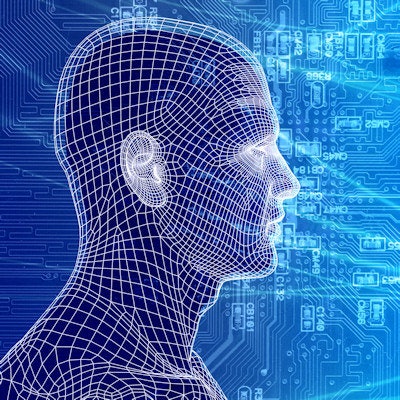
The current medical landscape for artificial intelligence (AI) allows for early adopters to embrace AI innovation with ease, yet it has left the majority of medical institutions struggling to catch up. Technical, regulatory, and financial barriers will continue to hinder the adoption of emerging AI technologies. Therefore, to facilitate the adoption of AI into the standard of care, important structural changes are needed.
For AI solutions to become an integral part of the standard of care, medical facilities, AI vendors, PACS and other IT suppliers, radiology leaders (e.g., the American College of Radiology), and governmental authorities (e.g., the U.S. Food and Drug Administration and U.S. Centers for Medicare and Medicaid Services) must work together and in parallel to establish an environment that fosters the incorporation of AI into standard medical practice.
In this context, diverse factors must be considered, including the introduction of reimbursement for AI-based solutions, FDA support, and the development of an IT ecosystem to support various AI solutions. All of these factors will play a crucial role in enabling the adoption of AI as part of the new standard of care.
Standard of care: A practical definition
The concept of "standard of care" derives from the definition of negligence in early American tort law. One widely accepted definition comes from the American Law Institute: "the standard of conduct to which one must conform ... [and] is that of a reasonable [person] under like circumstances."
When applied to medicine, this standard of conduct is established by explicit or implicit consensus within the medical community. As Moffett and Moore formulate it, "if others in the business are commonly practicing a certain way that eliminates hazards, then this practice can be used to define the standard of care."
We do not intend to equate AI with the fundamental elements of clinical medicine that we consider standard, such as vital signs and a physical exam, nor do we wish to make any predictions of AI's role in the future medicolegal landscape.
However, we wish to propose the somewhat bold notion that, in time, AI will eventually become so indispensable to eliminating hazards in our data-overloaded healthcare environment that practicing medicine in its absence may be tantamount to nonstandard care. And on a societal level, if the various stakeholders in the development of medical AI fail to act promptly and in concert, we risk allowing a gap to develop between AI adopters and nonadopters, which could lead to major disparities in patient outcomes.
Getting paid: ROI, insurance codes, and value-based reimbursement
Financial motivation will clearly play an important role in the adoption of AI. Accordingly, the AI community will need to develop deployment models that clearly demonstrate the financial benefits to all parties concerned. One can devise several such models, but which one of them will win the day?
D. Kurt Schoppe, chair of the American College of Radiology's reimbursement committee, explored the question of AI financial models in a recent commentary published in the Journal of the American College of Radiology. Schoppe highlighted the barriers AI vendors will face when attempting to receive reimbursement, and he concluded that investing in AI may become a simple cost of doing business like any other operational expense.
If that's the case, the burden rests on AI vendors to produce evidence-based return-on-investment (ROI) models through which hospitals and radiology departments can recognize the value of AI. Examples of such potential value propositions could include avoiding lost revenue, increasing efficiencies (either sheer relative value unit volume or deeper workflow efficiencies), or reducing overall costs (e.g., reducing length of stay).
Another approach would be to have current procedural terminology (CPT) codes granted directly to AI-based solutions. This would probably require even more clinical evidence on AI's overall benefits but could eventually lead to widespread adoption.
Moreover, CPT codes may be essential to motivate healthcare systems to optimize patient outcomes (as opposed to financial savings only). But CPT codes for AI seem a remote goal presently; it seems more likely that Medicare-based reimbursement will first occur through value-based reimbursement.
This presents itself as a great opportunity, as AI has the potential not only to improve known value-based metrics but also, in some settings, to provide a window into key value metrics that were previously unappreciated. In other words, AI is ideally positioned to improve key metrics that could then be measured and paid on in a value-based payment system.
One early example of AI's contribution to value-based care is the ability to measure, at a granular level, factors influencing turnaround time for positive intracranial hemorrhage cases. By utilizing a registry such as SaferMD, a radiology practice can not only improve this outcome but also get a payment based on the practice's improved performance. This factor is known to be important, but its measurement has been elusive until recently.
FDA support
The U.S. Food and Drug Administration (FDA) has recently made great strides in easing the clearance process for AI solutions to enter the market more quickly. FDA Commissioner Scott Gottlieb has made a variety of supportive statements; for example, declaring that the FDA is reconsidering its approach to regulating AI-powered software and devices. In fact, in 2018 alone, the FDA accelerated its approval process with more than 10 medical AI clearances, including Arterys for oncology, Aidoc for triaging intracranial hemorrhage (ICH), and Zebra Medical Vision for calcium scoring.
There are two new challenges that AI-based solutions pose. First, the scale and velocity in which new algorithms are being developed is staggering. It is now possible to submit five products to the FDA at the same time, so a streamlined regulatory framework needs to be adopted.
Second, AI solutions have the capacity to continuously evolve and improve. Patients could benefit greatly if the FDA would provide a pathway to continuously upgrade solutions on the fly, based on the best available data.
New FDA programs like precertification hold significant promise in matching the high speed of product development in the AI world. This program puts an emphasis on postmarket surveillance, thus reducing the time to market significantly.
For AI to become a truly integral part of the standard of care, the FDA will need to continue in this vein, adopting more flexible regulations in a world where AI solutions are emerging at an extraordinary pace. These recent changes are precisely the encouragement the AI community needs to continue innovating rapidly in a diverse array of applications.
Developing an IT ecosystem
An IT ecosystem that supports the simultaneous deployment of various AI solutions will become essential as more AI solutions reach the market. The challenge is to develop an infrastructure in which all AI companies can work together.
Each AI solution must become agnostic enough to allow for the creation of systems combining different AI modules. Within such an environment, PACS/electronic health record (EHR) vendors and hospital IT infrastructures will be able to adopt and implement AI solutions with greater ease.
Currently, hospitals are faced with a complicated web of integration problems. Sometimes, the introduction of an AI-based solution necessitates cumbersome changes in the existing workflow. If AI developers could produce one standard set of interfaces for the deployment of all AI solutions, which the other workflow vendors (e.g., PACS, reporting) could adopt easily, this would ensure better integration, more reliable performance, and ease of access for all. In addition, IT ecosystems should strive to allow the evaluation of interdependencies where one AI subsystem counteracts another.
Institutions are also seeing the need to provide structural support in developing a sustainable platform for integrating AI. At the Society for Imaging Informatics in Medicine (SIIM) 2018 annual meeting, the American College of Radiology Data Science Institute (ACR DSI) held a summit dedicated solely to moving AI algorithms into routine clinical practice. This important discussion is ongoing.
Evidence
To truly become part of the standard of care in an evidence-based medical world, AI will have to supply robust evidence to support its value.
The bare minimum is, of course, in proving the accuracy of the system. This evidence exists and the findings are encouraging, but accuracy is an insufficient metric. The coming challenge in the AI community is to provide evidence regarding the impact on actual clinical outcomes.
For example, we need to begin evaluating whether AI systems can significantly affect diagnostic turnaround times, emergency room (ER) callbacks, ER/inpatient stays, and so on. On the clinical level, an important consideration will be whether AI can increase sensitivity without a significantly negative effect on specificity and overdiagnosis.
The next phase would be a demonstration of the ROI benefits of AI adoption. We foresee a process similar to the ROI research that paved the way for PACS adoption. Going forward, perhaps we should also pursue some form of long-term prospective monitoring of AI's benefits, as has been done with other medical technologies. This would confirm earlier findings and determine venues for further improvement. The first companies that can publish such conclusive research will probably be the first ones to reap the benefits of their solutions' adoption as a standard of care.
A new standard of care
The challenge of integrating AI into the standard-of-care framework is not an easy one -- but with medical facilities, AI developers, PACS and EHR vendors, radiology leaders, and governmental authorities working together, very soon patients and medical centers across the healthcare spectrum will be able to benefit from the extraordinary clinical benefits that AI has to offer.
Elad Walach is the co-founder and CEO of Aidoc, a healthcare AI start-up focused on using deep learning to relieve the bottleneck in medical image diagnosis. Walach began his career in the elite Israeli Defense Forces' Talpiot technology program. He served as a researcher in the Israeli Air Force's algorithmic division, where he raised through the ranks, reaching the position of algorithmic research leader. He led several teams focused on machine-learning and computer-vision projects from inception to execution. Walach holds a Bachelor of Science in mathematics and physics from the Hebrew University of Jerusalem and a Master of Science in computer science with a focus on deep learning from Tel Aviv University.
Dr. Carl Aschkenasi graduated from Harvard Medical School, where he was honored with a Howard Hughes research fellowship. He completed his radiology residency at the Mallinckrodt Institute of Radiology in St. Louis. As a radiologist certified by the American Board of Radiology, he worked for two years performing general radiology in a hospital-based private practice in St. Louis, before moving to Israel in 2010 with his family. In Israel, Aschkenasi has been principally employed as a senior consultant radiologist at Teleradiology Solutions, which provides remote radiology services to numerous hospitals in 18 states and to underserved medical centers around the world. He has worked as a consultant for Aidoc for the past two years, and he believes AI applications in radiology will translate into more efficient and safer patient care.
The comments and observations expressed are those of the authors and do not necessarily reflect the opinions of AuntMinnie.com.