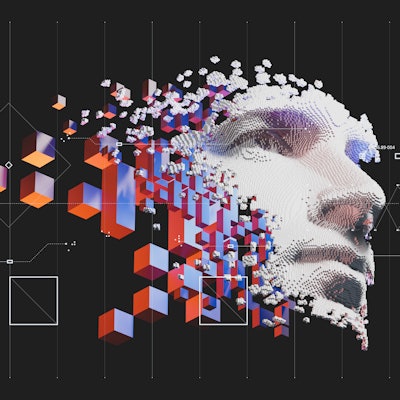
The use of deep learning to enhance image reconstruction has been considered to be a key application for artificial intelligence (AI) in radiology. A multinational team of researchers is warning, however, that the technology is prone to instability issues that could potentially even lead to a wrong diagnosis.
After testing six different AI-based medical image reconstruction methods, the researchers led by Anders Hansen, PhD, of the University of Cambridge in the U.K. and Ben Adcock, PhD, of Simon Fraser University in Burnaby, found widespread problems, including susceptibility to errors caused by patient movement, as well as blurring or removing of details when handling small structural changes. They shared their results in an article published online May 8 in the Proceedings of the National Academy of Sciences.
"There's been a lot of enthusiasm about AI in medical imaging, and it may well have the potential to revolutionize modern medicine: However, there are potential pitfalls that must not be ignored," Hansen said in a statement. "We've found that AI techniques are highly unstable in medical imaging, so that small changes in the input may result in big changes in the output."
The researchers developed a test to assess the stability of the methods in three areas: tiny worst-case perturbations, or small movements; small structural image changes such as a brain image with or without a tumor; and changing the number of images in the sampling device (i.e, MR or CT scanner). They chose six different deep-learning neural networks based on their strong performance, wide ranges in architectures, and difference in training data.
The networks included the following:
- Automated transform by manifold approximation (AUTOMAP): a neural network for low-resolution, single-coil MRI with 60% subsampling
- DAGAN: a conditional generative adversarial networks-based model for medium-resolution, single-coil MRI with 20% subsampling
- Deep MRI: a network for medium-resolution, single-coil MRI with 33% subsampling
- ELL 50: a network for CT or any Radon transform-based inverse problem. It samples 50 lines in the sinogram.
- Med 50: a network with the same architecture as ELL 50, but it was trained with medical images
- MRI VN: a variational network for medium-to-high resolution parallel MRI with 15 coil elements and 15% subsampling
The researchers found that certain tiny patient movements produced a myriad of artifacts in the final images for the algorithms. With the exception of Deep MRI, the researchers also demonstrated that image details were blurred or even completely removed when the algorithms were tested on their ability to consider small structural changes in images. What's more, a higher subsampling ratio led to deterioration in image reconstruction quality for ELL 50, Med 50, and DAGAN.
The most worrying errors were ones that radiologists might interpret as medical issues, as opposed to those that could be easily dismissed as a technical error, according to the researchers.
"We've found that the tiniest corruption, such as may be caused by a patient moving, can give a very different result if you're using AI and deep learning to reconstruct medical images -- meaning that these algorithms lack the stability they need," Hansen said.
The researchers said they are now focused on mathematically calculating the fundamental limits to what can be achieved with AI-based image reconstruction.