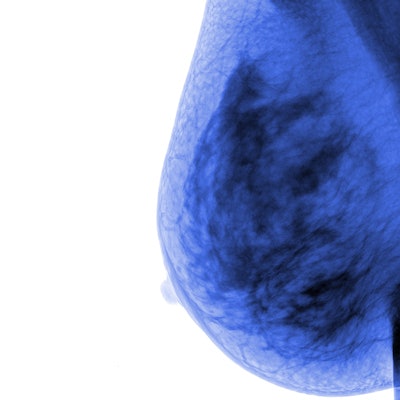
Artificial intelligence (AI) may create new opportunities to improve outcomes for breast cancer patients via treatments that can be adapted based on patient response, as well as targeted therapies based on radiomic and pathomic analysis, according to an article published online August 22 in the Journal of Medical Imaging and Radiation Sciences.
In a commentary article, a multinational group of authors, led by William Tran, PhD, of Sunnybrook Health Sciences Centre in Toronto, discussed AI's potential for detecting breast cancer and for predicting treatment response and the risk of cancer-related mortality.
"AI will be critical for identifying candidate biomarkers from digital imaging and developing robust and reliable predictive models," the authors wrote. "These models will be used to personalize oncologic treatment strategies, and identify confounding variables that are related to the complex biology of tumors and diversity of patient-related factors (i.e., mining 'big data')," they wrote.
The opportunities for AI in oncology are immense, according to Tran and colleagues.
"Machine-learning and deep-learning applications are demonstrating promising new assays for predicting treatment response and survival outcomes for high-risk breast cancer," they wrote.
In particular, the authors highlighted the potential for AI to personalize neoadjuvant chemotherapy for breast cancer and to enable radiotherapy dose de-escalation in early-stage, low-risk breast cancer. They also pointed to the potential for AI to combine and exploit "multi-omics" data, or information from multiple disciplines such as radiomics, genomics, pathomics, transcriptomics, proteomics, and metabolomics.
"Each of these branches [holds] great promise for understanding cancer's aberrant life cycle; however, together may provide a more comprehensive, multilevel portrait of the tumor's biology," they wrote. "Multi-omics data may also provide better models to [predict] treatment response."
Challenges do remain, however, in the areas of data provenance, optimization, and tuning parameters for machine- and deep-learning algorithms. Other thorny issues include the need to standardize imaging acquisition and its parameters and to align ground-truth labels with well-defined clinical end points, Tran and colleagues explained.
The authors also acknowledged current efforts to utilize less-expensive imaging modalities -- such as quantitative ultrasound -- to make radiomics more affordable and accessible. Work is underway to validate quantitative ultrasound radiomics in the long term, particularly in breast cancer.
"Novel [quantitative ultrasound] imaging techniques to attain imaging biomarkers aim for objective, reproducible [data], and have the high potential for AI-driven automation and interpretation," they wrote. "These attributes can be useful for low- and middle-income countries that have limited capital and human resources."
Despite these lingering issues, the authors believe that AI is poised to play an important role in managing the diagnosis, treatment, and follow-up of breast cancer.
"Developing such systems will lead to increasing presence of digital imaging and electronic medical information systems in oncology clinics," they wrote. "The shift to these digital platforms will allow immense datasets to be created and mined to extract new information that can be used for clinical decision-making."
Tran's group expects that AI will become commonplace in oncology as the technology evolves, offering significant potential to produce data-driven strategies for diagnosis and treatment.