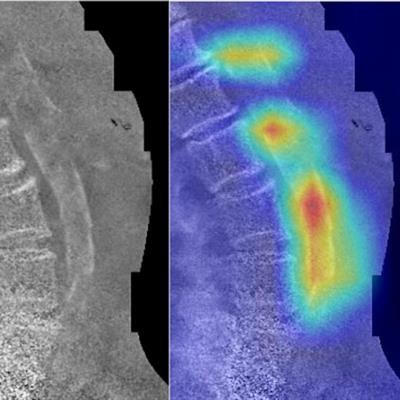
A deep-learning algorithm designed to read dual-energy x-ray absorptiometry (DEXA) images may also help to predict cardiovascular disease, according to a pilot study published online April 6 in Bone.
Canadian researchers trained a convolutional neural network (CNN) model to detect abdominal aortic calcification in vertebral fracture assessment lateral spine images. High abdominal aortic calcification (AAC) scores are predictive of coronary artery calcium, cardiovascular outcomes, and death. The study results suggest DEXA machine-learning models could have a significant impact on cardiovascular disease risk management at the population level for older individuals.
"We found that the trained CNNs were able to detect abdominal aortic calcification score in the VFA [vertebral fracture assessment] images with a high level of agreement between the human-labeled and CNN-predicted scores," wrote a team led by Dr. William Leslie of the University of Manitoba, Winnipeg, Canada.
Screening for vertebral fractures on lateral spine imaging is valuable for osteoporosis management. At the same time, the images can be used to score abdominal aortic calcification. But this information is not routinely reported in clinical practice, perhaps reflecting that few readers of DEXA studies are trained to score AAC, or due to the perception that this task is time-consuming, the authors said.
In the study, the researchers looked at whether the CNN model could be directly trained to detect and quantify abdominal aortic calcification from lateral spine vertebral fracture assessment scans and compared their results with those scored by two study contributors trained by a diagnostic imaging specialist with over 15 years of clinical and research experience. They developed a test dataset from a random sample of 1,100 vertebral fracture assessment images from the Province of Manitoba Bone Density Program DXA database of individuals qualifying for vertebral fracture assessment as part of their osteoporosis assessment. For all scans, abdominal aortic calcification was manually scored using a validated 24-point semiquantitative scale and categorized as low (score < 2), moderate (score 2 to < 6), or high (score ≥ 6).
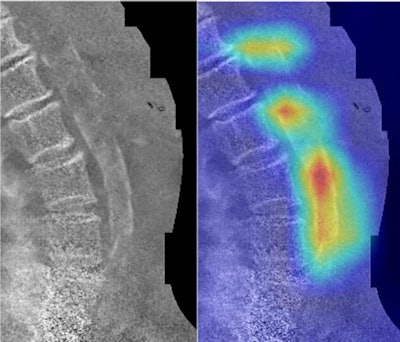
The researchers balanced training, validation, and test sets with respect to baseline characteristics and abdominal aortic calcification scores. The group found that weighted average sensitivity, specificity, and accuracy for predicting the correct abdominal aortic calcification category were 80.9%, 91.9%, and 88.1%, respectively.
Agreement between the human-labeled abdominal aortic calcification category and the CNN-predicted abdominal aortic calcification category | ||||
Performance measure | Low AAC < 2 (N = 83) | Moderate AAC 2 to < 6 (N = 55) | High AAC ≥ 6 (N = 82) | Weighted average (N = 220) |
Sensitivity | 84.3% | 74.5% | 81.7% | 80.9% |
Specificity | 92.7% | 83% | 97.1% | 91.9% |
F1 score | 85.9% | 66.1% | 87.6% | 81.6% |
Accuracy | 89.5% | 80.9% | 91.4% | 88.1% |
Negative predictive value | 90.7% | 90.7% | 89.9% | 90.4% |
Positive predictive value | 87.5% | 59.4% | 94.4% | 83.0% |
The CNN wasn't perfect: Out of 220 single-energy activation maps from the test data, 18 showed the CNN incorrectly localized on artifacts in the images (gas, ribs, noise, or medical pins located in the aorta region), three showed localization on abdominal aortic calcification next to the lumbar vertebra L5 or thoracic vertebra T12, and four showed the CNN did not detect the abdominal aortic calcification present. Sections of abdominal aortic calcification not fully detected were typically faint, segmented, or overlapping the spine.
But CNNs do show potential for detecting abdominal aortic calcification in vertebral fracture assessment images with high correlation between the human and predicted scores, Leslie and colleagues wrote.
"Our preliminary results suggest that CNNs are a promising method for automatically detecting and quantifying [abdominal aortic calcification]," they concluded. "Future work will need to confirm that these measures predict clinically relevant outcomes and generalize to other populations and DEXA manufacturers."