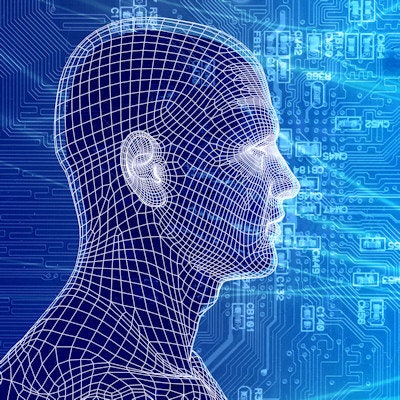
A machine-learning algorithm shows potential for facilitating the holy grail of real-time, patient-specific radiation dose estimates from CT scans, according to research presented at the Society for Imaging Informatics in Medicine (SIIM) annual meeting earlier this month in Pittsburgh.
In a proof-of-concept study, a team from Massachusetts General Hospital (MGH) implemented a machine-learning algorithm that could identify organs on CT datasets with approximately 99% accuracy -- the first step in creating real-time radiation dose estimates that are customized for individual patients.
"By using our [artificial intelligence (AI)]-based organ recognition combined with dose estimation tool we can provide patient-specific organ dose," said presenter and senior author Synho Do, PhD.
Estimating radiation dose
Radiation dose estimation is a big issue in radiology today. While reports of radiation dose to patient organs are an important part of radiation safety, current techniques for estimating organ dose rely on Monte Carlo simulations based on phantoms or use measurements on phantoms with a dosimeter, according to the researchers. The position, orientation, and shape of organs vary by patient, however, so it can be difficult to map a given CT slice to the slab number of a phantom model to accurately calculate organ dose.
The MGH team sought to apply machine learning to the problem in the hope of achieving real-time, patient-specific organ dose estimates. The researchers trained a 22-layer deep convolutional neural network using CT exams from 70 male patients with 20,424 image slices and 70 female patients with 19,335 image slices.
The first six layers of the neural network provide body part recognition, while the remaining 16 focus on organ detection. Using the axial views of the CT images, six different body parts are initially categorized and annotated: the brain, neck, shoulder, abdomen, chest, and pelvis. Next, the convolutional neural network classifies and labels the organs.
After an organ is classified by the algorithm, its shape and size are automatically mapped to the slab number of the mathematical phantom to determine the scan range for the ImPACT CT dose calculator, Do said. Fine-tuning is also performed to account for factors such as patient weight and organ volume.
"It's not perfect right now, but it's a proof of concept," Do said.
Accurate body-part classification
The researchers then tested the system on CT studies from 30 male patients with 8,564 image slices and 30 female patients with 8,017 image slices. The algorithm demonstrated high accuracy for classifying body parts:
- Male patients: 98.9%
- Female patients: 99.1%
"Compared to text-based body part retrieval in the DICOM header, image-based organ recognition is accurate and fast with a machine-learning algorithm," Do said. "This technique can be used for patient-specific organ dose estimation since the location and sizes of organs for each patient can be calculated independently."