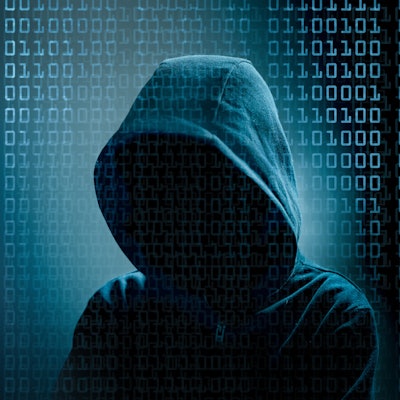
Artificial intelligence (AI) and machine learning are currently experiencing a renaissance in radiology. However, little to no research effort has focused on the security aspects of applying AI to the image data of our patients. Although machine learning can be tremendously helpful in the detection and prevention of attacks, it could also be used as a weapon by hackers or cyberterrorists.
In a study presented at the 2018 RSNA conference and published recently in the European Journal of Radiology, we explored the potential of a subclass of deep learning called generative adversarial networks (GANs) to be used for sinister injection or removal of breast cancer in mammograms. A study presented less than a year after our proof-of-concept at the 28th USENIX Security Symposium demonstrated that this scenario is already beyond theoretical deliberations, at least in a more handcrafted pipeline for low-resolution patches of CT images.
However, in any attack scenario explored above, other weaknesses in the system need to be exploited in order to gain inside access to the system -- for example, understaffed hospital security at night enabling physical access to the scanner room.
Trojan horse
The classical corporate IT security system is modeled after a fortress, i.e. firewalls control the network activities and block most communication to the outside, while the internal IT network is considered "safe" and only loosely controlled. However, this approach is only effective against attacks as long as the attack actually originates from the outside world. As soon as an attacker manages to (e.g., physically) pass this security perimeter -- analogous to the tale of the Trojan horse -- the fortress's walls become useless.
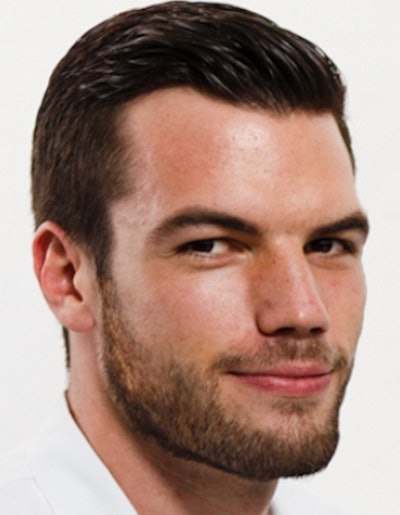
Today's advanced persistent threat (APT) attacks employ these very tactics to first infect a regular computer within an organization's network and subsequently use this computer to probe the company's network, steal information, and manipulate data. On average, more than 200 days pass until the security breach is noticed and the organization realizes that attackers are working from inside their network, according to a report analysis published online August 14 in Computer Fraud & Security.
Machine learning is becoming increasingly useful for providing faster and better detection of such attacks. Akin to finding the few cancerous lesions in a large, otherwise healthy screening mammography population, the challenge is to identify the few malicious activities amongst the billions of "healthy" data packets sent over the network on a typical business day.
This may well be the beginning of an arms race: Who has the better algorithms, the attacker or the defender?
Granted, using AI or GANs for criminal image manipulation has a certain dramaturgic appeal in this day and age. And while we welcome the new subfield of research that seems to be developing, it should be kept in mind that the problems enabling such attacks in the first place are of a much more basic nature.
A recent report by ProPublica and Bayerischer Rundfunk found that data from millions of patients was compromised by carelessly leaving PACS interfaces open to the web. One does not need any AI to exploit such a yawning gap. Hence, the most logical first step to prevent any AI- or non-AI-mediated cyberattacks is to conduct a sound security audit within the department and/or hospital and close any obvious leaks.
Sufficient firewalls
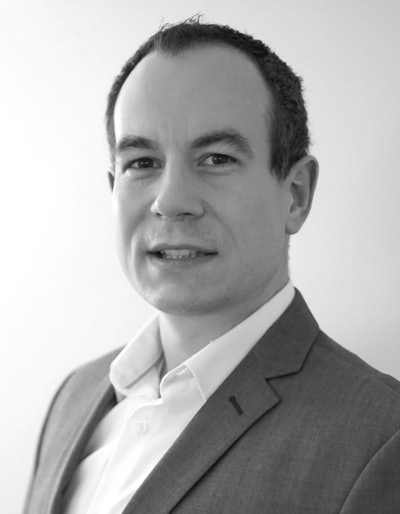
Devices and servers that are only used inside the hospital (which will be the vast majority) should not be accessible from the outside. The firewalls should be "high" enough to effectively block attacks and deter attempts to steal data but not so high that they would impair usability and effective communication between departments and/or hospitals.
Next, it is crucial to understand what is already on the inside. Are all devices registered? Are all the latest patches and updates applied? Which other devices does a certain device need to contact, and what does its normal traffic look like? This may get increasingly challenging as more and more medical imaging devices come equipped with embedded AI algorithms. Transparency on the side of the vendors will be key here, since one cannot secure what one does not understand.
Unfortunately, information security in healthcare is chronically underfunded, with the majority allocating less than 3% to 6% of the IT budget, compared with at least 15% in other industries (e.g. the banking sector). The reasons for this may be manifold, but nowadays patient care -- and in particular medical imaging -- is entirely dependent on digital workflows. Hence, we owe it to our patients to invest more resources in guaranteeing the safety and integrity of their data in our workflows -- from image generation all the way to clinical management.
Dr. Anton Becker, PhD, is a radiologist at Memorial Sloan Kettering Cancer Center in New York City. He trained in Switzerland at the University Hospital Zürich (MD) at the institute of diagnostic and interventional radiology led by Professor Jürg Hodler and at ETH Zürich (PhD) in the group of Professor Christian Wolfrum. His research interests are oncologic imaging and the application of machine learning in radiology.
David Gugelmann, PhD, is a security analytics researcher and CEO of the ETH spinoff Exeon Analytics. Prior to founding Exeon Analytics in 2016, he was a postdoctoral researcher at ETH Zurich in the Networked Systems Group. His research interests are in big data analytics, digital forensics, and machine learning for anomaly detection. He combines these areas by developing big-data security analytics solutions to fight advanced cyberattacks.
The comments and observations expressed are those of the authors and do not necessarily reflect the opinions of AuntMinnie.com.