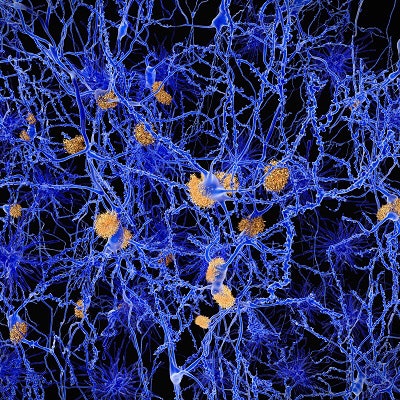
An artificial intelligence (AI) model that simulates a clinician's diagnostic process by analyzing both neuroimaging results and clinical cognitive impairment test scores can yield excellent performance for diagnosing Alzheimer's disease, according to research published online July 16 in Neurocomputing.
A team of researchers led by Fan Zhang of Henan University in China developed a model that first utilizes two individual deep-learning algorithms for evaluating brain PET and MRI studies. A final prediction on whether a patient is cognitively normal or has mild cognitive impairment (MCI) or Alzheimer's disease is then generated after also considering results from cognitive impairment tests.
In testing, the model produced over 97% sensitivity and 88% accuracy for distinguishing between cognitively normal subjects and Alzheimer's disease patients.
"A large number of experiments on the open database of [the Alzheimer's Disease Neuroimaging Initiative] ADNI show that the diagnostic effect of the proposed method is superior to other auxiliary diagnostic models in many indicators," the authors concluded. "The experimental results show that the proposed multimodal auxiliary diagnosis can achieve an excellent diagnostic efficiency."
The Chinese group sought to incorporate analysis of clinical neuropsychological diagnosis to improve on the promising results reported in the literature for applying deep-learning multimodality neuroimaging analysis algorithms to diagnose MCI and Alzheimer's disease.
To train and test their deep-learning algorithms, the researchers used data from 392 subjects from the baseline ADNI database. Of these, 200 had mild cognitive impairment (MCI), 101 were cognitively normal, and 91 had Alzheimer's disease. All subjects included in the trial had received both PET and MRI. The researchers used 90% of the dataset for training, 5% for verification, and 5% for testing.
First, two independent convolutional neural networks (CNNs) extracted features from MRI and PET images to arrive at a separate diagnosis for each modality. The researchers then used Pearson correlation analysis to determine if the diagnostic results were consistent for each modality.
"If the results diagnosed by the two CNN models are similar, it is intuitive that the diagnosis by different modality is consistent for the same patient," they wrote. "Based on this idea, we calculate the correlation between the diagnosis result of PET images and the diagnosis result of MRI images as the weight of the multimodal neuroimaging diagnosis."
This neuroimaging diagnosis is then integrated with Mini-Mental State Examination (MMSE) and Clinical Dementia Rating (CDR) scores to produce a comprehensive patient classification prediction.
Performance of deep-learning model for Alzheimer's disease | |||
Distinguishing between cognitively normal subjects and Alzheimer's disease patients | Distinguishing between cognitively normal subjects and patients with MCI | Distinguishing between MCI and Alzheimer's disease | |
Sensitivity | 96.6% | 90.1% | 97.4% |
Specificity | 95.4% | 91.8% | 84.3% |
Accuracy | 98.5% | 85.7% | 88.2% |
Area under the curve | 0.986 | 0.882 | 0.88 |
"The advantage of the proposed model is that it combines the neuroimaging diagnosis with the clinical neuropsychological diagnosis," the authors wrote. "The diagnosis process is closer to the process of clinician's diagnosis and easy to implement."