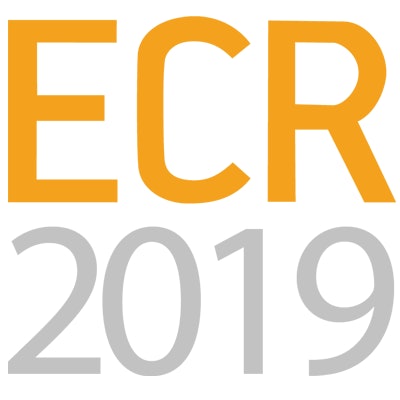
VIENNA - An artificial intelligence (AI) algorithm shows promise for helping radiologists predict progression-free survival in patients with glioblastoma based on the analysis of postoperative MRI scans, according to a presentation on Friday at ECR 2019.
Researchers from the University of Cologne in Germany and Philips Healthcare sister company Philips Research found that automated quantification of contrast-enhanced residual tumor volume by their deep-learning model was a significant predictor of progression-free survival.
"This has the potential to assist the radiologist," said presenter Dr. Michael Perkuhn.
Glioblastoma survival marker
Cytoreductive surgery is one of the cornerstones of glioblastoma therapy, and contrast-enhancing residual tumor volume on MRI has been discussed as a marker for predicting both overall survival and progression-free survival, Perkuhn said.
"The challenge with residual tumor volume is that it's very small [on postoperative MRI]; in our study, the overall volume was 1 mL," he said. "And it's also pretty heterogeneous, so this leads to challenges and there's a huge interrater variability between the readers. Having an automatic tool to derive the volumes could enhance this [analysis]."
As a result, the researchers sought to compare survival predictions using contrast-enhancing residual tumor volume obtained from an automatic deep-learning method as well as human readers. They retrospectively gathered a cohort of 80 patients out of 112 postoperative glioblastoma patients who had received postoperative MRI studies. These patients had an average age of 60 and included 55 men and 25 women. They had an average Karnofsky performance status (KPS) of 85 ± 14, with a median overall survival of 16.2 months (range, 0.5-63.3 months) and a median progression-free survival of 8.9 months (range, 2.2-46.9 months).
Postoperative MRI was performed on these patients within 48 hours after surgery and included T1, T2, fluid-attenuated inversion-recovery (FLAIR), and contrast-enhanced T1 sequences. All images underwent preprocessing, coregistration, skull stripping, resampling to isotropic resolution, and normalization, according to Perkuhn.
Deep learning
The researchers then trained a multiparametric deep-learning model to perform automatic segmentation of tumor compartments. They used DeepMedic brain lesion segmentation software architecture to develop the postoperative glioblastoma model. Developed by the Biomedical Image Analysis Group at the Imperial College London, DeepMedic consists of a deep 3D convolutional neural network, a 3D fully connected network, and a classification layer. The German researchers trained their model using both preoperative and postoperative data.
Automatic segmentation results for the contrast-enhanced residual tumor volume were then compared with manual segmentation using Dice similarity coefficient and Pearson volume correlations. The survival predictive strength of contrast-enhanced residual tumor volume was obtained automatically and manually and was assessed using Cox proportional hazard modeling.
The ground tumor compartments were delineated manually by a radiologist and a senior neuroradiologist in consensus using the IntelliSpace Discovery semiautomated software (Philips). The researchers then compared the segmented contrast-enhanced residual tumor volumes with the volumes that were segmented independently by neurosurgeons using iPlan software (Brainlab).
The deep-learning model produced an average contrast-enhancing tumor volume of 0.7 ± 1.4 mL, compared with an average of 1.1 ± 1.9 mL in manually calculated volumes. The Pearson correlation was 0.72, with a Dice similarity coefficient of only 0.33 ± 0.26.
"But we saw that the machine-learning approach [calculated residual tumor volume] in a very consistent fashion," Perkuhn said.
Survival predictors
After performing multiparametric Cox proportional hazard modeling and using age and KPS as additional covariates, the researchers found that only contrast-enhanced residual tumor volumes were significant predictors of progression-free survival. However, only patient age was a significant predictor of overall patient survival.
The hazard ratio calculated from the deep-learning model was slightly better than the overall hazard ratio for predicting progression-free survival, according to the researchers.
Hazard ratio for predicting progression-free survival in glioblastoma patients | |||
Based on neurosurgeon quantification of contrast-enhanced residual tumor volume | Based on radiologist quantification of contrast-enhanced residual tumor volume | Based on automatic quantification of contrast-enhanced residual tumor volume by deep-learning model | |
Hazard ratio (1 mL increment) | 1.11 | 1.13 | 1.21 |
p-value | < 0.02 | < 0.02 | < 0.001 |
The researchers then used Kaplan-Meier survival curves for progression-free survival based on the automatic quantitative analysis. Contrast-enhanced residual tumor volumes were categorized into one of three groups: no residual tumor, minimal residual tumor (less than 1 mL), and gross residual tumor (more than 1 mL). The subjects in the group with a residual tumor volume of more than 1 mL had a lower probability of progression-free survival and a shorter median progression-free survival time -- 6.8 months.
Perkuhn noted that other covariates such as additional treatment modalities and performance scores need to be included in their method for predicting overall survival and progression-free survival. Automatically derived volume-based tumor markers should also be investigated further, he said. In addition, the researchers aim to include more patients in the study.