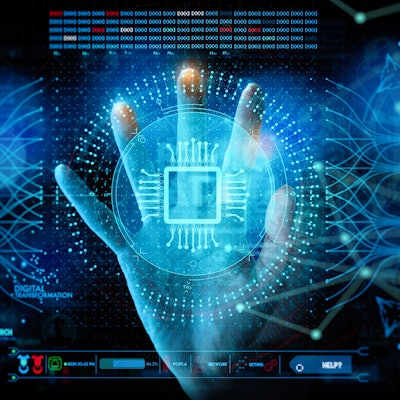
The use of artificial intelligence (AI) in radiology to aid in image interpretation tasks is evolving, but many of the old factors and concepts from the computer-aided detection (CAD) era still remain, according to a Sunday talk at the Conference on Machine Intelligence in Medical Imaging (C-MIMI).
A lot has changed as the new era of AI has emerged, such as faster computers, larger image datasets, and more advanced algorithms -- including deep learning. Another thing that's changed is the realization of additional reasons and means to incorporate AI into clinical practice, according to Maryellen Giger, PhD, of the University of Chicago. What's more, AI is also being developed for a broader range of clinical questions, more imaging modalities, and more diseases, she said.
At the same time, many of the issues are the same as those faced in the era of CAD. There are the same clinical tasks of detection, diagnosis, and response assessment, as well as the same concern of "garbage in, garbage out," she said. What's more, there's the same potential for off-label use of the software, and the same methods for statistical evaluations.
There's also the same need for a sufficient number of training cases that represent the diseases an AI algorithm might encounter, as well as the same need to team experts in the imaging domain with those of the computer realm, she said.
Giger discussed the changing role of AI in medical imaging interpretation in the opening keynote presentation at C-MIMI 2020, which is being held by the Society for Imaging Informatics in Medicine (SIIM).
Changing motivations
There are notable shifts between the CAD era and today's emerging AI epoch, however. In applying AI for breast cancer screening, for example, the focus in the past was on utilizing the technology as a second reader. But that's changed to a concurrent-reading approach, according to Giger.
There's also been a change in the motivation behind the use of these technologies.
"Initially, it was to improve the performance of radiologists and have them reduce the misses in their detection of [for example,] breast cancer," she said. "Now the motivation is improving efficiency, especially as we go into 3D imaging. We have more data for the radiologist to handle. ... And could we have it so that the radiologist performs just the way they were doing before, but in half of the time."
For example, various methods are being developed for whole-breast ultrasound to expedite finding a lesion in the 3D dataset, she said. Other notable areas in applying AI in breast cancer screening, diagnosis, and assessment include computer-assisted triage, developments in computer-assisted diagnosis such as assessing intratumor heterogeneity, and radiogenomics.
Explainable AI
"Explainable" AI, and the task of communicating AI to end users, have been hot topics in radiology. If radiologists don't find the interface to be user-friendly, they won't use the AI, Giger said.
But would radiologists trust AI more if the computer output could be explained?
"If you have an AI output that reaches a point where it's just so good, [explainable AI] may not be necessary," she said.
Also, radiologists have used "black boxes" before, such as CT technology, Giger noted.
"Radiologists understand in general how the CT scanner works, but unless you're an engineer working on that CT system itself, you don't know all of the different parameters," she said. "You know it gives a good image, so you trust it. So I think it's a tradeoff between performance and understanding [the AI]."
There's also a need to be careful in assessing heat maps. She discussed some cases where the AI algorithm reported a pneumothorax, but the heat map on the images focused on the wrong areas.
"So we have to relate performance to actually go back and see where the visualization is," she said.
Giger added that it's also very important to establish highly curated datasets for training AI. These datasets should be open and available to as many researchers as possible, she said.
Concluding thoughts
Giger concluded by noting that the use of AI in medical imaging has been around for decades, and it's important to learn from the past. In addition, the ultimate AI systems will contain combinations of human-engineered (i.e. traditional machine-learning techniques) and multiple deep-learning methods, she said.
"Don't forget about human-engineered [AI]," she said. "Keep looking at both [human-engineered and deep learning]."
She also emphasized that AI should ultimately be evaluated on how it impacts the performance of the end user or consumer of the AI, rather than just focusing on the algorithm's standalone performance statistics.
"We can't forget that," she said. "We have to always keep the big picture in mind."