
F1 score | 0.71 | 0.71 | 0.75 | 0.83 | 0.85 |
"It remains possible that with larger datasets and more complex architectures, [deep-learning] methods would provide better classification, as [deep learning] continues to break barriers in other fields, including pathology, cancer prognosis, and drug discovery," they wrote.
The researchers believe their findings open up the possibility of using automatically extracted follow-up recommendations from radiology reports in clinical practice.
"For reports containing further recommendations, a notification system may be developed that can monitor and inform providers so that necessary diagnostic follow-up is not delayed," they wrote. "In addition, identifying follow-up recommendations within one's own practice provides an opportunity to quantify between-provider variations in follow-up recommendations for specific findings, as well as variations resulting from clinical uncertainty."
Furthermore, an integrated system built on this work could be useful from a population health perspective, such as for validating radiologist recommendations with standards and providing real-time feedback when reports are generated, according to the researchers.
"Future work can leverage optimized models, expanding on current architectures with more computing power; focus on training and modifying models to extract further information regarding the details of recommendations, including the reason (why) and content (what) and test real-world generalizability," they wrote.