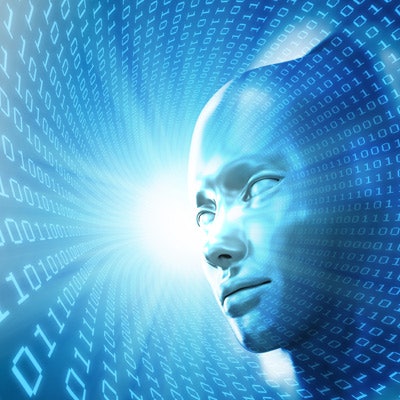
It's not enough for a healthcare artificial intelligence (AI) algorithm to be highly accurate. To be widely adopted in clinical use, it must demonstrate improvement in quality of care and patient outcomes, according to an opinion article published online October 29 in BMC Medicine.
A team from Google Health in London, U.K., led by Dr. Christopher Kelly, PhD, said that further work is needed to develop tools to address bias and unfairness in algorithms, reduce the brittleness of AI and improve the generalizability of models, and develop methods for improving the interpretability of machine-learning predictions.
"If these goals can be achieved, the benefits for patients are likely to be transformational," the group wrote.
AI faces a number of challenges standing in the way of translation into clinical practice, including those intrinsic to the science of machine learning, logistical difficulties in implementation, and barriers to adoption, as well as sociocultural or pathway changes associated with using the technology, according to the team.
Although performing peer-reviewed clinical evaluation as part of randomized clinical trials should be viewed as the gold standard for generating evidence, it may not always be appropriate or feasible to conduct these assessments in practice, Kelly and colleagues noted.
"Future studies should aim to use clinical outcomes as trial endpoints to demonstrate longer-term benefit, while recognizing that algorithms are likely to result in changes of the sociocultural context or care pathways; this may necessitate more sophisticated approaches to evaluation," they wrote.
Furthermore, performance metrics should aim to capture the real clinical applicability of AI and be understandable to the intended users of the algorithms, according to the group.
Kelly and his team noted that regulation -- including thoughtful postmarket surveillance -- is needed to balance the pace of innovation in artificial intelligence with the technology's potential for harm. Also, mechanisms to enable direct comparisons of AI systems must be developed, including utilizing independent, local, and representative test sets.
"Developers of AI algorithms must be vigilant to potential dangers, including dataset shift, accidental fitting of confounders, unintended discriminatory bias, the challenges of generalization to new populations, and the unintended negative consequences of new algorithms on health outcomes," they wrote.
In addition to the technical challenges that must be overcome, there are also human barriers to the adoption of AI.
"To ensure that this technology can reach and benefit patients, it will be important to maintain a focus on clinical applicability and patient outcomes, advance methods for algorithmic interpretability, and achieve a better understanding of human-computer interactions," the group wrote.
So-called "explainable" AI methods are likely to be adopted faster in clinical practice and will help foster transparency and trust with their users, according to the team.
"Further work to improve the interpretability of algorithms and to understand human-algorithm interactions will be essential to their future adoption and safety supported by the development of thoughtful regulatory frameworks," they wrote.