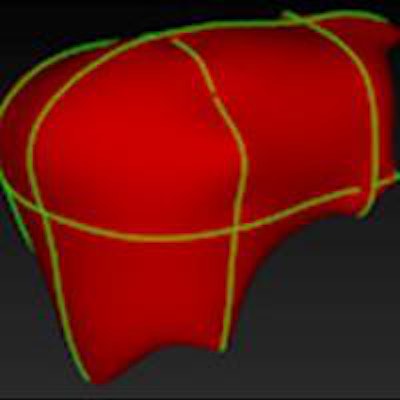
Liver segmentation, used to obtain liver volumes when planning for major interventions, can be automated without loss of accuracy compared to manual measurements, cutting procedure time by more than half, according to a new study in Academic Radiology.
The retrospective study of 41 patients who underwent liver CT scans for preoperative planning tested a semiautomated segmentation scheme. The researchers found that it produced liver volume measurements similar to those from a manual technique, while also demonstrating good reliability between different readers. In addition, the 30 minutes required for manual processing was reduced to just eight minutes with the algorithm (Acad Radiol, April 20, 2015).
"Liver segmentation is required prior to large hepatectomies, so whenever a surgeon anticipates resection of a large liver volume, they require liver volumetry," study co-author Dr. An Tang told AuntMinnie.com. "The process is time-consuming, so there's a need for automation of these steps. We developed a convenient segmentation method which is quite rapid to perform."
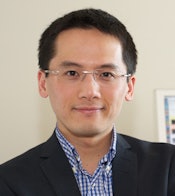
Tang is an associate professor of radiology at the University of Montreal.
Advantages of automation
The use of CT for presurgical imaging allows physicians to assess vascular anatomy and the quality of the liver parenchyma, as well as to determine total lobar volume. The potential advantages of automated segmentation include shorter processing times, greater agreement between readers, and repeatability, the authors wrote. And while many studies have proposed semiautomated or automated liver segmentation methods from CT datasets, few have been translated into clinical use.
Some of the previous studies of automated segmentation have been burdened by small sample sizes, poorly defined performance metrics, and datasets that do not reflect common clinical problems. Researchers in the liver segmentation community have proposed several methods to assess segmentation results objectively, including measuring agreement in volumetric overlap error, average symmetric surface distance, root mean square (RMS) symmetric surface distance, and maximum symmetric surface distance.
The algorithm developed by the Montreal group meets those challenges, according to the authors.
Patient testing
The researchers tested the algorithm on 41 subjects (22 men, 19 women; mean age, 55 years) who underwent hepatectomy between 2006 and 2009. Patients were included if they had primary or metastatic liver tumors and underwent major hepatectomy. The study describes the use of the algorithm with images generated by CT, but it can be used with MRI as well, the authors wrote.
In the current study, CT was performed using one of two MDCT scanners with standard abdominal imaging protocols. A 16-detector-row scanner (LightSpeed 16, GE Healthcare) was used for 25 patients (120-140 kV, 75-440 mAs, 1.25-mm collimation), and a 64-detector-row machine (Brilliance 64, Philips Healthcare) was used for the other 16 patients (120 kV, 151-499 mAs, 0.625-mm collimation).
The group segmented both total and subsegmental liver volumes from the venous-phase contrast-enhanced CT images. Two image analysts independently performed semiautomated segmentations, while two others performed manual segmentations. Intraclass correlation coefficients (ICCs) and Bland-Altman analysis were used to measure repeatability and agreement of both methods.
Filling gaps, smoothing bumps
The semiautomated liver segmentation software initiates segmentation by roughly delineating the liver contour on four to six CT slices. The software then uses variable interpolation to generate an initial 3D shape, which is deformed manually and then automatically using minimal-path surface segmentation, the authors wrote.
Interpolation refers to filling in the gaps between missing data to complete the 3D image, Tang explained. Minimal-path surface segmentation means "minimizing the surface of the liver to avoid the lumps and bumps, so you have a smooth curvature of the liver."
After the 3D shape is deformed, vessels are excluded using a locally seeded region-growing technique. Finally, the software calculates liver volume for each slice, the authors wrote.
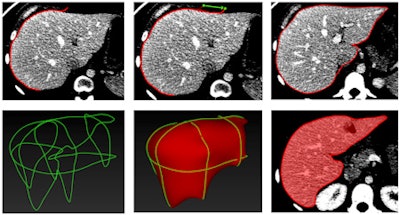
Quadruple the speed
The semiautomated method turned in an estimate of whole-liver volume that was nearly identical to the volume calculated manually: 1,688 ± 497 mL for the algorithm, compared with 1,689 ± 478 mL for the manual method, and the difference was not statistically significant (p = 0.92). The only significant difference in the comparison of segmental volumetry was for the caudate lobe (p = 0.01).
Interreader agreement was slightly higher for the manual method, but it was still good for the semiautomated technique. Bland-Altman analysis showed an intraclass correlation coefficient of 0.999 (p < 0.001) for manual segmentation and 0.991 (p < 0.001) for semiautomated segmentation.
Finally, the automated technique was more than twice as fast per case than manual segmentation, at 8.0 ± 1.2 minutes versus 34.3 ± 16.7 minutes (p < 0.001).
Manual vs. semiautomated liver volume measurement | |||
Method | Volume estimate | Interreader agreement | Speed |
Manual measurement | 1,689 ± 478 mL | 0.999 | 34.3 ± 16.7 minutes |
Semiautomated algorithm | 1,688 ± 497 mL | 0.991 | 8.0 ± 1.2 minutes |
"The [manual] segmentation time of half an hour is probably longer than it would take in real life -- just because for a study on diagnostic accuracy you have to be very meticulous doing the liver contour perfectly, where in real life I guess one would take 15 to 20 minutes," Tang said.
A semiautomated segmentation method can shorten interaction time while maintaining high repeatability and agreement with manual segmentation, the authors concluded. While the technique may not do as well in a future study of patients with cirrhosis, for example, the increased speed it offers is paramount, they added.
"Manual segmentation is often considered to be too time-consuming for clinical purposes," they wrote. "Thus, a fourfold decrease in mean interaction time is clinically relevant."
The fact that the method can be used with MR images as well as CT is another advantage, potentially revealing biomarkers of chronic diseases that can be measured with MRI, according to Tang.
"We wanted a tool that could work with both modalities, hence the need to develop our own in-house solution," Tang said. "It will make liver segmentation faster and easier to implement on a wider scale. We currently reserve segmentation for more complex cases, but [the algorithm] will speed up the process and could democratize our choice of cases."
Future plans include adapting the technique to MR-based liver volumetry, as well as automating segmental volumetry based on vascular anatomy, the authors wrote.