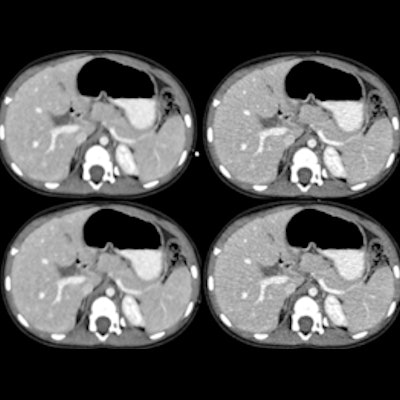
Deep learning-based image reconstruction algorithms can yield higher pediatric CT image quality and lower radiation dose than other conventional reconstruction techniques such as statistical-based iterative reconstruction (SBIR), according to research published online November 17 in Radiology.
After comparing a deep learning-based image reconstruction algorithm with filtered back projection (FBP), SBIR, and model-based iterative reconstruction (MBIR), a team of researchers led by Dr. Samuel Brady, PhD, of Cincinnati Children's Hospital Medical Center found that the deep-learning algorithm yielded greater ability to detect objects.
What's more, the deep-learning algorithm also produced higher image-quality ratings by radiologists and has the potential for lower radiation dose.
"The use of deep-learning reconstruction in the CT image reconstruction process is an innovative application of artificial intelligence and our results suggest that it has the potential to improve image quality, object detection accuracy, and radiologist confidence and to potentially reduce patient radiation dose," the authors wrote.
Although deep learning-based reconstruction algorithms have been developed to remove image noise, the impact of these techniques on image quality and radiation dose reduction hasn't been fully investigated yet, according to the researchers. As a result, they sought to compare the use of various reconstruction methods on 19 patients who had received clinical pediatric CT exams performed at their institution between February 2018 and December 2018.
First, the researchers sent the raw data from these contrast-enhanced pediatric chest-abdomen-pelvis exams to Canon Medical Systems for offline image reconstruction. The raw data were reconstructed transaxially at 0.5- and 3-mm image thicknesses using Canon's FBP, Adaptive Iterative Dose Reduction 3D statistical-based iterative reconstruction (SBIR), FIRST Body Standard model-based iterative reconstruction (MBIR), and Advanced Intelligent Clear-IQ Engine (deep learning-based reconstruction) algorithms.
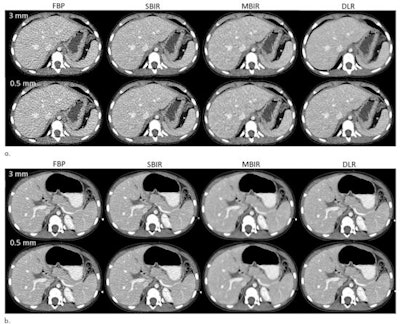
After a mathematical observer model was used to perform an objective comparison of the images produced by the various reconstruction methods, three fellowship-trained pediatric radiologists then provided a subjective comparison of the images. These observers assessed anatomic structures with low object-to-background signal and contrast to noise in the azygos vein, right hepatic vein, common bile duct, and superior mesenteric artery, providing ratings ranging from 1 (worst) to 10 (best) for edge definition, quantum noise level, and object conspicuity.
Performance of image reconstruction algorithms | ||||
FBP | SBIR | MBIR | Deep learning-based reconstruction | |
Object detectability | 10.9 | 13.9 | 14.8 | 16.5 |
Mean radiologist image quality ratings | 4.6 ± 1 | 6.2 ± 1 | 6.2 ± 1 | 7 ± 1 |
The researchers noted that deep learning-based reconstruction was able to reduce image noise without the noise texture effects experienced with MBIR. After performing two-way variance analysis, they also concluded that the difference in image quality ratings varied on the basis of reconstruction type (p < 0.001).
In other findings, the researchers found that the radiologists also consistently preferred the deep-learning images. The deep learning-based algorithm also yielded a 51% lower dose than SBIR, according to the authors.
"The [deep learning-based reconstruction] algorithm improved image quality and dose reduction without sacrificing noise texture and spatial resolution," the authors wrote.