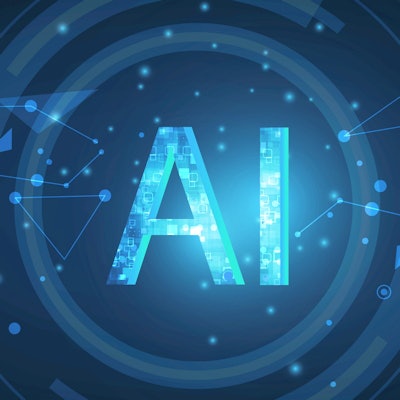
Lessons learned from decades of clinical use of mammography computer-aided detection (CAD) software are crucial in preparing for broad adoption of artificial intelligence (AI) tools in medical imaging, according to an article published on February 25 in JAMA Health Forum.
In a viewpoint article, Dr. Joann Elmore of the David Geffen School of Medicine at University of California, Los Angeles and Dr. Christoph Lee of the University of Washington School of Medicine in Seattle described the four key lessons that need to be applied now to avoid repeating the unfortunate history of mammography CAD software, which achieved widespread clinical use and reimbursement after first receiving U.S. Food and Drug Administration (FDA) clearance in 1998. However, the technology ultimately failed in large research studies to demonstrate improved radiologist accuracy.
"We stand at the precipice of widespread adoption of AI-directed tools in many areas of medicine beyond mammography, and the harms vs benefits hang in the balance for patients and physicians," the authors wrote. "We need to learn from our past embrace of emerging computer support tools and make conscientious changes to our approaches in reimbursement, regulatory review, malpractice mitigation, and surveillance after FDA clearance. Inaction now risks repeating past mistakes."
Complex user interactions
First off, it's important to remember that there are complex interactions between a computer algorithm's output and the interpreting physician, according to Elmore and Lee.
"While much research is being done in the development of the AI algorithms and tools, the extent to which physicians may be influenced by the many types and timings of computer cues when interpreting remains unknown," the authors wrote. "Automation bias, or the tendency of humans to defer to a presumably more accurate computer algorithm, likely affects physician judgment negatively if presented prior to a physician's independent assessment."
Before AI is widely adopted for medical imaging, it's important to evaluate the different user interfaces and better understand how and when AI outputs should be presented, according to the researchers.
"Ideally, we need prospective studies incorporating AI into routine clinical workflow," they wrote.
Reimbursement
Second, reimbursement of AI technologies needs to be incumbent on improved patient outcomes, not just better technical performance in artificial settings. Currently, FDA clearance can be obtained from small reader studies and a demonstration of noninferiority to existing technologies such as CAD, the authors noted.
"Newer AI technologies need to demonstrate disease detection that matters," they wrote. "For example, the use of AI in mammography should correspond with increased detection of invasive breast cancers with poor prognostic markers and decreased interval cancer rates. To demonstrate improved patient outcomes, AI technologies need to be evaluated in large population-based, real-world screening settings with longitudinal data collection and linkage to regional cancer registries."
Then, these results need to be confirmed to be consistent across diverse populations and settings to ensure health equity, according to the authors.
"Given the rapid pace of innovation and the many years often needed to adequately study important outcomes, we suggest coverage with evidence development, whereby payment is contingent on evidence generation and outcomes are reviewed on a periodic basis," they wrote.
Regulatory changes
In addition, revisions to the FDA clearance process are needed to encourage continued technological improvement, according to the researchers. Although the FDA's current review of AI tools in medical imaging is only provided for static software tools, the agency is drafting regulatory frameworks that will provide oversight over the total product lifecycle, including postmarket evaluation.
"One potential avenue for more vigorous, continuous evaluation of AI algorithms is to create prospectively collected imaging data sets that keep up with other temporal trends in medical imaging and are representative of target populations," they wrote.
Medicolegal risk
Finally, the impact of AI on medicolegal risk and responsibility in medical imaging interpretation also needs to be addressed.
"Without better guidance on individual party responsibilities for missed cancer, AI creates a new network of who, or what, is legally liable in complex and prolonged, multiparty malpractice lawsuits," the authors wrote. "Without national legislation addressing the medical and legal aspects of using AI, adoption will slow and we risk opportunities for predatory legal action."