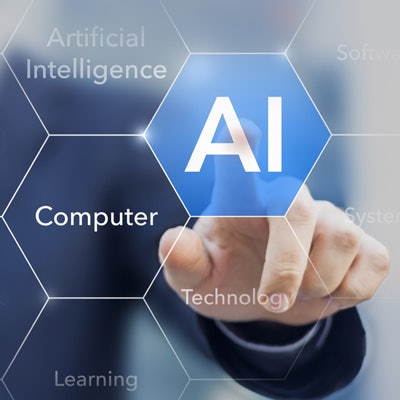
Bringing radiology artificial intelligence (AI) technology to routine clinical practice will require four major priorities: structured use cases, data sharing methods, validation and monitoring tools, and new standards and data elements, according to a report published online May 28 in the Journal of the American College of Radiology.
"An active AI ecosystem in which radiologists, their professional societies, researchers, developers, and government regulatory bodies can collaborate, contribute, and promote AI in clinical practice will be key to translating foundational AI research to clinical practice," wrote a team of authors led by Dr. Bibb Allen Jr. of the American College of Radiology (ACR) Data Science Institute.
Following up on an initial medical imaging artificial intelligence roadmap published April 16 in Radiology, which covered the challenges, opportunities, and priorities for foundational research in AI for medical imaging, Allen and colleagues turned their attention to the key priorities for translational research. Both articles were produced as a summary of last year's U.S. National Institute of Biomedical Imaging and Bioengineering (NIBIB) workshop on medical imaging, which was co-sponsored by the RSNA, the ACR, and the Academy for Radiology & Biomedical Imaging Research.
In their latest report, the authors highlighted four key translational research priorities:
- Create structured use cases to define and highlight the clinical challenges that AI could potentially solve.
- Create methods to encourage data sharing to support the training and testing of AI algorithms. This would promote generalizability of these algorithms to widespread clinical practice and mitigate unintended bias.
- Establish tools for validating and monitoring the performance of AI algorithms in clinical practice, to facilitate regulatory approval.
- Develop standards and common data elements to facilitate seamless integration of AI tools into existing clinical workflows.
In defining and prioritizing AI use cases, the medical imaging community should describe exactly what's important to radiology and what data scientists -- including researchers and developers -- can do to improve patient care, according to the authors.
"Those descriptions should go beyond narratives and flowcharts," they wrote. "Human language should be converted to machine-readable language using standardized data elements with specific instructions for standard inputs, relevant clinical guidelines that should be applied, and standard outputs so that inferences can be ingested by downstream HIT resources."
Standardized inputs would enable algorithms to run on the modality, on a local server, or in the cloud. Meanwhile, application programming interfaces (APIs) could be developed based on these standardized outputs to integrate AI into any system or electronic resource, according to the researchers.
Furthermore, structured use cases should include specifications for data that should be collected to inform the developer how the algorithm performs in actual clinical use, according to the researchers.
"Understanding performance variances that occur in different patient populations, across different equipment manufacturers, or using different acquisition protocols can then be used to refine the algorithm, modify the use case specifications, or inform regulatory agencies," they wrote.