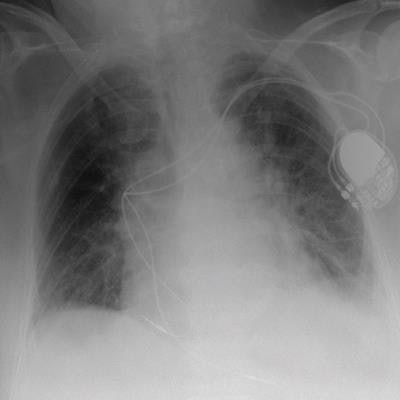
An artificial intelligence (AI) algorithm that provided readings on bedside chest x-rays helped nonradiologist physicians in the intensive care unit outperform expert radiologists, a study published December 6 in Radiology has found.
A group led by Firas Khader of the University Hospital Aachen in Germany developed a neural network-based model trained on structured, semiquantitative bedside chest x-rays. In a test comparing its use among expert radiologists nonradiologist physicians, the algorithm gave nonradiologists the edge, the researchers reported.
"There is a substantial need for computer-aided interpretation of chest radiographs, and neural networks are promising candidates to tackle this diagnostic problem," Khader and colleagues wrote.
Findings on chest radiographs can be ambiguous, and radiologists may not be available for immediate evaluation or consultation with physicians in the ICU, according to the authors. In approximately one-third of ICUs, nonradiologist physicians are solely responsible for the evaluation of chest radiographs.
For their study, Khader's team developed a neural network model based on an in-house data set that consisted of 193,566 portable bedside chest x-rays from 45,016 pediatric and adult patients acquired at their hospital between January 2009 to December 2020. The model was trained to identify cardiomegaly, pulmonary congestion, pleural effusion, pulmonary opacities, and atelectasis.
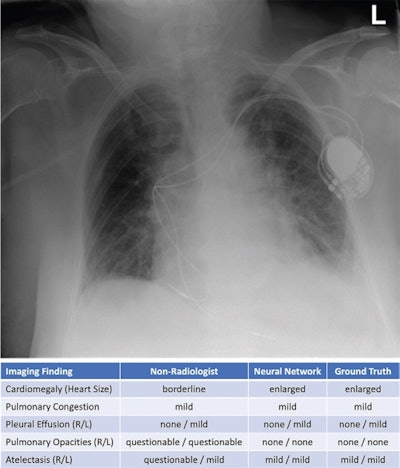
Next, a separate internal test set of 100 radiographs from 100 patients was assessed independently by a panel of six radiologists to establish ground truth for each exam. The team then used the kappa measure to compare these individual assessments, as well as those rendered by two ICU nonradiologist physicians using the neural network and by the neural network alone.
The neural network showed strong agreement with the majority vote of the radiologist panel (kappa, 0.86) -- a performance higher than the agreements of the individual radiologists with ground truth information (average kappa, 0.81).
In addition, with access to the results from the neural network when rating the radiographs, both of the ICU physicians improved their agreement with ground truth from 0.79 to 0.87 (p < 0.001), which was slightly higher than the agreement achieved by each unaided radiologist alone, the researchers reported.
In an accompanying editorial, Dr. Mark Wielputz of Heidelberg University Medical Center noted limitations of the study, among them that multiple human readers generated the ground truth by using structured reports and not by direct image annotation.
"The AI system presented may not perform much better than the average experienced radiologist in diagnostic precision or in detecting abnormalities," he wrote.
Despite this, the study shows that AI-based software can strongly support the interpretation of bedside chest radiographs for inexperienced readers, Wielputz concluded.