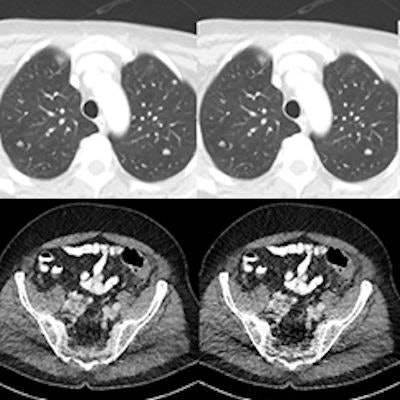
An artificial intelligence (AI) algorithm can transform low-dose CT (LDCT) scans into high-quality exams that radiologists may even prefer over LDCT studies produced via commercial iterative reconstruction techniques, according to research published online June 10 in Nature Machine Intelligence.
A team of researchers from Rensselaer Polytechnic Institute (RPI) in Troy, NY, and Massachusetts General Hospital (MGH) in Boston developed a deep-learning model called a modularized adaptive processing neural network (MAP-NN), which progressively reduces noise on LDCT images with guidance from the radiologist until the optimal level of image quality is achieved. Testing on images from three different vendors, three radiologists found the algorithm produced images that were either better or comparable to images processed with iterative reconstruction. The deep-learning method also processed images much faster.
"The deep-learning approach can thus already effectively compete with iterative reconstruction solutions and potentially replace the iterative reconstruction approach," wrote the group led by Hongming Shan of RPI.
Although commercial iterative reconstruction methods have helped to lower radiation dose in CT studies, they can also alter image appearance and add artifacts. To see if deep learning could yield improvements, the researchers tested the MAP-NN model on 60 low-dose CT scans performed at MGH.
The 60 scans, including 30 routine abdominal studies and 30 routine chest exams, were acquired on scanners from three different vendors: GE Healthcare, Philips Healthcare, and Siemens Healthineers. For each of the LDCT scans, the sinogram data were reconstructed separately using each vendors' specific commercial iterative reconstruction algorithm, as well as filtered back projection (FBP) methods.
The researchers then applied the deep-learning model to the FBP-reconstructed studies in order to produce three denoised images for all cases. Next, three radiologists independently evaluated and scored the best deep learning-generated images and the best iterative reconstruction studies for two features: noise suppression and structural fidelity.
The radiologists preferred the best deep-learning reconstruction in two of the three vendors for the abdominal imaging cases. For the third vendor, the two image types were deemed statistically comparable. In chest exams, the deep-learning images and the iterative reconstruction images were judged to be statistically comparable for all three vendors.
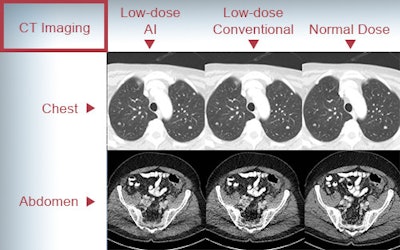
Delving further into the data, the researchers found that the deep-learning method earned significantly higher mean scores for noise suppression and structural fidelity than the iterative reconstruction methods.
The researchers noted that their deep-learning method was applied to CT images without access to the raw data. More powerful methods will require access to the sinogram data, however.
"In collaboration with a vendor, our algorithm could be specifically trained with their data and achieve an even better performance than what we have described here using our agnostic algorithm," they wrote. "With the availability of raw data, CT denoising can be performed from the sinogram domain to the image space, utilizing all the information for the best denoising results. Clearly, it is now time for CT vendors to open their data format, perform machine learning, and develop the next generation of CT image reconstruction algorithms in the deep-learning framework."