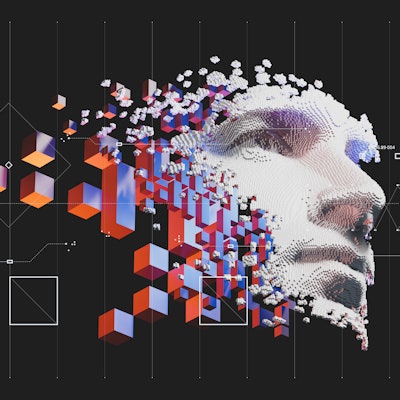
A preprint study that raised troublesome questions last year about potential bias in radiology artificial intelligence (AI) algorithms has now been formally published in the Lancet Digital Health with the same conclusions.
In July 2021, a team of 20 researchers from four countries led by Dr. Judy Gichoya from Emory University posted a preprint paper detailing how deep-learning models demonstrated consistently high performance for predicting a patient's racial identity across multiple imaging modalities and anatomical locations.
Significant changes were made from the original preprint after several rounds of peer review, including polishing of the message and the addition of new experiments, according to a spokesperson from MIT's Computer Science and Artificial Intelligence Laboratory.
However, the researchers still concluded that AI algorithms could readily learn to identify patients as Black, white, or Asian from medical imaging data alone -- even when radiologists could not -- and that this capability was generalizable across multiple imaging modalities and to external environments.
Despite repeated experiments and attempts to understand why the algorithms could achieve these results, the researchers were still unable to find an explanation.
"We strongly recommend that all developers, regulators, and users who are involved in medical image analysis consider the use of deep learning models with extreme caution as such information could be misused to perpetuate or even worsen the well documented racial disparities that exist in medical practice," the authors wrote.
Highly accurate performance
The researchers initially developed a deep-learning model using three large datasets: Emory CXR, MIMIC-CXR, and CheXpert. The algorithm was tested on both an unseen subset of the dataset used to train the model and a completely different dataset.
To assess whether this capability was limited to chest x-rays, they also developed other algorithms that analyzed nonchest x-ray images from other body locations, such as digital radiography (DR), lateral cervical spine radiographs, or chest CT exams. These algorithms utilized the Emory Chest CT, Emory Cervical Spine, Emory Mammography, National Lung Cancer Screening Trial, RSNA Pulmonary Embolism CT, and Digital Hand Atlas datasets for training and testing.
The algorithms achieved a high area under the curve (AUC) for identifying a patient's racial identity across multiple modalities:
- X-rays: 0.91-0.99
- Chest CT: 0.87-0.96
- Mammography: 0.81
In an attempt to understand the results of the models, the researchers explored a variety of potential factors, including differences in physical characteristics between racial groups, disease distribution, location-specific or tissue-specific differences, effects of societal bias and environmental stress, the ability of deep-learning systems to detect race when multiple demographic and patient factors were combined, and if specific image regions contributed to recognizing race.
Hard to explain
The study authors found that the algorithm's ability to detect a patient's race was not due to proxies such as body habitus, age, tissue density, or other potential imaging confounders such as a population's underlying disease distribution. Machine-learning models based on age, sex, gender, disease, and body habitus information performed much worse than the image-only models for classifying race. Notably, the researchers also found that the features learned by the algorithm appear to involve all regions of the image and frequency spectrum.
Even when variables such as differences in anatomy, bone density, and image resolution were considered, the models could still detect race from chest x-rays with a high level of accuracy.
Reason to pause?
Just because data from different groups are represented in training data doesn't guarantee that algorithms won't perpetuate or magnify existing disparities or inequities, according to co-author Dr. Leo Celi from MIT.
"Feeding the algorithms with more data with representation is not a panacea," he said in a statement. "This paper should make us pause and truly reconsider whether we are ready to bring AI to the bedside."
The researchers said that their findings indicate that future medical imaging AI efforts should emphasize model performance audits on the basis of racial identity, sex, and age. Furthermore, medical imaging datasets should include, when possible, the self-reported race of patients. This would enable further investigation and research into the racial identity information contained in images that are decipherable only by models, according to the authors.
The authors emphasized that the capability to predict self-reported race is, in and of itself, not the important issue.
"However, our finding that AI can accurately predict self-reported race, even from corrupted, cropped, and noised medical images, often when clinical experts cannot, creates an enormous risk for all model deployments in medical imaging," they wrote.