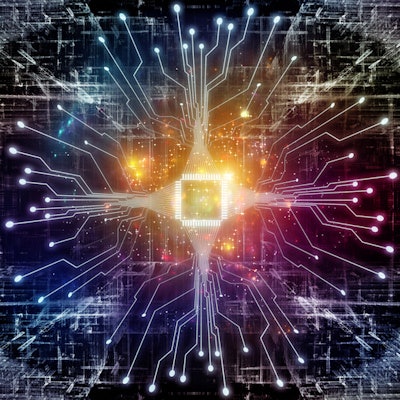
A group from Alabama has developed a deep-learning artificial intelligence (AI) algorithm capable of detecting lung nodules on CT scans while maintaining a low false-positive rate, according to an article published online August 22 in the Journal of the American Medical Informatics Association.
The researchers, led by Ashish Gupta, PhD, from Auburn University, trained a 3D deep-learning convolutional neural network (CNN) to perform two consecutive tasks: identifying potential pulmonary nodules and then reducing the number of false positives among the nodules detected.
Recent studies have shown that deep neural networks are capable of detecting nodules on CT with an accuracy that matches, and even occasionally surpasses, that of human readers, the authors noted. If integrated into clinical practice, such algorithms could aid radiologists in tasks such as CT lung cancer screening by reducing reading times or acting as a second reader. However, many AI algorithms continue to have trouble recognizing low-density nodules, as well as those attached to blood vessels or the pleural wall.
To address these and other deficiencies, Gupta and colleagues adapted a deep-learning CNN known as U-Net to perform nodule detection. U-Net was capable of learning representations of internal nodule features -- such as varying shape and size -- directly from imaging data and then using this information to recognize increasingly complex features.
They tested the 3D deep neural network on 888 CT scans from the U.S. National Cancer Institute's Lung Image Database Consortium (LIDC) and Image Database Resource Initiative (IDRI).
For the first task, the 3D deep neural network detected lung nodules on CT with a sensitivity of 94.8%, including 30.4 false positives per scan. Subsequently, the deep-learning CNN examined the same scans and was able to lower the false-positive rate all the way down to 1.8 per scan.
Performance of a 3D deep neural network for lung nodule detection on CT | |
3D deep neural network | |
Sensitivity | 89.29% |
No. of false positives per scan | 1.789 |
Area under the curve | 0.9324 |
Other researchers using computer-aided detection (CAD) of pulmonary nodules have reported a wide range in sensitivity, from 76% to 95.45%, and in false positives, from 1.91 to 15.28 per scan, the authors noted. Though several other models achieved slightly higher sensitivity than the present 3D deep neural network, those particular models also had higher false-positive rates.
"We present a novel, fully automated system for the detection of pulmonary nodules that was extensively validated using each scan in the dataset for both training and test. ... As a whole, the system outperforms all previous deep-learning systems evaluated on test data and is among the best existing pulmonary nodule CAD systems," they wrote.
Moving forward, Gupta and colleagues hope to validate the 3D deep neural network on an external dataset such as the CT exams from the National Lung Screening Trial. They also plan to use the deep-learning technology to quantify the malignancy of lung nodules and possibly even provide diagnostic predictions.