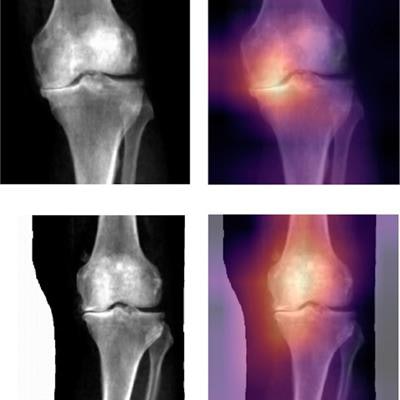
AI appears to be able to flag people with genetic traits for osteoarthritis based on their bone scans, according to researchers in Texas.
A group led by PhD students Brianna Flynn and Emily Javan at the University of Texas at Austin developed a deep-learning model to detect genetic traits associated with osteoarthritis on dual-energy x-ray absorptiometry (DEXA) knee scans from 29,257 individuals. The findings support the emerging concept of "image-based phenotyping," according to the researchers.
"For some diseases, such as knee osteoarthritis where the routine course of diagnosis involves an x-ray, image-based phenotyping offers an alternate and unbiased way to ascertain disease cases," the group wrote in an article published August 21 in npj Digital Medicine.
Genetic association studies that seek to link mutations with disease traits typically rely on biobanks that contain detailed genetic and health information on millions of participants. Culling patients from these databases usually involves identifying them based on ICD-10 billing codes with limited clinical information, the authors explained.
An alternate approach might be to directly perform association studies using medical images in these biobanks based on a consistent diagnosis protocol, they hypothesized.
To that end, the group first identified image-based phenotypes by matching osteoarthritis characteristics on DEXA images with osteoarthritis-associated genome sequence data of 42,284 individuals in the U.K. Biobank. The pairing of the data was based on joint space narrowing, subchondral sclerosis, and the presence of osteophytes, three hallmarks of the disease.
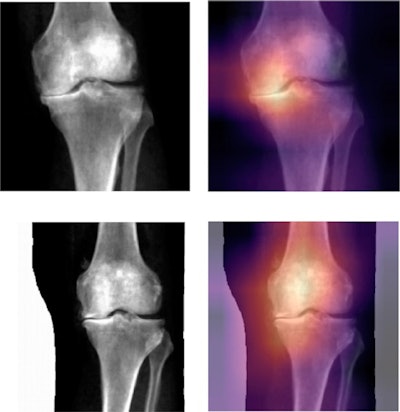
The researchers then trained the AI model to identify these image-based phenotypes on a separate dataset of 546 images that had been independently annotated by three board-certified orthopedic surgeons. Next, in a test dataset of 110 images, the deep-learning model identified the imaging phenotypes with a sensitivity of 82% compared with clinicians (77%) and a specificity of 95% compared with the clinicians (97%).
Finally, the researchers compared the AI model's performance to results from using the ICD-10 code M17 (knee osteoarthritis) to identify potential patients in a dataset of 28,725 cases from U.K. biobank.
"Using our model, we identified 1,931 (178%) more cases than currently diagnosed in the health record," the group wrote.
Ultimately, while computer vision approaches to extract and analyze DEXA scan-derived phenotypes are not themselves novel, this work is among the first to use the approach on a disease for which diagnosis is primarily radiographic, the authors noted.
"For diseases with radiographic diagnosis, our results demonstrate the potential for using deep learning to phenotype at biobank scale, improving power for both genetic and epidemiological association analysis," the researchers concluded.
The full article is available here.