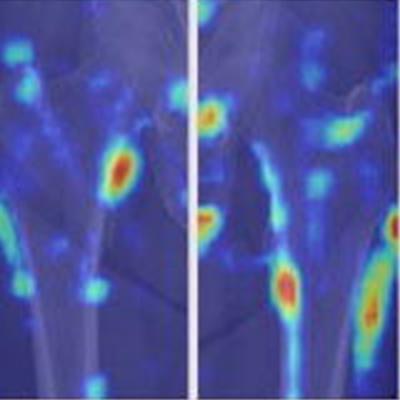
An artificial intelligence (AI) model based on features seen in hip x-rays could help clinicians diagnose osteoporosis. Researchers from South Korea explained their study in a paper published May 25 in Radiology: Artificial Intelligence.
With access to dual-energy x-ray absorptiometry (DEXA) for osteoporosis screening either limited or unavailable in many regions in the world, the authors suggest the tool could serve as a second reader to identify patients at risk for the disease.
"Our model can serve as a triage tool recommending DEXA in patients with highly suspected osteoporosis," said radiologist Dr. Hee-Dong Chae of Seoul National University Hospital (SNUH), in a news release from RSNA.
DEXA exams of bone mineral density are used globally as a reference for diagnosing osteoporosis. Yet its use for screening in regions with developing economies is limited due to the low availability of scanners and relatively high cost. Even in developed countries, many patients are left at risk for fracture without undergoing osteoporosis screening due to a lack of understanding among healthcare providers, according to the authors.
Comparatively, plain x-ray is "an almost ubiquitous imaging modality," the authors wrote.
Recent studies have shown the potential of deep learning as a promising tool for osteoporosis diagnosis, but most previous studies have been performed using either texture or deep-learning features alone, and studies using both features simultaneously are scarce, the authors wrote.
In this study, the researchers aimed to develop a "deep-radiomics" model that combined radiomics features, texture features, and clinical features of hip x-rays and validate it for diagnosing osteoporosis.
The researchers began with data on 54,687 consecutive adult patients with hip or pelvic anteroposterior x-rays acquired between January 2008 and April 2020 at SNUH. Among these records, they selected 8,686 patients with suspected osteoporosis who underwent DEXA exams.
After exclusions, they included 4,308 patients with 4,924 x-rays. Among these, 42% showed normal bone mineral density, 46% indicated osteopenia, and 13% showed osteoporosis.
From this dataset, the group extracted 20 deep-learning radiomics features, 32 bone region texture features, and three clinical features (age, sex, and weight) to train and test the model, named Model-DTC.
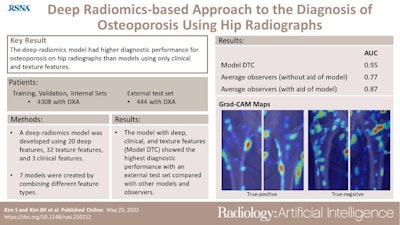
Next, six readers (two fourth-year radiology residents, two musculoskeletal radiology fellows, and two staff radiologists with 10 and seven years of experience) reviewed an external set of 444 hip x-rays over two sessions. In the first session, each observer reviewed the x-rays without the help of the deep-radiomics model, and in the second session, they evaluated the image while referring to the predictions of Model-DTC.
According to results, the stand-alone prediction by Model-DTC (AUC, 0.95) demonstrated higher performance diagnosing osteoporosis than predictions by observers with or without aid of the deep-radiomics model.
Moreover, with regard to the added value of Model-DTC as a second reader, the researchers found the diagnostic performance of all six observers significantly improved in the second session (average AUC, 0.87) over the first session (average AUC, 0.77).
In addition, the average sensitivity and specificity for the readers in the first session were 88% and 49%, which improved to 89% and 66% in the second session, the researchers found.
"With regard to the added value of the deep-radiomics model (Model-DTC) as a second reader, the diagnostic performance of all six observers was significantly improved in the second interpretation session," the authors wrote.
The group noted several limitations, namely that the study was retrospective and may have been affected by selection bias and that the model was not based on regression methods that continuously reflect bone mineral density. Thus, its use for treatment monitoring would be limited, they wrote.
Nonetheless, the bottom line is that the study showed a deep-radiomics model can be used to help diagnose osteoporosis on hip radiographs with high diagnostic performance, Chae and colleagues wrote.
Future studies are planned to evaluate the performance of deep-learning approaches for predicting fracture risks on x-rays in patients with osteoporosis, as well as validating the model in various ethnic populations other than Asians with a wide range of body weights, the authors concluded.