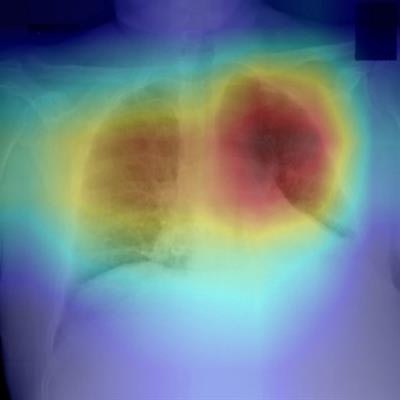
An artificial intelligence (AI) algorithm called CV19-Net boosted the performance of thoracic radiologists when it came to distinguishing COVID-19 pneumonia from other types of pneumonia on chest x-ray, according to a study published September 24 in Radiology.
The findings are good news as radiologists try to better understand COVID-19's appearance on x-ray, wrote a team led by Ran Zhang, PhD, of the University of Wisconsin in Madison.
Recent studies have found that the sensitivity of chest x-ray was poor for diagnosing COVID-19 cases, the group noted. It's possible that this poor diagnostic performance is because many radiologists are seeing pneumonia cases caused by COVID-19 for the very first time. Radiologists should read more cases to learn the telltale features of COVID-19-induced pneumonia, but machine learning can help in the meantime.
"Machine learning, particularly deep-learning methods, have unique advantages in quick and tireless learning to differentiate COVID-19 pneumonia from other types of pneumonia using chest x-ray images," the authors wrote
Zhang's group developed an AI algorithm to address the problem of poor diagnostic performance on diagnosing COVID-19 on x-ray. The team trained, validated, and tested a deep neural network called CV19-Net with chest x-rays taken from patients with and without COVID-19. Those patients with the disease had been confirmed for it between February and May of this year, while the group of chest x-rays from patients without COVID-19-induced pneumonia were taken between October and December 2019.
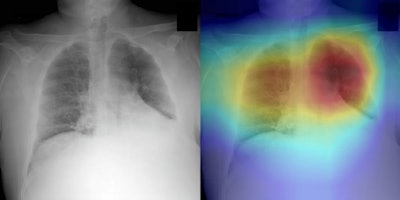
The researchers compared the sensitivity, specificity, and area under the receiver operating characteristic curve (AUC) of three experienced thoracic radiologist readers with those of the algorithm. Data from a total of 2,060 patients with COVID-19 pneumonia (5,806 chest x-rays) and 3,148 patients with non-COVID-19 pneumonia (5,300 chest x-rays) were used to train, validate, and test the algorithm; the team used 500 x-rays for the study test set (half of the cases were COVID-19 positive and half were negative).
The algorithm performed comparably or better in sensitivity to the radiologist readers; in particular, when the researchers used interpretation results of one particular image from the three readers, they found an averaged AUC of 0.85 compared with the algorithm's AUC of 0.94 on the study test set.
Performance of CV19-Net algorithm for COVID-19 pneumonia | ||||
Measure | Reader 1 | Reader 2 | Reader 3 | AI algorithm |
Sensitivity | 42% | 68% | 90% | 96% |
Specificity | 96% | 85% | 55% | 99% |
"The combination of chest radiography with the proposed CV19-Net deep learning algorithm has potential as an accurate method to improve the accuracy and timeliness of the radiological interpretation of COVID-19 pneumonia," the team concluded.