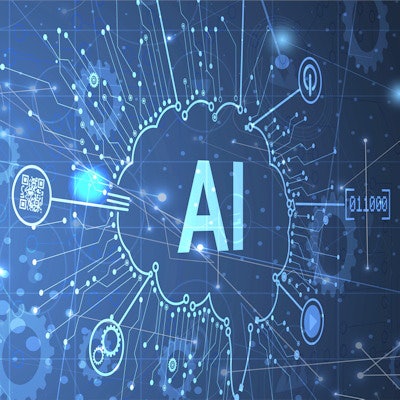
Radiologists can interpret advanced imaging studies more efficiently and identify suspicious findings faster with help from an artificial intelligence (AI) algorithm, according to a recent study.
Researchers from Switzerland developed a prototype natural language processing and image-matching algorithm to identify and present relevant prior examinations for the radiologist. In testing, they found that mean interpretation times for CT and MRI exams dropped by 40%.
The results suggest a way to streamline the radiology department's workflow.
"[Acceleration] of finding evaluation by automatizing reoccurring actions otherwise manually performed by a radiologist is feasible, as demonstrated with an algorithm assisting in finding comparison to relevant prior studies," wrote a team led by Dr. Thomas Weikert of University Hospital Basel in Switzerland in an article published May 18 in Academic Radiology. "This is especially helpful in incidentally identified findings that warrant a quick comparison to available priors, such as pulmonary nodules. The time-saving potential of applications of this kind seems high."
Accessing relevant priors
Comparing current imaging findings to prior exams is a key part of patient workup, but it's time-consuming, especially when radiologists are dealing with cross-sectional images, the team noted. This comparison is done manually by adapting the DICOM viewer display; finding relevant priors; navigating to the appropriate anatomic location being investigated; optimizing the display of the image by zooming in, panning, and adjusting brightness or contrast; and measuring the dimension of the suspicious finding. It can be pretty tricky, the team noted.
"The time-intensive nature of this ... assessment is not only suboptimal in the setting of increasing radiology workloads, but can also result in limited review of relevant prior examinations, putting interpretive accuracy and report quality at risk," the authors wrote. "For example, it is known that slow-growing pulmonary tumors are a meaningful clinical entity with only subtle changes over time that are easily overlooked in radiological routine if only the most recent prior examination is reviewed. Thus, optimizing identification and presentation of all relevant prior studies can enhance both efficiency of radiology workflows and report quality."
Deep learning would seem like an excellent way to streamline the process of comparing imaging findings to prior exams, according to Weikert and colleagues.
TimeLens
They conducted a study to assess the impact of a deep-learning tool called TimeLens -- a natural language processing and image-matching algorithm -- on what they call the radiology department's "finding assessment workflow." TimeLens is a research prototype, the group noted.
The study included 3,872 series of 246 radiology exams from 75 patients; of these, 189 were CT exams and 95 were MRI exams. The investigators used TimeLens to track the following findings: aortic aneurysm, intracranial aneurysm, kidney lesion, meningioma, and pulmonary nodules. Nine radiologists (four board-certified and five residents) underwent a training for using the algorithm and performed two reading sessions; their task was to measure the diameter of the finding of interest on two or more exams -- a most recent and at least one prior -- first without using the deep-learning tool and then with the algorithm after an interval of at least 21 days.
The authors also tracked readers' mouse movement patterns as part of evaluating interpretation time. They then evaluated TimeLens's effect in total, per finding type, per reader, per practice experience (resident compared with board-certified radiologist), and per modality.
The deep-learning tool reduced the average time needed to assess a finding by 40.1% (107 seconds compared with 65 seconds, p < 0.001). The biggest interpretation time reductions were for assessing pulmonary nodules (47% reduction). The group also discovered that using TimeLens reduced readers' mouse clicks for by 17.2%.
Effect of using TimeLens deep-learning algorithm for CT and MRI exam interpretation, including comparison with priors | |||
Metric | Without TimeLens | With TimeLens | Percent change |
Mean time per case (in seconds) | 107.4 | 64.3 | -40.1% |
Number of mouse clicks | 15,787 | 13.075 | -17.2% |
As comparing current exams to priors is a routine task for radiologists, "the time-saving potential of the method seems high," the group noted.
"[TimeLens] significantly reduced both the amount of user interactions with the radiology image viewer and the time needed to assess findings of interest on cross-sectional imaging with relevant prior exams," the team wrote.
The authors conceded that there are a few factors that could also have contributed to reduced interpretation times: The fact that the readers had seen same cases before and after using TimeLens and may have remembered the location of a finding (what the team called "case memory effect") and the fact that in the second interpretation round (with TimeLens), readers were used to the evaluation platform ("habituation effect").
Significant time savings
Weikert and colleagues also performed a basic analysis of how many hours of interpretation time TimeLens could save, writing that "on the basis of official NHS [National Health Service] statistics reporting 780,000 cross-sectional imaging examinations in August 2021, and assuming an average of three findings per examination warranting longitudinal comparison, this adds up to an estimated time-saving of 1,145 days (or 37 months) of radiologists reading time per month in the NHS system alone."
"Of course, those numbers have to be interpreted with highest caution and further assessments of true time savings in clinical routine are needed," they urged.