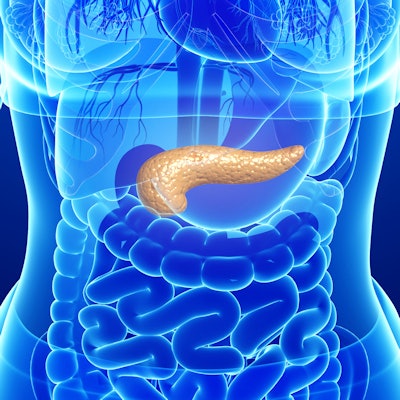
Radiomics and a machine-learning algorithm can differentiate pancreatic ductal adenocarcinoma (PDAC) from a normal pancreas on CT studies, potentially enabling earlier diagnosis of this highly lethal cancer, according to research published online April 23 in the American Journal of Roentgenology.
In a preliminary study, researchers from Johns Hopkins University, led by Dr. Linda Chu and Dr. Seyoun Park, found that a machine learning-based analysis of CT radiomics features extracted from the entire pancreas could be highly accurate for differentiating PDAC cases from normal control cases.
"There is the potential to combine this algorithm with automatic organ segmentation algorithms for automatic detection of PDAC," the authors wrote.
The 12th most common cancer and fourth most common cause of cancer deaths in the U.S., pancreatic ductal adenocarcinoma has an overall five-year survival rate of only 8%. Unfortunately, only up to 20% of patients present with localized disease -- when PDAC has the highest five-year survival rate at 32%. Although CT has been shown in the literature to be highly sensitive for PDAC, early cancer can be subtle and missed on CT by even experienced radiologists, according to the authors.
Radiomics, or quantitative imaging, features have been used previously to predict survival in patients with PDAC and to differentiate precursor lesions to the condition. In their study, the Johns Hopkins researchers sought to determine if CT radiomics features extracted from the entire pancreas could be utilized to differentiate PDAC from normal pancreatic tissue.
The researchers retrospectively gathered CT scans from 190 patients with PDAC and 190 healthy potential renal donors without known pancreatic disease from 2012 to 2017. The average size of the tumor was 4.1 cm ± 1.7. After four trained researchers manually segmented the 3D volume of the pancreas, 478 radiomics features were extracted to express the phenotype of the pancreas. Of these, 40 were selected for analysis by a machine-learning (random forest) classifier.
Of the 380 total cases, 255 (130 PDAC and 125 normal cases) were used for training the random forest classifier to provide a binary classification of PDAC versus normal. The remaining 65 normal control cases and 60 PDAC cases were used to validate the algorithm.
Performance of machine-learning algorithm for differentiating PDAC from normal cases | |
Machine-learning algorithm | |
Sensitivity | 100% |
Specificity | 98.5% |
Accuracy | 99.2% |
In the one false-positive case, an artifact related to the cholecystectomy clip may have contributed to alterations of the radiomics features, according to the researchers.
"Although the mean tumor size in the current study was larger than those that are easily missed on imaging in practice and the need for manual segmentation was labor intensive, this study provided evidence that radiomics is a feasible approach in tumor detection," the authors wrote. "Future work will evaluate the performance of radiomics in the detection of smaller tumors that may be more easily missed on imaging."
In addition, the radiomics algorithm will need to be validated across different institutions, vendors, and scanning protocols, the team noted.