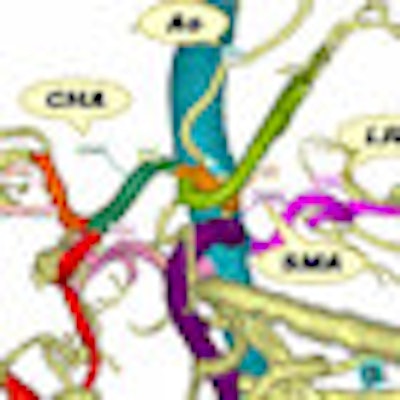
A new computer-aided detection (CAD) algorithm automatically labels abdominal arteries extracted from CT images. The work-in-progress system is more accurate and less dependent on error-prone features than previous CAD schemes, say researchers from Nagoya, Japan.
The study demonstrates that CAD software has potential to be used both preoperatively and intraoperatively to reduce errors, save time, and improve clinicians' understanding of complex anatomy, said Takayuki Kitasaka from Nagoya University at the June 2010 Computer Assisted Radiology and Surgery (CARS) meeting in Geneva.
"CAD systems should understand blood vessel structure," Kitasaka said. "In current CAD systems, the artery regions can be segmented automatically, but recognition of its structure ... is very complicated."
Understanding blood vessel anatomy is especially important in presurgical planning, where the knowledge is critical to operating in difficult environments such as laparoscopic surgery, which is limited to a small field-of-view.
However, blood vessel networks are complex and highly variable among individuals, Kitasaka said.
Surgeons need a "selective display of arteries of interest by color coding or annotation," he said. "To do this, the CAD system should recognize artery structure and anatomical names."
It's certainly been tried before: Shinoda and colleagues proposed anatomical name labeling for abdominal arteries based on position information of organs, Kitasaka said. However, the method required organ segmentation, and as a result, any segmentation failure resulted in labeling failure.
In another previous study, one of Kitasaka's co-authors, Kensaku Mori, "proposed an anatomic labeling method for bronchial branches that utilizes machine learning, and labels each branch from trachea to descendant branches sequentially," Kitasaka said. The drawback of this technique, however, was a predisposition toward continuous errors once labeling failed at a particular vessel branch. In any case, this method is not applicable to abdominal arteries due to far greater variations in branching patterns compared to the bronchus.
"Our purpose is to develop an anatomical labeling method that can find a globally suitable solution to avoid continuous errors and without organ position information, by analyzing blood vessel features," he said.
Learning features
First, the proposed method "learns" the blood vessel features. It then tests the nomenclature process by optimizing vessel candidate name combinations based on a series of features.
The labeling process targets 10 major arteries: the aorta (AO), celiac artery (CA), splenic artery (SA), common hepatic artery (CHA), proper hepatic artery (PHA), gastroduodenal artery (GDA), right gastroepiploic artery (RGEA), superior mesenteric artery (SMA), left renal artery (LRA), and right renal artery (RRA).
These vessels are divided into four main groups that share certain features useful in their identification -- for example, the aorta can be identified only by vessel thickness, Kitasaka said.
"Group A is aorta, group B includes these arteries starting from celiac artery [CA, SA, CHA, PHA, GDA, RGEA], group C is SMA, and group D is the renal arteries [LRA, RRA]," Kitasaka said. The CAD scheme characterizes each arterial branch by its "running direction" (orientation), length, and thickness, he said.
![]() |
Labeling focused on 10 arteries in the central abdomen: the aorta, celiac artery, splenic artery, common hepatic artery, proper hepatic artery, gastroduodenal artery, right gastroepiploic artery, superior mesenteric artery, left renal artery, and right renal artery. These were divided into four groups: the aorta, celiac artery group, superior mesenteric artery, and renal arteries. All images courtesy of Takayuki Kitasaka. |
In the learning phase of the CAD function, the arterial tree structure is extracted and skeletonized using a thinning algorithm, and a tree structure is built. The second step calculates the blood vessel features for each tree structure for learning, running direction (di) and length (li), which are calculated for each branch (bi), yielding vector x, Kitasaka said.
"Then we calculate the mean vector [Dj, Nj, Lj], covariance matrix, and standard deviations for each target name," he said. "The third step is learning the distribution of features," assuming a normal distribution. Preprocessing and feature calculation are performed for an input CT image using the same procedures as in the learning phase (yielding tree T and feature vector x).
Finally, anatomical labeling is performed by optimizing the candidate name combination for each branch. Potential sets of calculations that are both anatomically and topologically possible are then enumerated from the tree.
![]() |
Above, vessels were correctly identified; however, incorrect results were found in the case below. |
![]() |
The value of each candidate combination is calculated by an equation, then the candidate combination with the highest likelihood is chosen for display, Kitasaka explained.
The study team, which included researchers from Aichi Institute of Technology in Toyota and Aichi Cancer Center in Nagoya as well as Nagoya University, applied the proposed method to 89 cases of contrast-enhanced 3D abdominal CT images acquired in the arterial phase, 30 seconds after contrast injection. CT images were acquired at a slice thickness of 0.5 to 1 mm, with a reconstruction pitch of 0.4 to 0.5 mm and pixel size of 0.430 to 0.782 mm, Kitasaka said.
The results were evaluated using a leave-one-out scheme defined by the number of correctly labeled branches divided by the number of all branches with unique anatomical names, Kitasaka said. The average correct rate was 85.6%.
![]() |
CAD results in 89 patients showed an average correct rate of 85.6%. |
"The best combination corresponded to the correct one in most cases; however, there were some failure cases [where] an incorrect combination [of features] showed the highest likelihood," Kitasaka said. This means that a single branch having a very high likelihood of a certain name, while others are low, can become the branch with the highest likelihood.
"Additional features or improvement of the likelihood function is needed" and will be considered in future work, Kitasaka said.
By Eric Barnes
AuntMinnie.com staff writer
September 2, 2010
Related Reading
ASIR cuts dose by 27% in coronary CT angiography, August 20, 2010
Cardiac CAD safely rules out coronary artery disease, June 1, 2010
CAD takes on arteries with coronary stenosis detection, November 19, 2009
Automated segmentation could improve fetal heart echo, November 6, 2009
CARS report: Fuzzy theory yields sharper CTA images, June 27, 2008
Copyright © 2010 AuntMinnie.com